Deep learning-based image analysis of eyelid morphology in thyroid-associated ophthalmopathy
- PMID: 36915314
- PMCID: PMC10006102
- DOI: 10.21037/qims-22-551
Deep learning-based image analysis of eyelid morphology in thyroid-associated ophthalmopathy
Abstract
Background: We aimed to propose a deep learning-based approach to automatically measure eyelid morphology in patients with thyroid-associated ophthalmopathy (TAO).
Methods: This prospective study consecutively included 74 eyes of patients with TAO and 74 eyes of healthy volunteers visiting the ophthalmology department in a tertiary hospital. Patients diagnosed as TAO and healthy volunteers who were age- and gender-matched met the eligibility criteria for recruitment. Facial images were taken under the same light conditions. Comprehensive eyelid morphological parameters, such as palpebral fissure (PF) length, margin reflex distance (MRD), eyelid retraction distance, eyelid length, scleral area, and mid-pupil lid distance (MPLD), were automatically calculated using our deep learning-based analysis system. MRD1 and 2 were manually measured. Bland-Altman plots and intraclass correlation coefficients (ICCs) were performed to assess the agreement between automatic and manual measurements of MRDs. The asymmetry of the eyelid contour was analyzed using the temporal: nasal ratio of the MPLD. All eyelid features were compared between TAO eyes and control eyes using the independent samples t-test.
Results: A strong agreement between automatic and manual measurement was indicated. Biases of MRDs in TAO eyes and control eyes ranged from -0.01 mm [95% limits of agreement (LoA): -0.64 to 0.63 mm] to 0.09 mm (LoA: -0.46 to 0.63 mm). ICCs ranged from 0.932 to 0.980 (P<0.001). Eyelid features were significantly different in TAO eyes and control eyes, including MRD1 (4.82±1.59 vs. 2.99±0.81 mm; P<0.001), MRD2 (5.89±1.16 vs. 5.47±0.73 mm; P=0.009), upper eyelid length (UEL) (27.73±4.49 vs. 25.42±4.35 mm; P=0.002), lower eyelid length (LEL) (31.51±4.59 vs. 26.34±4.72 mm; P<0.001), and total scleral area (SATOTAL) (96.14±34.38 vs. 56.91±14.97 mm2; P<0.001). The MPLDs at all angles showed significant differences in the 2 groups of eyes (P=0.008 at temporal 180°; P<0.001 at other angles). The greatest temporal-nasal asymmetry appeared at 75° apart from the midline in TAO eyes.
Conclusions: Our proposed system allowed automatic, comprehensive, and objective measurement of eyelid morphology by only using facial images, which has potential application prospects in TAO. Future work with a large sample of patients that contains different TAO subsets is warranted.
Keywords: Thyroid-associated ophthalmopathy (TAO); automatic measurement; deep learning; eyelid morphology; facial images.
2023 Quantitative Imaging in Medicine and Surgery. All rights reserved.
Conflict of interest statement
Conflicts of Interest: All authors have completed the ICMJE uniform disclosure form (available at https://qims.amegroups.com/article/view/10.21037/qims-22-551/coif). The authors have no conflicts of interest to declare.
Figures
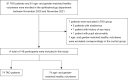
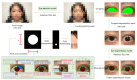
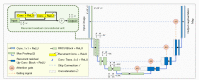
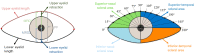
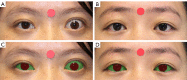
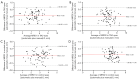
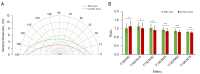
Similar articles
-
Automatic Measurement and Comparison of Normal Eyelid Contour by Age and Gender Using Image-Based Deep Learning.Ophthalmol Sci. 2024 Mar 21;4(5):100518. doi: 10.1016/j.xops.2024.100518. eCollection 2024 Sep-Oct. Ophthalmol Sci. 2024. PMID: 38881605 Free PMC article.
-
Deep learning-based image analysis for automated measurement of eyelid morphology before and after blepharoptosis surgery.Ann Med. 2021 Dec;53(1):2278-2285. doi: 10.1080/07853890.2021.2009127. Ann Med. 2021. PMID: 34844503 Free PMC article.
-
A Novel Automatic Morphologic Analysis of Eyelids Based on Deep Learning Methods.Curr Eye Res. 2021 Oct;46(10):1495-1502. doi: 10.1080/02713683.2021.1908569. Epub 2021 Jun 14. Curr Eye Res. 2021. PMID: 33827341
-
Triamcinolone injection in the treatment of lid retraction for thyroid-associated ophthalmopathy: A systematic review.Eur J Ophthalmol. 2025 Jan;35(1):69-76. doi: 10.1177/11206721241254405. Epub 2024 May 15. Eur J Ophthalmol. 2025. PMID: 38751133 Review.
-
Orbital and eyelid diseases: The next breakthrough in artificial intelligence?Front Cell Dev Biol. 2022 Nov 18;10:1069248. doi: 10.3389/fcell.2022.1069248. eCollection 2022. Front Cell Dev Biol. 2022. PMID: 36467418 Free PMC article. Review.
Cited by
-
Development of a neural network-based automated eyelid measurement system.Sci Rep. 2024 Jan 12;14(1):1202. doi: 10.1038/s41598-024-51838-6. Sci Rep. 2024. PMID: 38216653 Free PMC article.
-
Automatic Measurement and Comparison of Normal Eyelid Contour by Age and Gender Using Image-Based Deep Learning.Ophthalmol Sci. 2024 Mar 21;4(5):100518. doi: 10.1016/j.xops.2024.100518. eCollection 2024 Sep-Oct. Ophthalmol Sci. 2024. PMID: 38881605 Free PMC article.
-
Application of artificial intelligence in the assessment of thyroid eye disease (TED) - a scoping review.Front Endocrinol (Lausanne). 2023 Dec 20;14:1300196. doi: 10.3389/fendo.2023.1300196. eCollection 2023. Front Endocrinol (Lausanne). 2023. PMID: 38174334 Free PMC article.
-
Effective encoder-decoder network for pupil light reflex segmentation in facial photographs of ptosis patients.Sci Rep. 2024 Oct 31;14(1):26220. doi: 10.1038/s41598-024-77001-9. Sci Rep. 2024. PMID: 39482369 Free PMC article.
-
Multidimensional quantitative characterization of periocular morphology: distinguishing esotropia from epicanthus by deep learning network.Quant Imaging Med Surg. 2024 Sep 1;14(9):6273-6284. doi: 10.21037/qims-24-155. Epub 2024 Jul 29. Quant Imaging Med Surg. 2024. PMID: 39281168 Free PMC article.
References
-
- Manji N, Carr-Smith JD, Boelaert K, Allahabadia A, Armitage M, Chatterjee VK, Lazarus JH, Pearce SH, Vaidya B, Gough SC, Franklyn JA. Influences of age, gender, smoking, and family history on autoimmune thyroid disease phenotype. J Clin Endocrinol Metab 2006;91:4873-80. 10.1210/jc.2006-1402 - DOI - PubMed
LinkOut - more resources
Full Text Sources
Miscellaneous