A collaborative computer aided diagnosis (C-CAD) system with eye-tracking, sparse attentional model, and deep learning
- PMID: 30399507
- PMCID: PMC6407631
- DOI: 10.1016/j.media.2018.10.010
A collaborative computer aided diagnosis (C-CAD) system with eye-tracking, sparse attentional model, and deep learning
Abstract
Computer aided diagnosis (CAD) tools help radiologists to reduce diagnostic errors such as missing tumors and misdiagnosis. Vision researchers have been analyzing behaviors of radiologists during screening to understand how and why they miss tumors or misdiagnose. In this regard, eye-trackers have been instrumental in understanding visual search processes of radiologists. However, most relevant studies in this aspect are not compatible with realistic radiology reading rooms. In this study, we aim to develop a paradigm shifting CAD system, called collaborative CAD (C-CAD), that unifies CAD and eye-tracking systems in realistic radiology room settings. We first developed an eye-tracking interface providing radiologists with a real radiology reading room experience. Second, we propose a novel algorithm that unifies eye-tracking data and a CAD system. Specifically, we present a new graph based clustering and sparsification algorithm to transform eye-tracking data (gaze) into a graph model to interpret gaze patterns quantitatively and qualitatively. The proposed C-CAD collaborates with radiologists via eye-tracking technology and helps them to improve their diagnostic decisions. The C-CAD uses radiologists' search efficiency by processing their gaze patterns. Furthermore, the C-CAD incorporates a deep learning algorithm in a newly designed multi-task learning platform to segment and diagnose suspicious areas simultaneously. The proposed C-CAD system has been tested in a lung cancer screening experiment with multiple radiologists, reading low dose chest CTs. Promising results support the efficiency, accuracy and applicability of the proposed C-CAD system in a real radiology room setting. We have also shown that our framework is generalizable to more complex applications such as prostate cancer screening with multi-parametric magnetic resonance imaging (mp-MRI).
Keywords: Attention; Eye-tracking; Graph sparsification; Lung cancer screening; Multi-task deep learning; Prostate cancer screening.
Copyright © 2018 Elsevier B.V. All rights reserved.
Figures
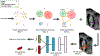
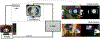
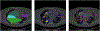

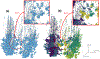
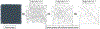
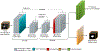
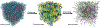
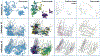
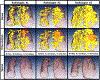
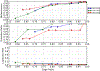
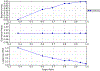
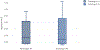

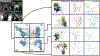
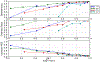
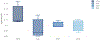
Similar articles
-
Visual search in breast imaging.Br J Radiol. 2019 Oct;92(1102):20190057. doi: 10.1259/bjr.20190057. Epub 2019 Jul 18. Br J Radiol. 2019. PMID: 31287719 Free PMC article.
-
A Novel Deep Learning Based Computer-Aided Diagnosis System Improves the Accuracy and Efficiency of Radiologists in Reading Biparametric Magnetic Resonance Images of the Prostate: Results of a Multireader, Multicase Study.Invest Radiol. 2021 Oct 1;56(10):605-613. doi: 10.1097/RLI.0000000000000780. Invest Radiol. 2021. PMID: 33787537
-
Evaluating the performance of a deep learning-based computer-aided diagnosis (DL-CAD) system for detecting and characterizing lung nodules: Comparison with the performance of double reading by radiologists.Thorac Cancer. 2019 Feb;10(2):183-192. doi: 10.1111/1759-7714.12931. Epub 2018 Dec 8. Thorac Cancer. 2019. PMID: 30536611 Free PMC article.
-
Shedding light on ai in radiology: A systematic review and taxonomy of eye gaze-driven interpretability in deep learning.Eur J Radiol. 2024 Mar;172:111341. doi: 10.1016/j.ejrad.2024.111341. Epub 2024 Feb 1. Eur J Radiol. 2024. PMID: 38340426 Review.
-
Convolutional neural networks for computer-aided detection or diagnosis in medical image analysis: An overview.Math Biosci Eng. 2019 Jul 15;16(6):6536-6561. doi: 10.3934/mbe.2019326. Math Biosci Eng. 2019. PMID: 31698575 Review.
Cited by
-
REFLACX, a dataset of reports and eye-tracking data for localization of abnormalities in chest x-rays.Sci Data. 2022 Jun 18;9(1):350. doi: 10.1038/s41597-022-01441-z. Sci Data. 2022. PMID: 35717401 Free PMC article.
-
Artificial intelligence for early detection of renal cancer in computed tomography: A review.Camb Prism Precis Med. 2022 Nov 11;1:e4. doi: 10.1017/pcm.2022.9. eCollection 2023. Camb Prism Precis Med. 2022. PMID: 38550952 Free PMC article. Review.
-
Development and multicenter validation of chest X-ray radiography interpretations based on natural language processing.Commun Med (Lond). 2021 Oct 28;1:43. doi: 10.1038/s43856-021-00043-x. eCollection 2021. Commun Med (Lond). 2021. PMID: 35602222 Free PMC article.
-
Deep learning applications in pulmonary medical imaging: recent updates and insights on COVID-19.Mach Vis Appl. 2020;31(6):53. doi: 10.1007/s00138-020-01101-5. Epub 2020 Jul 28. Mach Vis Appl. 2020. PMID: 32834523 Free PMC article.
-
A review of machine learning in scanpath analysis for passive gaze-based interaction.Front Artif Intell. 2024 Jun 5;7:1391745. doi: 10.3389/frai.2024.1391745. eCollection 2024. Front Artif Intell. 2024. PMID: 38903158 Free PMC article. Review.
References
-
- Al Mohammad B, Brennan P, Mello-Thoms C, 2017. A review of lung cancer screening and the role of computer-aided detection. Clinical Radiology 72, 433–442. - PubMed
-
- Al-Moteri MO, Symmons M, Plummer V, Cooper S, 2017. Eye tracking to investigate cue processing in medical decision-making: A scoping review. Computers in Human Behavior 66, 52–66.
-
- Badrinarayanan V, Kendall A, Cipolla R, 2017. Segnet: A deep convolutional encoder-decoder architecture for scene segmentation. IEEE transactions on pattern analysis and machine intelligence. - PubMed
-
- Buty M, Xu Z, Gao M, Bagci U, Wu A, Mollura DJ, 2016. Characterization of lung nodule malignancy using hybrid shape and appearance features, in: International Conference on Medical Image Computing and Computer-Assisted Intervention, Springer; pp. 662–670.
MeSH terms
Grants and funding
LinkOut - more resources
Full Text Sources
Other Literature Sources
Medical
Research Materials
Miscellaneous