Abstract
Author networks in science often rely on citation analyses. In such cases, as in others, network interpretation usually depends on supplementary data, notably about authors’ research domains when disciplinary interpretations are sought. More general social networks also face similar interpretation challenges as to the semantic content specificities of their members. In this research-in-progress, we propose to infer author networks not from citation analyses but from topic similarity analyses based on a topic-model of published documents. Such author networks reveal, as we call them, “hidden communities of interest” (HCoIs) whose semantic content can easily be interpreted by means of their associated topics in the model. We use an astrobiology corpus of full-text articles (N = 3,698) to illustrate the approach. Having conducted an LDA topic-model on all publications, we identify the underlying communities of authors by measuring author correlations in terms of topic distributions. Adding publication dates makes it possible to examine HCoI evolution over time. This approach to social networks supplements traditional methods in contexts where textual data are available.
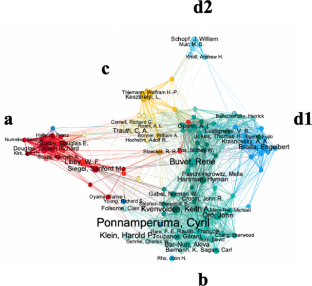

Similar content being viewed by others
Abbreviations
- HCoI:
-
Hidden communities of interest
- SNA:
-
Social network analysis
- LDA:
-
Latent Dirichlet analysis
References
Angelov, D. (2020). Top2Vec: Distributed representations of topics (arXiv:2008.09470). http://arxiv.org/abs/2008.09470
Arbelaitz, O., Gurrutxaga, I., Muguerza, J., Pérez, J. M., & Perona, I. (2013). An extensive comparative study of cluster validity indices. Pattern Recognition, 46(1), 243–256. https://doi.org/10.1016/j.patcog.2012.07.021
Bastian, M., Heymann, S., & Jacomy, M. (2009). Gephi: An open source software for exploring and manipulating networks. International Conference on Weblogs and Social Media. https://doi.org/10.1609/icwsm.v3i1.13937
Beyer, K., Goldstein, J., Ramakrishnan, R., & Shaft, U. (1999). When is “nearest neighbor” meaningful? International Conference on Database Theory. https://doi.org/10.1007/3-540-49257-7_15
Blei, D. M., Ng, A. Y., & Jordan, M. I. (2003). Latent dirichlet allocation. Journal of Machine Learning Research, 3, 993–1022.
Boyack, K. W., Klavans, R., & Börner, K. (2005). Mapping the backbone of science. Scientometrics, 64(3), 351–374. https://doi.org/10.1007/s11192-005-0255-6
Boyd-Graber, J. L., Hu, Y., & Mimno, D. (2017). Applications of topic models. Foundations and Trends in Information Retrieval. https://doi.org/10.1561/1500000030
Carley, K. (1993). Coding choices for textual analysis: A comparison of content analysis and map analysis. Sociological Methodology, 23, 75–126. https://doi.org/10.2307/271007
Castelblanco, G., Guevara, J., Mesa, H., & Sanchez, A. (2021). Semantic network analysis of literature on public-private partnerships. Journal of Construction Engineering and Management, 147(5), 04021033. https://doi.org/10.1061/(ASCE)CO.1943-7862.0002041
Christensen, A. P., & Kenett, Y. N. (2023). Semantic network analysis (SemNA): A tutorial on preprocessing, estimating, and analyzing semantic networks. Psychological Methods, 28(4), 860–879. https://doi.org/10.1037/met0000463
Crane, D. (1969). Social structure in a group of scientists: A test of the “invisible college” hypothesis. American Sociological Review, 34(3), 335. https://doi.org/10.2307/2092499
Danowski, J. A. (1993). Network analysis of message content. In W. D. Richards & G. A. Barnett (Eds.), Progress in communication sciences. Ablex Publishing Corporation.
Danowski, J. A. (2011). Counterterrorism mining for individuals semantically-similar to watchlist members. In U. K. Wiil (Ed.), Counterterrorism and open source intelligence. Springer.
Danowski, J. A., & Cepela, N. (2010). Automatic mapping of social networks of actors from text corpora: Time series analysis. In N. Memon, J. J. Xu, D. L. Hicks, & H. Chen (Eds.), Data mining for social network data. Springer.
Danowski, J. A., Van Klyton, A., Tavera-Mesías, J. F., Duque, K., Radwan, A., & Rutabayiro-Ngoga, S. (2023). Policy semantic networks associated with ICT utilization in Africa. Social Network Analysis and Mining, 13(1), 73. https://doi.org/10.1007/s13278-023-01068-x
de Vries, E., Schoonvelde, M., & Schumacher, G. (2018). No longer lost in translation: Evidence that Google translate works for comparative bag-of-words text applications. Political Analysis, 26(4), 417–430. https://doi.org/10.1017/pan.2018.26
Dick, S. J., & Strick, J. E. (2004). The living universe NASA and the development of astrobiology. Rutgers University Press.
Diesner, J., & Carley, K. M. (2004). Using network text analysis to detect the organizational structure of covert networks. In Proceedings of the North American Association for Computational Social and Organizational Science (NAACSOS) Conference (Vol. 3). Pittsburgh: NAACSOS.
DiMaggio, P., Nag, M., & Blei, D. (2013). Exploiting affinities between topic modeling and the sociological perspective on culture: Application to newspaper coverage of U.S. government arts funding. Poetics, 41(6), 570–606. https://doi.org/10.1016/j.poetic.2013.08.004
Doerfel, M. L., & Barnett, G. A. (1999). A semantic network analysis of the international communication association. Human Communication Research, 25(4), 589–603. https://doi.org/10.1111/j.1468-2958.1999.tb00463.x
Field, A. P. (2009). Discovering statistics using SPSS: And sex, drugs and rock “n” roll. SAGE Publications.
Firth, J. R. (1957). A synopsis of linguistic theory 1930–1955. In J. R. Firth (Ed.), Studies in linguistic analysis (pp. 1–32). Blackwell.
Fortunato, S., Bergstrom, C. T., Börner, K., Evans, J. A., Helbing, D., Milojević, S., Petersen, A. M., Radicchi, F., Sinatra, R., Uzzi, B., Vespignani, A., Waltman, L., Wang, D., & Barabási, A.-L. (2018). Science of science. Science. https://doi.org/10.1126/science.aao0185
Griffiths, T. L., & Steyvers, M. (2004). Finding scientific topics. Proceedings of the National Academy of Sciences, 101, 5228–5235. https://doi.org/10.1073/pnas.0307752101
Harris, Z. S. (1954). Distributional structure. Word, 10(2–3), 146–162. https://doi.org/10.1080/00437956.1954.11659520
Horneck, G., Walter, N., Westall, F., Grenfell, J. L., Martin, W. F., Gomez, F., Leuko, S., Lee, N., Onofri, S., Tsiganis, K., Saladino, R., Pilat-Lohinger, E., Palomba, E., Harrison, J., Rull, F., Muller, C., Strazzulla, G., Brucato, J. R., Rettberg, P., & Capria, M. T. (2016). AstRoMap European astrobiology roadmap. Astrobiology, 16(3), 201–243. https://doi.org/10.1089/ast.2015.1441
Kherwa, P., & Bansal, P. (2020). Topic modeling: A comprehensive review. EAI Endorsed Transactions on Scalable Information Systems. https://doi.org/10.4108/eai.13-7-2018.159623
Malaterre, C., & Lareau, F. (2022). The early days of contemporary philosophy of science: Novel insights from machine translation and topic-modeling of non-parallel multilingual corpora. Synthese, 200(3), 242. https://doi.org/10.1007/s11229-022-03722-x
Malaterre, C., & Lareau, F. (2023). The emergence of astrobiology: A topic-modeling perspective. Astrobiology, 23(5), 496–512. https://doi.org/10.1089/ast.2022.0122
Des Marais, D. J., Allamandola, L. J., Benner, S. A., Boss, A. P., Deamer, D., Falkowski, P. G., Farmer, J. D., Hedges, S. B., Jakosky, B. M., Knoll, A. H., Liskowsky, D. R., Meadows, V. S., Meyer, M. A., Pilcher, C. B., Nealson, K. H., Spormann, A. M., Trent, J. D., Turner, W. W., Woolf, N. J., & Yorke, H. W. (2003). The NASA astrobiology roadmap. Astrobiology, 3(2), 219–235. https://doi.org/10.1089/153110703769016299
McCallum, A., Wang, X., & Corrada-Emmanuel, A. (2007). Topic and role discovery in social networks with experiments on enron and academic email. Journal of Artificial Intelligence Research, 30, 249–272. https://doi.org/10.1613/jair.2229
Röder, M., Both, A., & Hinneburg, A. (2015). Exploring the space of topic coherence measures. Proceedings of the Eighth ACM International Conference on Web Search and Data Mining-WSDM ’15. https://doi.org/10.1145/2684822.2685324
Schmid, H. (1994). Probabilistic part-of-speech tagging using decision trees. Proceedings of International Conference on New Methods in Language Processing, 44–49.
Segev, E. (2021). Semantic network analysis in social sciences. Routledge.
Siew, C. S. Q., Wulff, D. U., Beckage, N. M., & Kenett, Y. N. (2019). Cognitive network science: A review of research on cognition through the lens of network representations, processes, and dynamics. Complexity, 2019, e2108423. https://doi.org/10.1155/2019/2108423
Steyvers, M., Smyth, P., Rosen-Zvi, M., & Griffiths, T. (2004). Probabilistic author-topic models for information discovery. Proceedings of the of the 2004 ACM SIGKDD International Conference on Knowledge Discovery and Data Mining-KDD ’04. https://doi.org/10.1145/1014052.1014087
Ye, F., Chen, C., & Zheng, Z. (2018). Deep autoencoder-like nonnegative matrix factorization for community detection. Proceedings of the 27th ACM International Conference on Information and Knowledge Management, 1393–1402.
Zhang, H., Qiu, B., Giles, C. L., Foley, H. C., & Yen, J. (2007). An LDA-based community structure discovery approach for large-scale social networks. 2007 IEEE Intelligence and Security Informatics, 200–207
Zhao, W., Chen, J. J., Perkins, R., Liu, Z., Ge, W., Ding, Y., & Zou, W. (2015). A heuristic approach to determine an appropriate number of topics in topic modeling. BMC Bioinformatics. https://doi.org/10.1186/1471-2105-16-S13-S8
Acknowledgements
C.M. acknowledges funding from Canada Social Sciences and Humanities Research Council (Grant 430-2018-00899) and Canada Research Chairs (CRC-950-230795). F.L. acknowledges funding from the Canada Social Sciences and Humanities Research Council (756-2024-0557) and the Canada Research Chair in Philosophy of the Life Sciences at UQAM. The authors thank the audience of ISSI 2023 for most helpful comments on an earlier version of this paper published in the conference proceedings as: Malaterre, C., & Lareau, F. (2023). Visualizing hidden communities of interest: A preliminary analysis of topic-based social networks in astrobiology. Proceedings of ISSI 2023. The 19th Conference of the International Society for Scientometrics and Informetrics, Bloomington, IN.
Author information
Authors and Affiliations
Contributions
Conceptualization: CM, FL; Data curation: FL; Formal analysis and investigation: CM, FL; Funding acquisition: CM; Investigation: CM, FL; Methodology: CM, FL; Project administration: CM; Resources: CM; Software: FL; Supervision: CM; Validation: CM, FL; Visualization: CM; Writing – original draft preparation: CM; Writing—review and editing: CM, FL. Both authors approved the final submitted manuscript.
Corresponding author
Ethics declarations
Conflict of interest
The authors declare no competing interests.
Additional information
Publisher's Note
Springer Nature remains neutral with regard to jurisdictional claims in published maps and institutional affiliations.
Rights and permissions
Springer Nature or its licensor (e.g. a society or other partner) holds exclusive rights to this article under a publishing agreement with the author(s) or other rightsholder(s); author self-archiving of the accepted manuscript version of this article is solely governed by the terms of such publishing agreement and applicable law.
About this article
Cite this article
Malaterre, C., Lareau, F. Visualizing hidden communities of interest: A case-study analysis of topic-based social networks in astrobiology. Scientometrics 129, 6167–6181 (2024). https://doi.org/10.1007/s11192-024-05047-7
Received:
Accepted:
Published:
Issue Date:
DOI: https://doi.org/10.1007/s11192-024-05047-7