Enhancement of Non-Linear Deep Learning Model by Adjusting Confounding Variables for Bone Age Estimation in Pediatric Hand X-rays
- PMID: 37268839
- PMCID: PMC10501988
- DOI: 10.1007/s10278-023-00849-2
Enhancement of Non-Linear Deep Learning Model by Adjusting Confounding Variables for Bone Age Estimation in Pediatric Hand X-rays
Abstract
In medicine, confounding variables in a generalized linear model are often adjusted; however, these variables have not yet been exploited in a non-linear deep learning model. Sex plays important role in bone age estimation, and non-linear deep learning model reported their performances comparable to human experts. Therefore, we investigate the properties of using confounding variables in a non-linear deep learning model for bone age estimation in pediatric hand X-rays. The RSNA Pediatric Bone Age Challenge (2017) dataset is used to train deep learning models. The RSNA test dataset is used for internal validation, and 227 pediatric hand X-ray images with bone age, chronological age, and sex information from Asan Medical Center (AMC) for external validation. U-Net based autoencoder, U-Net multi-task learning (MTL), and auxiliary-accelerated MTL (AA-MTL) models are chosen. Bone age estimations adjusted by input, output prediction, and without adjusting the confounding variables are compared. Additionally, ablation studies for model size, auxiliary task hierarchy, and multiple tasks are conducted. Correlation and Bland-Altman plots between ground truth and model-predicted bone ages are evaluated. Averaged saliency maps based on image registration are superimposed on representative images according to puberty stage. In the RSNA test dataset, adjusting by input shows the best performances regardless of model size, with mean average errors (MAEs) of 5.740, 5.478, and 5.434 months for the U-Net backbone, U-Net MTL, and AA-MTL models, respectively. However, in the AMC dataset, the AA-MTL model that adjusts the confounding variable by prediction shows the best performance with an MAE of 8.190 months, whereas the other models show the best performances by adjusting the confounding variables by input. Ablation studies of task hierarchy reveal no significant differences in the results of the RSNA dataset. However, predicting the confounding variable in the second encoder layer and estimating bone age in the bottleneck layer shows the best performance in the AMC dataset. Ablations studies of multiple tasks reveal that leveraging confounding variables plays an important role regardless of multiple tasks. To estimate bone age in pediatric X-rays, the clinical setting and balance between model size, task hierarchy, and confounding adjustment method play important roles in performance and generalizability; therefore, proper adjusting methods of confounding variables to train deep learning-based models are required for improved models.
Keywords: Bone age estimation; Confounding variable; Deep learning; Model enhancement; Multi-task learning, Pediatric X-ray.
© 2023. The Author(s) under exclusive licence to Society for Imaging Informatics in Medicine.
Conflict of interest statement
The authors report no conflicts of interest.
Figures
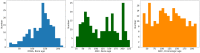
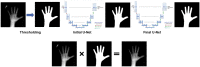
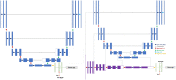
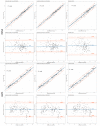
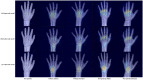
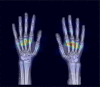
Similar articles
-
Generalizability and Bias in a Deep Learning Pediatric Bone Age Prediction Model Using Hand Radiographs.Radiology. 2023 Feb;306(2):e220505. doi: 10.1148/radiol.220505. Epub 2022 Sep 27. Radiology. 2023. PMID: 36165796
-
Evaluating the Robustness of a Deep Learning Bone Age Algorithm to Clinical Image Variation Using Computational Stress Testing.Radiol Artif Intell. 2024 May;6(3):e230240. doi: 10.1148/ryai.230240. Radiol Artif Intell. 2024. PMID: 38477660 Free PMC article.
-
MABAL: a Novel Deep-Learning Architecture for Machine-Assisted Bone Age Labeling.J Digit Imaging. 2018 Aug;31(4):513-519. doi: 10.1007/s10278-018-0053-3. J Digit Imaging. 2018. PMID: 29404850 Free PMC article.
-
Deep Learning Framework for Automatic Bone Age Assessment.Annu Int Conf IEEE Eng Med Biol Soc. 2021 Nov;2021:3093-3096. doi: 10.1109/EMBC46164.2021.9629650. Annu Int Conf IEEE Eng Med Biol Soc. 2021. PMID: 34891896
-
Deep learning-based automated bone age estimation for Saudi patients on hand radiograph images: a retrospective study.BMC Med Imaging. 2024 Aug 1;24(1):199. doi: 10.1186/s12880-024-01378-2. BMC Med Imaging. 2024. PMID: 39090563 Free PMC article.
References
-
- Goodfellow, I., Y. Bengio, and A. Courville, Deep learning. 2016: MIT press.
-
- Szegedy, C., et al. Going deeper with convolutions. in Proceedings of the IEEE conference on computer vision and pattern recognition. 2015.
-
- He, K., et al. Deep residual learning for image recognition. in Proceedings of the IEEE conference on computer vision and pattern recognition. 2016.
Publication types
MeSH terms
LinkOut - more resources
Full Text Sources