Can a Machine Learn from Radiologists' Visual Search Behaviour and Their Interpretation of Mammograms-a Deep-Learning Study
- PMID: 31410677
- PMCID: PMC6737161
- DOI: 10.1007/s10278-018-00174-z
Can a Machine Learn from Radiologists' Visual Search Behaviour and Their Interpretation of Mammograms-a Deep-Learning Study
Abstract
Visual search behaviour and the interpretation of mammograms have been studied for errors in breast cancer detection. We aim to ascertain whether machine-learning models can learn about radiologists' attentional level and the interpretation of mammograms. We seek to determine whether these models are practical and feasible for use in training and teaching programmes. Eight radiologists of varying experience levels in reading mammograms reviewed 120 two-view digital mammography cases (59 cancers). Their search behaviour and decisions were captured using a head-mounted eye-tracking device and software allowing them to record their decisions. This information from radiologists was used to build an ensembled machine-learning model using top-down hierarchical deep convolution neural network. Separately, a model to determine type of missed cancer (search, perception or decision-making) was also built. Analysis and comparison of variants of these models using different convolution networks with and without transfer learning were also performed. Our ensembled deep-learning network architecture can be trained to learn about radiologists' attentional level and decisions. High accuracy (95%, p value ≅ 0 [better than dumb/random model]) and high agreement between true and predicted values (kappa = 0.83) in such modelling can be achieved. Transfer learning techniques improve by < 10% with the performance of this model. We also show that spatial convolution neural networks are insufficient in determining the type of missed cancers. Ensembled hierarchical deep convolution machine-learning models are plausible in modelling radiologists' attentional level and their interpretation of mammograms. However, deep convolution networks fail to characterise the type of false-negative decisions.
Keywords: Breast cancer; Deep learning; Machine learning; Mammography; Visual search.
Figures
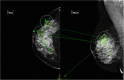
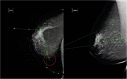
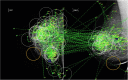
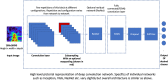
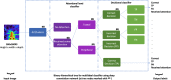

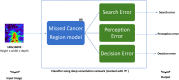

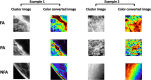
Similar articles
-
Modeling visual search behavior of breast radiologists using a deep convolution neural network.J Med Imaging (Bellingham). 2018 Jul;5(3):035502. doi: 10.1117/1.JMI.5.3.035502. Epub 2018 Aug 11. J Med Imaging (Bellingham). 2018. PMID: 30128329 Free PMC article.
-
Visual search in breast imaging.Br J Radiol. 2019 Oct;92(1102):20190057. doi: 10.1259/bjr.20190057. Epub 2019 Jul 18. Br J Radiol. 2019. PMID: 31287719 Free PMC article.
-
Evaluation of Combined Artificial Intelligence and Radiologist Assessment to Interpret Screening Mammograms.JAMA Netw Open. 2020 Mar 2;3(3):e200265. doi: 10.1001/jamanetworkopen.2020.0265. JAMA Netw Open. 2020. PMID: 32119094 Free PMC article.
-
Breast cancer detection using deep learning: Datasets, methods, and challenges ahead.Comput Biol Med. 2022 Oct;149:106073. doi: 10.1016/j.compbiomed.2022.106073. Epub 2022 Aug 31. Comput Biol Med. 2022. PMID: 36103745 Review.
-
Artificial Intelligence for Mammography and Digital Breast Tomosynthesis: Current Concepts and Future Perspectives.Radiology. 2019 Nov;293(2):246-259. doi: 10.1148/radiol.2019182627. Epub 2019 Sep 24. Radiology. 2019. PMID: 31549948 Free PMC article. Review.
Cited by
-
Reducing the number of unnecessary biopsies for mammographic BI-RADS 4 lesions through a deep transfer learning method.BMC Med Imaging. 2023 Jun 13;23(1):82. doi: 10.1186/s12880-023-01023-4. BMC Med Imaging. 2023. PMID: 37312026 Free PMC article.
-
The Use of Machine Learning in Eye Tracking Studies in Medical Imaging: A Review.IEEE J Biomed Health Inform. 2024 Jun;28(6):3597-3612. doi: 10.1109/JBHI.2024.3371893. Epub 2024 Jun 6. IEEE J Biomed Health Inform. 2024. PMID: 38421842 Free PMC article. Review.
References
-
- AIHW: Cancer in Australia 2017," in Cancer series no. 101. Cat. No. CAN 100. Canberra: AIHW, 2017
-
- (May, 2018). Australian Institute of Health and Welfare 2017. Australian Cancer Incidence and Mortality (ACIM) books: Breast Cancer. Available: https://www.aihw.gov.au/reports/cancer/acim-books
-
- S. I. Ferlay J, Ervik M, Dikshit R, Eser S, Mathers C, Rebelo M, Parkin DM, Forman D, Bray, F. (2014, 16/1/2015). GLOBOCAN 2012 v1.1, Cancer Incidence and Mortality Worldwide: IARC Cancer Base No. 11 [Internet]. Available: http://globocan.iarc.fr - PubMed
MeSH terms
LinkOut - more resources
Full Text Sources
Medical