FHIR-PYrate: a data science friendly Python package to query FHIR servers
- PMID: 37415138
- PMCID: PMC10326955
- DOI: 10.1186/s12913-023-09498-1
FHIR-PYrate: a data science friendly Python package to query FHIR servers
Abstract
Background: We present FHIR-PYrate, a Python package to handle the full clinical data collection and extraction process. The software is to be plugged into a modern hospital domain, where electronic patient records are used to handle the entire patient's history. Most research institutes follow the same procedures to build study cohorts, but mainly in a non-standardized and repetitive way. As a result, researchers spend time writing boilerplate code, which could be used for more challenging tasks.
Methods: The package can improve and simplify existing processes in the clinical research environment. It collects all needed functionalities into a straightforward interface that can be used to query a FHIR server, download imaging studies and filter clinical documents. The full capacity of the search mechanism of the FHIR REST API is available to the user, leading to a uniform querying process for all resources, thus simplifying the customization of each use case. Additionally, valuable features like parallelization and filtering are included to make it more performant.
Results: As an exemplary practical application, the package can be used to analyze the prognostic significance of routine CT imaging and clinical data in breast cancer with tumor metastases in the lungs. In this example, the initial patient cohort is first collected using ICD-10 codes. For these patients, the survival information is also gathered. Some additional clinical data is retrieved, and CT scans of the thorax are downloaded. Finally, the survival analysis can be computed using a deep learning model with the CT scans, the TNM staging and positivity of relevant markers as input. This process may vary depending on the FHIR server and available clinical data, and can be customized to cover even more use cases.
Conclusions: FHIR-PYrate opens up the possibility to quickly and easily retrieve FHIR data, download image data, and search medical documents for keywords within a Python package. With the demonstrated functionality, FHIR-PYrate opens an easy way to assemble research collectives automatically.
Keywords: Dataframe; Dicom; Electronic patient record; FHIR; Information extraction; Python.
© 2023. The Author(s).
Conflict of interest statement
The authors declare that they have no competing interests.
Figures
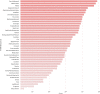
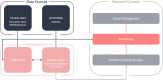
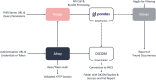
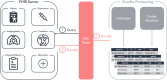
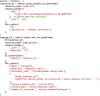
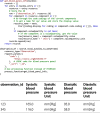
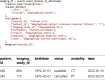
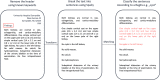
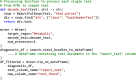
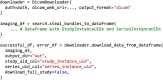
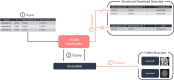
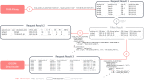
Similar articles
-
A Fast Healthcare Interoperability Resources (FHIR) layer implemented over i2b2.BMC Med Inform Decis Mak. 2017 Aug 14;17(1):120. doi: 10.1186/s12911-017-0513-6. BMC Med Inform Decis Mak. 2017. PMID: 28806953 Free PMC article.
-
Learning HL7 FHIR Using the HAPI FHIR Server and Its Use in Medical Imaging with the SIIM Dataset.J Digit Imaging. 2018 Jun;31(3):334-340. doi: 10.1007/s10278-018-0090-y. J Digit Imaging. 2018. PMID: 29725959 Free PMC article. Review.
-
"fhircrackr": An R Package Unlocking Fast Healthcare Interoperability Resources for Statistical Analysis.Appl Clin Inform. 2023 Jan;14(1):54-64. doi: 10.1055/s-0042-1760436. Epub 2023 Jan 25. Appl Clin Inform. 2023. PMID: 36696915 Free PMC article.
-
Patient-Centered Radiology with FHIR: an Introduction to the Use of FHIR to Offer Radiology a Clinically Integrated Platform.J Digit Imaging. 2018 Jun;31(3):327-333. doi: 10.1007/s10278-018-0087-6. J Digit Imaging. 2018. PMID: 29725963 Free PMC article. Review.
-
Opening the Duke electronic health record to apps: Implementing SMART on FHIR.Int J Med Inform. 2017 Mar;99:1-10. doi: 10.1016/j.ijmedinf.2016.12.005. Epub 2016 Dec 12. Int J Med Inform. 2017. PMID: 28118917
Cited by
-
Use of Real-World FHIR Data Combined with Context-Sensitive Decision Modeling to Guide Sentinel Biopsy in Melanoma.J Clin Med. 2024 Jun 6;13(11):3353. doi: 10.3390/jcm13113353. J Clin Med. 2024. PMID: 38893064 Free PMC article.
References
-
- Bender D, Sartipi K. HL7 FHIR: An Agile and RESTful approach to healthcare information exchange. Proceedings of the 26th IEEE International Symposium on Computer-Based Medical Systems. IEEE; 2013. p. 326–31. 10.1109/CBMS.2013.6627810.
-
- Hehner S, Liese K, Loos G, Möller M, Schiegnitz S, Schneider T, et al. Die Digitalisierung in deutschen Krankenhäusern - eine Chance mit Milliardenpotenzial. McKinsey & Company Healthcare Practice. 2018. https://www.mckinsey.de/publikationen/digitalisierung-chance-mit-milliar.... Accessed 15 Aug 2022.
-
- Dash P, Henricson C, Kumar P, Stern N. The hospital is dead, long live the hospital! McKinsey & Company Healthcare Systems and Services Practice. 2019. https://www.mckinsey.com/industries/healthcare-systems-and-services/our-.... Accessed 15 Aug 2022.
MeSH terms
LinkOut - more resources
Full Text Sources
Research Materials