Changes in Radiologists' Gaze Patterns Against Lung X-rays with Different Abnormalities: a Randomized Experiment
- PMID: 36622464
- PMCID: PMC9838425
- DOI: 10.1007/s10278-022-00760-2
Changes in Radiologists' Gaze Patterns Against Lung X-rays with Different Abnormalities: a Randomized Experiment
Abstract
The workload of some radiologists increased dramatically in the last several, which resulted in a potentially reduced quality of diagnosis. It was demonstrated that diagnostic accuracy of radiologists significantly reduces at the end of work shifts. The study aims to investigate how radiologists cover chest X-rays with their gaze in the presence of different chest abnormalities and high workload. We designed a randomized experiment to quantitatively assess how radiologists' image reading patterns change with the radiological workload. Four radiologists read chest X-rays on a radiological workstation equipped with an eye-tracker. The lung fields on the X-rays were automatically segmented with U-Net neural network allowing to measure the lung coverage with radiologists' gaze. The images were randomly split so that each image was shown at a different time to a different radiologist. Regression models were fit to the gaze data to calculate the treads in lung coverage for individual radiologists and chest abnormalities. For the study, a database of 400 chest X-rays with reference diagnoses was assembled. The average lung coverage with gaze ranged from 55 to 65% per radiologist. For every 100 X-rays read, the lung coverage reduced from 1.3 to 7.6% for the different radiologists. The coverage reduction trends were consistent for all abnormalities ranging from 3.4% per 100 X-rays for cardiomegaly to 4.1% per 100 X-rays for atelectasis. The more image radiologists read, the smaller part of the lung fields they cover with the gaze. This pattern is very stable for all abnormality types and is not affected by the exact order the abnormalities are viewed by radiologists. The proposed randomized experiment captured and quantified consistent changes in X-ray reading for different lung abnormalities that occur due to high workload.
Keywords: Eye-tracking; Human-AI interaction; Lung fields; Radiologist performance; U-Net.
© 2023. The Author(s) under exclusive licence to Society for Imaging Informatics in Medicine.
Conflict of interest statement
The authors declare no competing interests.
Figures
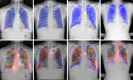
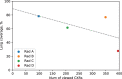
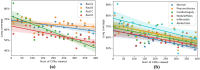
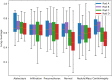
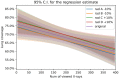
Similar articles
-
Artificial Intelligence for the Analysis of Workload-Related Changes in Radiologists' Gaze Patterns.IEEE J Biomed Health Inform. 2022 Sep;26(9):4541-4550. doi: 10.1109/JBHI.2022.3183299. Epub 2022 Sep 9. IEEE J Biomed Health Inform. 2022. PMID: 35704540
-
Performance of an AI based CAD system in solid lung nodule detection on chest phantom radiographs compared to radiology residents and fellow radiologists.J Thorac Dis. 2021 May;13(5):2728-2737. doi: 10.21037/jtd-20-3522. J Thorac Dis. 2021. PMID: 34164165 Free PMC article.
-
REFLACX, a dataset of reports and eye-tracking data for localization of abnormalities in chest x-rays.Sci Data. 2022 Jun 18;9(1):350. doi: 10.1038/s41597-022-01441-z. Sci Data. 2022. PMID: 35717401 Free PMC article.
-
The Radiologist Workload Increase; Where Is the Limit?: Mini Review and Case Study.Psychiatr Danub. 2021 Spring-Summer;33(Suppl 4):768-770. Psychiatr Danub. 2021. PMID: 34718316 Review.
-
[Imaging science and technology in diagnostic radiology: expectations in the second century of Roentgen's discovery of X-rays].Nihon Igaku Hoshasen Gakkai Zasshi. 1995 Oct;55(13):475-86. Nihon Igaku Hoshasen Gakkai Zasshi. 1995. PMID: 8721341 Review. Japanese.
Cited by
-
The Use of Machine Learning in Eye Tracking Studies in Medical Imaging: A Review.IEEE J Biomed Health Inform. 2024 Jun;28(6):3597-3612. doi: 10.1109/JBHI.2024.3371893. Epub 2024 Jun 6. IEEE J Biomed Health Inform. 2024. PMID: 38421842 Free PMC article. Review.
References
-
- E. Ranschaert, L. Topff, and O. Pianykh, Optimization of radiology workflow with artificial intelligence, Radiol. Clin., vol. 59, no. 6, Art. no. 6, Nov. 2021. 10.1016/j.rcl.2021.06.006. - PubMed
Publication types
MeSH terms
LinkOut - more resources
Full Text Sources