Industrial Anomaly Detection with Skip Autoencoder and Deep Feature Extractor
- PMID: 36502029
- PMCID: PMC9737726
- DOI: 10.3390/s22239327
Industrial Anomaly Detection with Skip Autoencoder and Deep Feature Extractor
Abstract
Over recent years, with the advances in image recognition technology for deep learning, researchers have devoted continued efforts toward importing anomaly detection technology into the production line of automatic optical detection. Although unsupervised learning helps overcome the high costs associated with labeling, the accuracy of anomaly detection still needs to be improved. Accordingly, this paper proposes a novel deep learning model for anomaly detection to overcome this bottleneck. Leveraging a powerful pre-trained feature extractor and the skip connection, the proposed method achieves better feature extraction and image reconstructing capabilities. Results reveal that the areas under the curve (AUC) for the proposed method are higher than those of previous anomaly detection models for 16 out of 17 categories. This indicates that the proposed method can realize the most appropriate adjustments to the needs of production lines in order to maximize economic benefits.
Keywords: anomaly detection; deep learning; feature extraction; industrial defect detection.
Conflict of interest statement
The authors declare no conflict of interest.
Figures
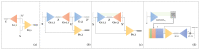
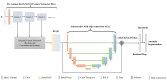
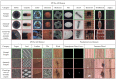
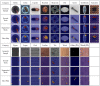
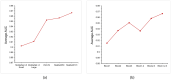
Similar articles
-
Anomaly Detection Neural Network with Dual Auto-Encoders GAN and Its Industrial Inspection Applications.Sensors (Basel). 2020 Jun 12;20(12):3336. doi: 10.3390/s20123336. Sensors (Basel). 2020. PMID: 32545489 Free PMC article.
-
Unsupervised Deep Anomaly Detection for Medical Images Using an Improved Adversarial Autoencoder.J Digit Imaging. 2022 Apr;35(2):153-161. doi: 10.1007/s10278-021-00558-8. Epub 2022 Jan 10. J Digit Imaging. 2022. PMID: 35013826 Free PMC article.
-
DOC-IDS: A Deep Learning-Based Method for Feature Extraction and Anomaly Detection in Network Traffic.Sensors (Basel). 2022 Jun 10;22(12):4405. doi: 10.3390/s22124405. Sensors (Basel). 2022. PMID: 35746191 Free PMC article.
-
3D-Convolutional Neural Network with Generative Adversarial Network and Autoencoder for Robust Anomaly Detection in Video Surveillance.Int J Neural Syst. 2020 Jun;30(6):2050034. doi: 10.1142/S0129065720500343. Epub 2020 May 28. Int J Neural Syst. 2020. PMID: 32466693
-
Anomaly Detection of GAN Industrial Image Based on Attention Feature Fusion.Sensors (Basel). 2022 Dec 29;23(1):355. doi: 10.3390/s23010355. Sensors (Basel). 2022. PMID: 36616953 Free PMC article.
Cited by
-
Two-Stream Network One-Class Classification Model for Defect Inspections.Sensors (Basel). 2023 Jun 20;23(12):5768. doi: 10.3390/s23125768. Sensors (Basel). 2023. PMID: 37420932 Free PMC article.
-
Anomaly Detection via Progressive Reconstruction and Hierarchical Feature Fusion.Sensors (Basel). 2023 Oct 27;23(21):8750. doi: 10.3390/s23218750. Sensors (Basel). 2023. PMID: 37960450 Free PMC article.
-
Image-Based Detection of Modifications in Assembled PCBs with Deep Convolutional Autoencoders.Sensors (Basel). 2023 Jan 25;23(3):1353. doi: 10.3390/s23031353. Sensors (Basel). 2023. PMID: 36772392 Free PMC article.
References
-
- Xie S., Girshick R., Dollár P., Tu Z., He K. Aggregated Residual Transformations for Deep Neural Networks; Proceedings of the IEEE Conference on Computer Vision and Pattern Recognition (CVPR); Honolulu, HI, USA. 21–26 July 2017; pp. 1492–1500.
-
- Chen Y., Li J., Xiao H., Jin X., Yan S., Feng J. Dual Path Networks. arXiv. 20171707.01629
-
- Tan M., Le Q. EfficientNet: Rethinking Model Scaling for Convolutional Neural Networks; Proceedings of the 36th International Conference on Machine Learning; Long Beach, CA, USA. 9–15 June 2019; pp. 6105–6114.
MeSH terms
Grants and funding
LinkOut - more resources
Full Text Sources