Classification of Activities of Daily Living Based on Grasp Dynamics Obtained from a Leap Motion Controller
- PMID: 36365969
- PMCID: PMC9656805
- DOI: 10.3390/s22218273
Classification of Activities of Daily Living Based on Grasp Dynamics Obtained from a Leap Motion Controller
Abstract
Stroke is one of the leading causes of mortality and disability worldwide. Several evaluation methods have been used to assess the effects of stroke on the performance of activities of daily living (ADL). However, these methods are qualitative. A first step toward developing a quantitative evaluation method is to classify different ADL tasks based on the hand grasp. In this paper, a dataset is presented that includes data collected by a leap motion controller on the hand grasps of healthy adults performing eight common ADL tasks. Then, a set of features with time and frequency domains is combined with two well-known classifiers, i.e., the support vector machine and convolutional neural network, to classify the tasks, and a classification accuracy of over 99% is achieved.
Keywords: Leap Motion Controller; activities of daily living; hand grasps classification.
Conflict of interest statement
The authors declare no conflict of interest.
Figures
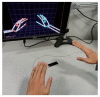
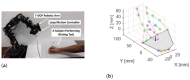
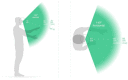
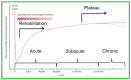

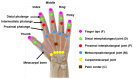
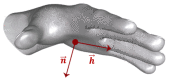
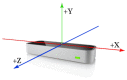
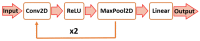
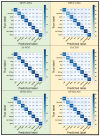
Similar articles
-
An introductory study of common grasps used by adults during performance of activities of daily living.J Hand Ther. 2014 Jul-Sep;27(3):225-33; quiz 234. doi: 10.1016/j.jht.2014.04.002. Epub 2014 Apr 21. J Hand Ther. 2014. PMID: 24878351
-
Hand gesture recognition using surface electromyography.Annu Int Conf IEEE Eng Med Biol Soc. 2020 Jul;2020:682-685. doi: 10.1109/EMBC44109.2020.9175770. Annu Int Conf IEEE Eng Med Biol Soc. 2020. PMID: 33018079
-
Objective and automatic classification of Parkinson disease with Leap Motion controller.Biomed Eng Online. 2018 Nov 12;17(1):168. doi: 10.1186/s12938-018-0600-7. Biomed Eng Online. 2018. PMID: 30419916 Free PMC article.
-
Transcranial direct current stimulation (tDCS) for improving function and activities of daily living in patients after stroke.Cochrane Database Syst Rev. 2013 Nov 15;(11):CD009645. doi: 10.1002/14651858.CD009645.pub2. Cochrane Database Syst Rev. 2013. Update in: Cochrane Database Syst Rev. 2016 Mar 21;3:CD009645. doi: 10.1002/14651858.CD009645.pub3 PMID: 24234980 Updated. Review.
-
Occupational therapy for adults with problems in activities of daily living after stroke.Cochrane Database Syst Rev. 2017 Jul 19;7(7):CD003585. doi: 10.1002/14651858.CD003585.pub3. Cochrane Database Syst Rev. 2017. PMID: 28721691 Free PMC article. Review.
Cited by
-
Development of a Three-Finger Adaptive Robotic Gripper to Assist Activities of Daily Living.Designs (Basel). 2024;8(2):10.3390/designs8020035. doi: 10.3390/designs8020035. Designs (Basel). 2024. PMID: 39286448 Free PMC article.
-
Portable Head-Mounted System for Mobile Forearm Tracking.Sensors (Basel). 2024 Mar 30;24(7):2227. doi: 10.3390/s24072227. Sensors (Basel). 2024. PMID: 38610437 Free PMC article.
References
-
- Hatem S.M., Saussez G., Della Faille M., Prist V., Zhang X., Dispa D., Bleyenheuft Y. Rehabilitation of motor function after stroke: A multiple systematic review focused on techniques to stimulate upper extremity recovery. Front. Hum. Neurosci. 2016;10:442. doi: 10.3389/fnhum.2016.00442. - DOI - PMC - PubMed
-
- [(accessed on 12 July 2017)]. Available online: http://www.strokeassociation.org/STROKEORG/AboutStroke/Impact-of-Stroke-....
-
- Duruoz M.T. Hand Function. Springer; Berlin/Heidelberg, Germany: 2016. - DOI
MeSH terms
Grants and funding
LinkOut - more resources
Full Text Sources
Medical