Evaluating Hyperspectral Vegetation Indices for Leaf Area Index Estimation of Oryza sativa L. at Diverse Phenological Stages
- PMID: 28588596
- PMCID: PMC5438995
- DOI: 10.3389/fpls.2017.00820
Evaluating Hyperspectral Vegetation Indices for Leaf Area Index Estimation of Oryza sativa L. at Diverse Phenological Stages
Abstract
Hyperspectral reflectance derived vegetation indices (VIs) are used for non-destructive leaf area index (LAI) monitoring for precise and efficient N nutrition management. This study tested the hypothesis that there is potential for using various hyperspectral VIs for estimating LAI at different growth stages of rice under varying N rates. Hyperspectral reflectance and crop canopy LAI measurements were carried out over 2 years (2015 and 2016) in Meichuan, Hubei, China. Different N fertilization, 0, 45, 82, 127, 165, 210, 247, and 292 kg ha-1, were applied to generate various scales of VIs and LAI values. Regression models were used to perform quantitative analyses between spectral VIs and LAI measured under different phenological stages. In addition, the coefficient of determination and RMSE were employed to evaluate these models. Among the nine VIs, the ratio vegetation index, normalized difference vegetation index (NDVI), modified soil-adjusted vegetation index (MSAVI), modified triangular vegetation index (MTVI2) and exhibited strong and significant relationships with the LAI estimation at different phenological stages. The enhanced vegetation index performed moderately. However, the green normalized vegetation index and blue normalized vegetation index confirmed that there is potential for crop LAI estimation at early phenological stages; the soil-adjusted vegetation index and optimized soil-adjusted vegetation index were more related to the soil optical properties, which were predicted to be the least accurate for LAI estimation. The noise equivalent accounted for the sensitivity of the VIs and MSAVI, MTVI2, and NDVI for the LAI estimation at phenological stages. The results note that LAI at different crop phenological stages has a significant influence on the potential of hyperspectral derived VIs under different N management practices.
Keywords: LAI; N-nutrition; hyperspectral reflectance; phenology; rice.
Figures
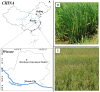
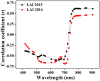
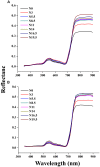
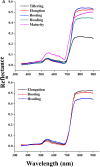
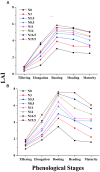
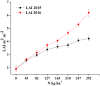
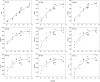
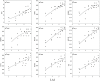
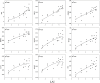
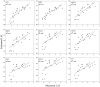
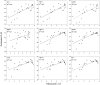
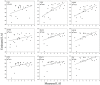
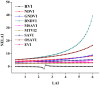
Similar articles
-
[The estimation model of rice leaf area index using hyperspectral data based on support vector machine].Guang Pu Xue Yu Guang Pu Fen Xi. 2008 Aug;28(8):1837-41. Guang Pu Xue Yu Guang Pu Fen Xi. 2008. PMID: 18975815 Chinese.
-
Estimation of Dynamic Canopy Variables Using Hyperspectral Derived Vegetation Indices Under Varying N Rates at Diverse Phenological Stages of Rice.Front Plant Sci. 2019 Jan 15;9:1883. doi: 10.3389/fpls.2018.01883. eCollection 2018. Front Plant Sci. 2019. PMID: 30697219 Free PMC article.
-
[Inversion of leaf area index during different growth stages in winter wheat].Guang Pu Xue Yu Guang Pu Fen Xi. 2013 Sep;33(9):2546-52. Guang Pu Xue Yu Guang Pu Fen Xi. 2013. PMID: 24369669 Chinese.
-
Applications of a Hyperspectral Imaging System Used to Estimate Wheat Grain Protein: A Review.Front Plant Sci. 2022 Apr 8;13:837200. doi: 10.3389/fpls.2022.837200. eCollection 2022. Front Plant Sci. 2022. PMID: 35463397 Free PMC article. Review.
-
Hyperspectral remote sensing for tobacco quality estimation, yield prediction, and stress detection: A review of applications and methods.Front Plant Sci. 2023 Mar 8;14:1073346. doi: 10.3389/fpls.2023.1073346. eCollection 2023. Front Plant Sci. 2023. PMID: 36968402 Free PMC article. Review.
Cited by
-
Hyperspectral Reflectance Response of Wild Rocket (Diplotaxis tenuifolia) Baby-Leaf to Bio-Based Disease Resistance Inducers Using a Linear Mixed Effect Model.Plants (Basel). 2021 Nov 25;10(12):2575. doi: 10.3390/plants10122575. Plants (Basel). 2021. PMID: 34961046 Free PMC article.
-
Inversion of Nitrogen Concentration in Apple Canopy Based on UAV Hyperspectral Images.Sensors (Basel). 2022 May 4;22(9):3503. doi: 10.3390/s22093503. Sensors (Basel). 2022. PMID: 35591193 Free PMC article.
-
Quantifying physiological trait variation with automated hyperspectral imaging in rice.Front Plant Sci. 2023 Sep 20;14:1229161. doi: 10.3389/fpls.2023.1229161. eCollection 2023. Front Plant Sci. 2023. PMID: 37799551 Free PMC article.
-
Estimation of Rice Aboveground Biomass by UAV Imagery with Photosynthetic Accumulation Models.Plant Phenomics. 2023 May 31;5:0056. doi: 10.34133/plantphenomics.0056. eCollection 2023. Plant Phenomics. 2023. PMID: 37273463 Free PMC article.
-
IoT-Based Systems for Soil Nutrients Assessment in Horticulture.Sensors (Basel). 2022 Dec 30;23(1):403. doi: 10.3390/s23010403. Sensors (Basel). 2022. PMID: 36617000 Free PMC article. Review.
References
-
- Abdel-Rahman E. M., Ahmed F. B., van den Berg M. (2010). Estimation of sugarcane leaf nitrogen concentration using in situ spectroscopy. Int. J. Appl. Earth Obs. 12 S52–S57. 10.1016/j.jag.2009.11.003 - DOI
-
- Bajwa S. G., Mishra A. R., Norman R. J. (2010). Canopy reflectance response to plant nitrogen accumulation in rice. Precis. Agric. 11 488–506. 10.1007/s11119-009-9142-0 - DOI
-
- Broge N. H., Leblanc E. (2000). Comparing prediction power and stability of broadband and hyperspectral vegetation indices for estimation of green leaf area index and canopy chlorophyll density. Remote Sens. Environ. 76 156–172. 10.1016/0034-4257(95)00132-K - DOI
-
- Casa R., Varella H., Buis S., Guérif M., De Solan B., Baret F. (2012). Forcing a wheat crop model with LAI data to access agronomic variables: evaluation of the impact of model and LAI uncertainties and comparison with an empirical approach. Eur. J. Agron. 37 1–10. 10.1016/j.eja.2011.09.004 - DOI
LinkOut - more resources
Full Text Sources
Other Literature Sources
Research Materials