Abstract
This paper presents the rule generation method based on evolutionary computation and rough set, which integrates the procedure of discretization and reduction using information entropy-based uncertainty measures and evolutionary computation. Based on the definitions of certain rules and approximate certain rules, the paper focuses on the reduction by meanings of evolutionary computation. Experimental results reveal that the proposed method leads to better classification quality and smaller number of decision rules comparing with other methods.
Preview
Unable to display preview. Download preview PDF.
Similar content being viewed by others
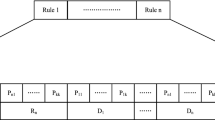
References
Polkowski, L., Skowron, A. (eds.): Rough Sets and Knowledge discovery 1, 2. Physica Verlag, Heidelberg (1998)
Tsumoto, S.: Automated Discovery of Positive and Negative Knowledge in Clinical Databases based on Rough Set Model. IEEE EMB Magazine, 56–62 (2000)
Yao, J.T., Yao, Y.Y.: Induction of Classification Rules by Granular Computing. In: Alpigini, J.J., Peters, J.F., Skowron, A., Zhong, N. (eds.) RSCTC 2002. LNCS (LNAI), vol. 2475, pp. 331–338. Springer, Heidelberg (2002)
Sankar, K.P., Sushmita, M., Pabitra, M.L.: Rough-Fuzzy MLP: Modular Evolution, Rule Generation, and Evaluation. IEEE Trans. Knowledge and Data Engineering 15, 14–25 (2003)
RSES. Rough Set Exploration System ver. 2.1.1 Institute of Mathematics, Warsaw University, Poland (2004), http://logic.mimuw.edu.pl/~rses
Pawlak, Z.: Rough Sets. Theoretical Aspects of Reasoning about Data. Kluwer Acasemic Publisher, Dordrecht (1991)
Salvatore, G., Zdzislaw, P., Roman, S.: Generalized Decision Algorithms, Rough Inference Rules, and Flow Graphs. In: Alpigini, J.J., Peters, J.F., Skowron, A., Zhong, N. (eds.) RSCTC 2002. LNCS (LNAI), vol. 2475, pp. 93–104. Springer, Heidelberg (2002)
Wang, G.Y.: Algebra View and Information View of Rough Sets Theory. In: Dasarathy, B.V. (ed.) Data Miming and Knowledge Discovery: Theory, Tools, and Technology III. Proceedings of SPIE, vol. 4384, pp. 200–207 (2001)
Rissanen, J.: Minimum-Discription-Length Principle. In: Kotz, S., Johnson, N.L. (eds.) Encyclopedia of Statistical Science, pp. 523–527. Wiley, New York (1985)
GrÄunwald, P., Myung, P.: Advances in Minimum Description Length: Theory and Applications. MIT Press, Cambridge (2004)
Ivo, D., Guncher, G.: Uncertainty Measures of Rough Set Prediction. Artificial Intelligence 106, 109–137 (1998)
Han, B., Wu, T.-J., Yang, M.-H.: Reduct Algorithm Based on Information Entropy and Rough Set Theory. Journal of Circuits and Systems 7(2), 96–100 (2002)
Blake, C.L., Merz, C.J.: UCI Repository of Machine Learning Databases (1998), http://www.ics.uci.edu/~mlearn/MLRepository.html
Author information
Authors and Affiliations
Editor information
Editors and Affiliations
Rights and permissions
Copyright information
© 2005 Springer-Verlag Berlin Heidelberg
About this paper
Cite this paper
Shang, L., Wan, Q., Zhao, ZH., Chen, SF. (2005). Evolutionary Computation and Rough Set-Based Hybrid Approach to Rule Generation. In: Wang, L., Chen, K., Ong, Y.S. (eds) Advances in Natural Computation. ICNC 2005. Lecture Notes in Computer Science, vol 3612. Springer, Berlin, Heidelberg. https://doi.org/10.1007/11539902_105
Download citation
DOI: https://doi.org/10.1007/11539902_105
Publisher Name: Springer, Berlin, Heidelberg
Print ISBN: 978-3-540-28320-1
Online ISBN: 978-3-540-31863-7
eBook Packages: Computer ScienceComputer Science (R0)