Age-Based Developmental Biomarkers in Eye Movements: A Retrospective Analysis Using Machine Learning
- PMID: 39061426
- PMCID: PMC11274786
- DOI: 10.3390/brainsci14070686
Age-Based Developmental Biomarkers in Eye Movements: A Retrospective Analysis Using Machine Learning
Abstract
This study aimed to identify when and how eye movements change across the human lifespan to benchmark developmental biomarkers. The sample size comprised 45,696 participants, ranging in age from 6 to 80 years old (M = 30.39; SD = 17.46). Participants completed six eye movement tests: Circular Smooth Pursuit, Horizontal Smooth Pursuit, Vertical Smooth Pursuit, Horizontal Saccades, Vertical Saccades, and Fixation Stability. These tests examined all four major eye movements (fixations, saccades, pursuits, and vergence) using 89 eye-tracking algorithms. A semi-supervised, self-training, machine learning classifier was used to group the data into age ranges. This classifier resulted in 12 age groups: 6-7, 8-11, 12-14, 15-25, 26-31, 32-38, 39-45, 46-53, 54-60, 61-68, 69-76, and 77-80 years. To provide a descriptive indication of the strength of the self-training classifier, a series of multiple analyses of variance (MANOVA) were conducted on the multivariate effect of the age groups by test set. Each MANOVA revealed a significant multivariate effect on age groups (p < 0.001). Developmental changes in eye movements across age categories were identified. Specifically, similarities were observed between very young and elderly individuals. Middle-aged individuals (30s) generally showed the best eye movement metrics. Clinicians and researchers may use the findings from this study to inform decision-making on patients' health and wellness and guide effective research methodologies.
Keywords: eye movements; eye tracking; lifespan development; machine learning.
Conflict of interest statement
Greenstein, Singh, Murray, and Carrick have no conflicts of interest to declare. Hunfalvay and Bolte are full-time employees of RightEye, LLC.
Figures
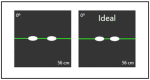
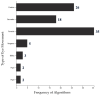
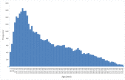
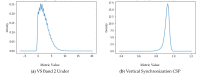
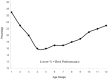
Similar articles
-
Smooth Pursuit Eye Movements as a Biomarker for Mild Concussion within 7-Days of Injury.Brain Inj. 2021 Dec 6;35(14):1682-1689. doi: 10.1080/02699052.2021.2012825. Epub 2021 Dec 11. Brain Inj. 2021. PMID: 34894915
-
[Eye tracking disorders in schizophrenic patients and their parents].Encephale. 2001 Nov-Dec;27(6):551-8. Encephale. 2001. PMID: 11865562 French.
-
A geometric method for computing ocular kinematics and classifying gaze events using monocular remote eye tracking in a robotic environment.J Neuroeng Rehabil. 2016 Jan 26;13:10. doi: 10.1186/s12984-015-0107-4. J Neuroeng Rehabil. 2016. PMID: 26812907 Free PMC article.
-
The Measurement of Eye Movements in Mild Traumatic Brain Injury: A Structured Review of an Emerging Area.Front Sports Act Living. 2020 Jan 28;2:5. doi: 10.3389/fspor.2020.00005. eCollection 2020. Front Sports Act Living. 2020. PMID: 33345000 Free PMC article. Review.
-
Review and Evaluation of Eye Movement Event Detection Algorithms.Sensors (Basel). 2022 Nov 15;22(22):8810. doi: 10.3390/s22228810. Sensors (Basel). 2022. PMID: 36433407 Free PMC article. Review.
References
-
- Leigh J.R., Zee D.S. The Neurology of Eye Movements. 5th ed. Oxford University Press; Oxford, UK: 2012.
-
- Poole A., Ball L.J. Eye tracking in human-computer interaction and usability research: Current status and future prospects. In: Ghaoui C., editor. Encyclopedia of Human-Computer Interaction. Idea Group Publishing; Hershey, PA, USA: 2005.
-
- Tenenbaum G. Expert athletes: An integrated approach to decision making. In: Starkes J.L., Ericsson K.A., editors. Expert Performance in Sports: Advances in Research on Sport Expertise. Human Kinetics; Champaign, IL, USA: 2003.
-
- Campbell D. Normative Data. In: Volkmar F.R., editor. Encyclopedia of Autism Spectrum Disorders. Springer; New York, NY, USA: 2013. - DOI
Grants and funding
LinkOut - more resources
Full Text Sources