Abstract
This paper presents a recurrent fuzzy-neural filter for adaptive noise cancelation. The cancelation task is transformed to a system-identification problem, which is tackled by use of the dynamic neuron-based fuzzy neural network (DN-FNN). The fuzzy model is based on Takagi–Sugeno–Kang fuzzy rules, whose consequent parts consist of linear combinations of dynamic neurons. The orthogonal least squares method is employed to select the number of rules, along with the number and kind of dynamic neurons that participate in each rule. Extensive simulation results are given and performance comparison with a series of other dynamic fuzzy and neural models is conducted, underlining the effectiveness of the proposed filter and its superior performance over its competing rivals.






Similar content being viewed by others
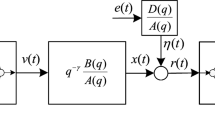
Explore related subjects
Discover the latest articles, news and stories from top researchers in related subjects.References
Wiener N (1949) Extrapolation, interpolation and smoothing of stationary time series, with engineering applications. Wiley, New York
Bode H, Shannon C (1950) A simplified derivation of linear least squares smoothing and prediction theory. Proc IRE 38:417–425
Kalman R, Bucy R (1961) New results in linear filtering and prediction theory. Trans ACME 83:95–107
Feintuch PL (1976) An adaptive recursive LMS filter. IEEE Proc 64:1622–1624
Widrow B et al (1975) Adaptive noise cancellation: principles and applications. IEEE Proc 63:1692–1716
Coker MJ, Simkins DN (1980) A nonlinear adaptive noise canceller. In: Proceedings of IEEE international conference on acoustics, speech, and signal processing, pp 470–483
Sicuranza GL, Bucconi A, Mitri P (1984) Adaptive echo cancellation with nonlinear digital filter. In: Proceedings of IEEE international conference acoustics, speech, and signal processing, pp 1–44
Billings SA, El-Hitmy M (1990) Adaptive noise cancellation for a class of nonlinear IIR filters. J Sound Vibration 140:457–473
Leontaritis LJ, Billings SA (1985) Input output parametric models for nonlinear systems, part II: Deterministic nonlinear systems. Int J Control 45:303–344
Billings SA, Fung CF (1995) Recurrent radial basis function networks for adaptive noise cancellation. Neural Netw 8:273–290
Jang J-SR, Sun C-T, Mizutani E (1997) Neuro-fuzzy and soft computing. Prentice Hall, New Jersey
Lin C-T, Juang C-F (1997) An Adaptive neural fuzzy filter and its applications. IEEE Trans Syst Man Cybern B 27:635–656
Wang L-X, Mendel JM (1993) Fuzzy adaptive filters with application to nonlinear channel equalization. IEEE Trans Fuzzy Syst 1:161–170
Mastorocostas PA, Theocharis JB (2002) A recurrent fuzzy neural model for dynamic system identification. IEEE Trans Syst Man Cybern B 32:176–190
Mastorocostas PA, Theocharis JB (2003) An orthogonal least squares method for recurrent fuzzy-neural modeling. Fuzzy Sets Syst 140:285–300
Takagi T, Sugeno M (1985) Fuzzy identification of systems and its applications. IEEE Trans Syst Man Cybern 15:116–132
Frasconi P, Gori M, Soda G (1992) Local feedback multilayered networks. Neural Comput 4:120–130
Tsoi AC, Back AD (1994) Locally recurrent globally feedforward networks: a critical review of architectures. IEEE Trans Neural Netw 5:229–239
Wang L-X, Mendel JM (1992) Fuzzy basis functions, universal approximation, and orthogonal least-squares learning. IEEE Trans Neural Netw 3:807–814
Acknowledgments
The Project is co-funded by the European Social Fund and National Resources—(EPEAEK–II) ARXIMHDHS.
Author information
Authors and Affiliations
Corresponding author
Rights and permissions
About this article
Cite this article
Mastorocostas, P., Varsamis, D., Hilas, C. et al. A generalized Takagi–Sugeno–Kang recurrent fuzzy-neural filter for adaptive noise cancelation. Neural Comput & Applic 17, 521–529 (2008). https://doi.org/10.1007/s00521-007-0129-3
Received:
Accepted:
Published:
Issue Date:
DOI: https://doi.org/10.1007/s00521-007-0129-3