Kernel density estimation of allele frequency including undetected alleles
- PMID: 38666077
- PMCID: PMC11044881
- DOI: 10.7717/peerj.17248
Kernel density estimation of allele frequency including undetected alleles
Abstract
Whereas undetected species contribute to estimation of species diversity, undetected alleles have not been used to estimated genetic diversity. Although random sampling guarantees unbiased estimation of allele frequency and genetic diversity measures, using undetected alleles may provide biased but more precise estimators useful for conservation. We newly devised kernel density estimation (KDE) for allele frequency including undetected alleles and tested it in estimation of allele frequency and nucleotide diversity using population generated by coalescent simulation as well as well as real population data. Contrary to expectations, nucleotide diversity estimated by KDE had worse bias and accuracy. Allele frequency estimated by KDE was also worse except when the sample size was small. These might be due to finity of population and/or the curse of dimensionality. In conclusion, KDE of allele frequency does not contribute to genetic diversity estimation.
Keywords: Allele frequency; Genetic diversity; Kernel density estimation.
© 2024 Aoki and Fukasawa.
Conflict of interest statement
The authors declare that they have no competing interests.
Figures
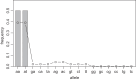
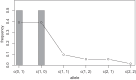
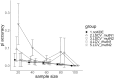
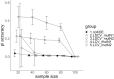
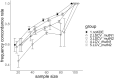
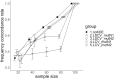
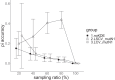
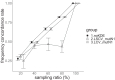
Similar articles
-
Robustness of genetic diversity measures under spatial sampling and a new frequency-independent measure.PeerJ. 2023 Sep 18;11:e16027. doi: 10.7717/peerj.16027. eCollection 2023. PeerJ. 2023. PMID: 37744217 Free PMC article.
-
Microsatellite null alleles and estimation of population differentiation.Mol Biol Evol. 2007 Mar;24(3):621-31. doi: 10.1093/molbev/msl191. Epub 2006 Dec 5. Mol Biol Evol. 2007. PMID: 17150975
-
Estimating pairwise relatedness in a small sample of individuals.Heredity (Edinb). 2017 Nov;119(5):302-313. doi: 10.1038/hdy.2017.52. Epub 2017 Aug 30. Heredity (Edinb). 2017. PMID: 28853716 Free PMC article.
-
On selecting markers for association studies: patterns of linkage disequilibrium between two and three diallelic loci.Genet Epidemiol. 2003 Jan;24(1):57-67. doi: 10.1002/gepi.10217. Genet Epidemiol. 2003. PMID: 12508256 Review.
-
Characterizing allelic association in the genome era.Genet Res (Camb). 2010 Dec;92(5-6):461-70. doi: 10.1017/S0016672310000637. Genet Res (Camb). 2010. PMID: 21429275 Free PMC article. Review.
References
-
- Blum MGB, Nunes MA, Prangle D, Sission SA. A comparative review of dimension reduction methods in approximate Bayesian computation. Statistical Science. 2013;28(2):189–208. doi: 10.1214/12-STS406. - DOI
-
- Heidenreich N-B, Schindler A, Sperlich S. Bandwidth selection for kernel density estimation: a review of fully automatic selectors. Advances in Statistical Analysis. 2013;97(4):403–433. doi: 10.1007/s10182-013-0216-y. - DOI
Publication types
MeSH terms
Grants and funding
LinkOut - more resources
Full Text Sources