Benchmarking large language models' performances for myopia care: a comparative analysis of ChatGPT-3.5, ChatGPT-4.0, and Google Bard
- PMID: 37625267
- PMCID: PMC10470220
- DOI: 10.1016/j.ebiom.2023.104770
Benchmarking large language models' performances for myopia care: a comparative analysis of ChatGPT-3.5, ChatGPT-4.0, and Google Bard
Abstract
Background: Large language models (LLMs) are garnering wide interest due to their human-like and contextually relevant responses. However, LLMs' accuracy across specific medical domains has yet been thoroughly evaluated. Myopia is a frequent topic which patients and parents commonly seek information online. Our study evaluated the performance of three LLMs namely ChatGPT-3.5, ChatGPT-4.0, and Google Bard, in delivering accurate responses to common myopia-related queries.
Methods: We curated thirty-one commonly asked myopia care-related questions, which were categorised into six domains-pathogenesis, risk factors, clinical presentation, diagnosis, treatment and prevention, and prognosis. Each question was posed to the LLMs, and their responses were independently graded by three consultant-level paediatric ophthalmologists on a three-point accuracy scale (poor, borderline, good). A majority consensus approach was used to determine the final rating for each response. 'Good' rated responses were further evaluated for comprehensiveness on a five-point scale. Conversely, 'poor' rated responses were further prompted for self-correction and then re-evaluated for accuracy.
Findings: ChatGPT-4.0 demonstrated superior accuracy, with 80.6% of responses rated as 'good', compared to 61.3% in ChatGPT-3.5 and 54.8% in Google Bard (Pearson's chi-squared test, all p ≤ 0.009). All three LLM-Chatbots showed high mean comprehensiveness scores (Google Bard: 4.35; ChatGPT-4.0: 4.23; ChatGPT-3.5: 4.11, out of a maximum score of 5). All LLM-Chatbots also demonstrated substantial self-correction capabilities: 66.7% (2 in 3) of ChatGPT-4.0's, 40% (2 in 5) of ChatGPT-3.5's, and 60% (3 in 5) of Google Bard's responses improved after self-correction. The LLM-Chatbots performed consistently across domains, except for 'treatment and prevention'. However, ChatGPT-4.0 still performed superiorly in this domain, receiving 70% 'good' ratings, compared to 40% in ChatGPT-3.5 and 45% in Google Bard (Pearson's chi-squared test, all p ≤ 0.001).
Interpretation: Our findings underscore the potential of LLMs, particularly ChatGPT-4.0, for delivering accurate and comprehensive responses to myopia-related queries. Continuous strategies and evaluations to improve LLMs' accuracy remain crucial.
Funding: Dr Yih-Chung Tham was supported by the National Medical Research Council of Singapore (NMRC/MOH/HCSAINV21nov-0001).
Keywords: ChatGPT-3.5; ChatGPT-4.0; Chatbot; Google Bard; Large language models; Myopia.
Copyright © 2023 The Author(s). Published by Elsevier B.V. All rights reserved.
Conflict of interest statement
Declaration of interests All authors declare no competing interests.
Figures
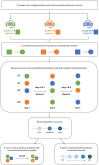
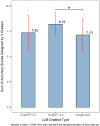
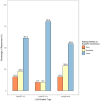
Similar articles
-
Popular large language model chatbots' accuracy, comprehensiveness, and self-awareness in answering ocular symptom queries.iScience. 2023 Oct 10;26(11):108163. doi: 10.1016/j.isci.2023.108163. eCollection 2023 Nov 17. iScience. 2023. PMID: 37915603 Free PMC article.
-
Large Language Models in Hematology Case Solving: A Comparative Study of ChatGPT-3.5, Google Bard, and Microsoft Bing.Cureus. 2023 Aug 21;15(8):e43861. doi: 10.7759/cureus.43861. eCollection 2023 Aug. Cureus. 2023. PMID: 37736448 Free PMC article.
-
Evaluation of the Performance of Generative AI Large Language Models ChatGPT, Google Bard, and Microsoft Bing Chat in Supporting Evidence-Based Dentistry: Comparative Mixed Methods Study.J Med Internet Res. 2023 Dec 28;25:e51580. doi: 10.2196/51580. J Med Internet Res. 2023. PMID: 38009003 Free PMC article.
-
ChatGPT and large language model (LLM) chatbots: The current state of acceptability and a proposal for guidelines on utilization in academic medicine.J Pediatr Urol. 2023 Oct;19(5):598-604. doi: 10.1016/j.jpurol.2023.05.018. Epub 2023 Jun 2. J Pediatr Urol. 2023. PMID: 37328321 Review.
-
Utility of artificial intelligence-based large language models in ophthalmic care.Ophthalmic Physiol Opt. 2024 May;44(3):641-671. doi: 10.1111/opo.13284. Epub 2024 Feb 25. Ophthalmic Physiol Opt. 2024. PMID: 38404172 Review.
Cited by
-
Prompt engineering in consistency and reliability with the evidence-based guideline for LLMs.NPJ Digit Med. 2024 Feb 20;7(1):41. doi: 10.1038/s41746-024-01029-4. NPJ Digit Med. 2024. PMID: 38378899 Free PMC article.
-
Adapted large language models can outperform medical experts in clinical text summarization.Nat Med. 2024 Apr;30(4):1134-1142. doi: 10.1038/s41591-024-02855-5. Epub 2024 Feb 27. Nat Med. 2024. PMID: 38413730 Free PMC article.
-
Educational Limitations of ChatGPT in Neurosurgery Board Preparation.Cureus. 2024 Apr 20;16(4):e58639. doi: 10.7759/cureus.58639. eCollection 2024 Apr. Cureus. 2024. PMID: 38770467 Free PMC article.
-
Embracing the future: Integrating ChatGPT into China's nursing education system.Int J Nurs Sci. 2024 Mar 7;11(2):295-299. doi: 10.1016/j.ijnss.2024.03.006. eCollection 2024 Apr. Int J Nurs Sci. 2024. PMID: 38707690 Free PMC article.
-
AI in Hand Surgery: Assessing Large Language Models in the Classification and Management of Hand Injuries.J Clin Med. 2024 May 11;13(10):2832. doi: 10.3390/jcm13102832. J Clin Med. 2024. PMID: 38792374 Free PMC article.
References
-
- Moor M., Banerjee O., Abad Z.S.H., et al. Foundation models for generalist medical artificial intelligence. Nature. 2023;616(7956):259–265. - PubMed
-
- Haupt C.E., Marks M. AI-generated medical advice—GPT and beyond. JAMA. 2023;329(16):1349–1350. - PubMed
-
- Lee P., Bubeck S., Petro J. Benefits, limits, and risks of GPT-4 as an AI chatbot for medicine. N Engl J Med. 2023;388(13):1233–1239. - PubMed
MeSH terms
LinkOut - more resources
Full Text Sources