Abstract
Currently, the state of a house is typically assessed by an expert, which is time and resource intensive. Therefore, an automatic assessment could have economic, social and ecological benefits. Hence, this study presents a binary classification model using transfer learning to classify Google Street View images of houses. For this purpose, a three-by-three analysis is conducted that allows to compare three different network architectures and three differently-sized data sets, using properties located in Leuven, Belgium. A DenseNet201 architecture was found to work best, as illustrated quantitatively as well as by means of state-of-the-art explainability methods.
This work was co-sponsored by a research chair (“Real Estate Analytics”) granted by the Royal Federation of Belgian Notaries (Fednot).
Access this chapter
Tax calculation will be finalised at checkout
Purchases are for personal use only
Similar content being viewed by others
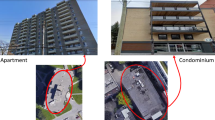
References
Buda, M., Maki, A., Mazurowski, M.A.: A systematic study of the class imbalance problem in convolutional neural networks. Neural Netw. 106, 249–259 (2018)
Campbell, A., Both, A., Sun, Q.C.: Detecting and mapping traffic signs from Google Street View images using deep learning and GIS. Comput. Environ. Urban Syst. 77 (2019). Article no. 101350
Deng, J., Dong, W., Socher, R., Li, L.J., Li, K., Fei-Fei, L.: ImageNet: a large-scale hierarchical image database. In: 2009 IEEE CVPR, pp. 248–255. IEEE Computer Society, Washington, DC, USA, June 2009
Gebru, T., et al.: Using deep learning and Google street view to estimate the demographic makeup of neighborhoods across the United States. In: Proceedings of the National Academy of Sciences, vol. 114, no. 50, pp. 13108–13113, December 2017
Hastie, T., Tibshirani, R., Friedman, J.H., Friedman, J.H.: The Elements of Statistical Learning: Data Mining, Inference, and Prediction, vol. 2. Springer, Cham (2009). https://doi.org/10.1007/978-0-387-21606-5
He, K., Zhang, X., Ren, S., Sun, J.: Identity mappings in deep residual networks. CoRR abs/1603.05027, pp. 630–645, March 2016
Heylen, K., Vanderstraeten, L.: Wonen in Vlaanderen anno 2018. Gompel & Svacina, Sint-Niklaas (2019)
Huang, G., Liu, Z., Maaten, L.V.D., Weinberger, K.Q.: Densely connected convolutional networks. CoRR abs/1608.06993, pp. 2261–2269 (2016)
Ioffe, S., Szegedy, C.: Batch normalization: accelerating deep network training by reducing internal covariate shift. In: 32nd International Conference on Machine Learning, ICML 2015, vol. 1, pp. 448–456, February 2015
Kang, J., Körner, M., Wang, Y., Taubenböck, H., Zhu, X.X.: Building instance classification using street view images. ISPRS J. Photogramm. Remote. Sens. 145, 44–59 (2018)
Krawczyk, B.: Learning from imbalanced data: open challenges and future directions. Progress Artif. Intell. 5(4), 221–232 (2016). Article no. 101350. https://doi.org/10.1007/s13748-016-0094-0
Krizhevsky, A., Sutskever, I., Hinton, G.E.: ImageNet classification with deep convolutional neural networks. In: Pereira, F., Burges, C.J.C., Bottou, L., Weinberger, K.Q. (eds.) Advances in Neural Information Processing Systems, vol. 25. Curran Associates Inc, Red Hook (2012)
Law, S., Paige, B., Russell, C.: Take a look around: using street view and satellite images to estimate house prices. ACM Trans. Intell. Syst. Technol. 10(5), 1–19 (2019)
Li, Y., Chen, Y., Rajabifard, A., Khoshelham, K., Aleksandrov, M.: Estimating building age from google street view images using deep learning. In: Winter, S., Griffin, A., Sester, M. (eds.) GIScience 2018, vol. 114, pp. 1–40. Schloss Dagstuhl-Leibniz-Zentrum fuer Informatik, Dagstuhl (2018)
Liu, S., Deng, W.: Very deep convolutional neural network based image classification using small training sample size. In: 2015 3rd IAPR Asian Conference on Pattern Recognition (ACPR), pp. 730–734. IEEE Computer Society, Washington, DC, USA, November 2015
Perez, H., Tah, J.H.M., Mosavi, A.: Deep learning for detecting building defects using convolutional neural networks. Sensors (Switzerland) 19(16), 3556 (2019)
Ribeiro, M.T., Singh, S., Guestrin, C.: “why should I trust you?": Explaining the predictions of any classifier. CoRR abs/1602.04938 (2016)
Shu, M.: Deep learning for image classification on very small datasets using transfer learning. Creative Components 345, 14–21 (2019)
Simonyan, K., Zisserman, A.: Very deep convolutional networks for large-scale image recognition. In: Bengio, Y., LeCu, Y. (eds.) 3rd International Conference on Learning Representations. ICLR, La Jolla, CA, USA (2015)
Srivastava, N., Hinton, G., Krizhevsky, A., Sutskever, I., Salakhutdinov, R.: Dropout: a simple way to prevent neural networks from overfitting. J. Mach. Learn. Res. 15, 1929–1958 (2014). Article no. 101350
Tavakkoli, V., Mohsenzadegan, K., Kyamakya, K.: A visual sensing concept for robustly classifying house types through a convolutional neural network architecture involving a multi-channel features extraction. Sensors (Switzerland) 20(19), 1–16 (2020)
Ustaoglu, A., Yaras, A., Sutcu, M., Gencel, O.: Investigation of the residential building having novel environment-friendly construction materials with enhanced energy performance in diverse climate regions: Cost-efficient, low-energy and low-carbon emission. J. Build. Eng. 43, 102617 (2021)
Wojna, Z., et al.: Attention-based extraction of structured information from street view imagery. In: Proceedings of the International Conference on Document Analysis and Recognition, ICDAR, vol. 1, pp. 844–850 (2017)
Author information
Authors and Affiliations
Corresponding author
Editor information
Editors and Affiliations
Rights and permissions
Copyright information
© 2022 The Author(s), under exclusive license to Springer Nature Switzerland AG
About this paper
Cite this paper
Geerts, M., Shaikh, K., De Weerdt, J., Broucke, S.V. (2022). Predicting the State of a House Using Google Street View. In: Guizzardi, R., Ralyté, J., Franch, X. (eds) Research Challenges in Information Science. RCIS 2022. Lecture Notes in Business Information Processing, vol 446. Springer, Cham. https://doi.org/10.1007/978-3-031-05760-1_46
Download citation
DOI: https://doi.org/10.1007/978-3-031-05760-1_46
Published:
Publisher Name: Springer, Cham
Print ISBN: 978-3-031-05759-5
Online ISBN: 978-3-031-05760-1
eBook Packages: Computer ScienceComputer Science (R0)