End-to-End Deep-Learning-Based Diagnosis of Benign and Malignant Orbital Tumors on Computed Tomography Images
- PMID: 36836437
- PMCID: PMC9960119
- DOI: 10.3390/jpm13020204
End-to-End Deep-Learning-Based Diagnosis of Benign and Malignant Orbital Tumors on Computed Tomography Images
Abstract
Determining the nature of orbital tumors is challenging for current imaging interpretation methods, which hinders timely treatment. This study aimed to propose an end-to-end deep learning system to automatically diagnose orbital tumors. A multi-center dataset of 602 non-contrast-enhanced computed tomography (CT) images were prepared. After image annotation and preprocessing, the CT images were used to train and test the deep learning (DL) model for the following two stages: orbital tumor segmentation and classification. The performance on the testing set was compared with the assessment of three ophthalmologists. For tumor segmentation, the model achieved a satisfactory performance, with an average dice similarity coefficient of 0.89. The classification model had an accuracy of 86.96%, a sensitivity of 80.00%, and a specificity of 94.12%. The area under the receiver operating characteristics curve (AUC) of the 10-fold cross-validation ranged from 0.8439 to 0.9546. There was no significant difference on diagnostic performance of the DL-based system and three ophthalmologists (p > 0.05). The proposed end-to-end deep learning system could deliver accurate segmentation and diagnosis of orbital tumors based on noninvasive CT images. Its effectiveness and independence from human interaction allow the potential for tumor screening in the orbit and other parts of the body.
Keywords: classification; deep learning; hemangioma; lymphoma; segmentation.
Conflict of interest statement
The authors declare no conflict of interest.
Figures
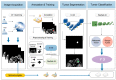
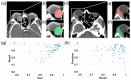
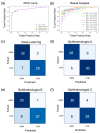
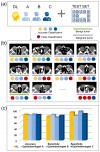
Similar articles
-
Development of a deep learning model for early gastric cancer diagnosis using preoperative computed tomography images.Front Oncol. 2023 Oct 6;13:1265366. doi: 10.3389/fonc.2023.1265366. eCollection 2023. Front Oncol. 2023. PMID: 37869090 Free PMC article.
-
Fully automated deep learning system for osteoporosis screening using chest computed tomography images.Quant Imaging Med Surg. 2024 Apr 3;14(4):2816-2827. doi: 10.21037/qims-23-1617. Epub 2024 Mar 21. Quant Imaging Med Surg. 2024. PMID: 38617137 Free PMC article.
-
Automatic coronary artery segmentation and diagnosis of stenosis by deep learning based on computed tomographic coronary angiography.Eur Radiol. 2022 Sep;32(9):6037-6045. doi: 10.1007/s00330-022-08761-z. Epub 2022 Apr 8. Eur Radiol. 2022. PMID: 35394183
-
Deep Learning Computer-Aided Diagnosis for Breast Lesion in Digital Mammogram.Adv Exp Med Biol. 2020;1213:59-72. doi: 10.1007/978-3-030-33128-3_4. Adv Exp Med Biol. 2020. PMID: 32030663 Review.
-
Deep Learning Approaches Towards Skin Lesion Segmentation and Classification from Dermoscopic Images - A Review.Curr Med Imaging. 2020;16(5):513-533. doi: 10.2174/1573405615666190129120449. Curr Med Imaging. 2020. PMID: 32484086 Review.
Cited by
-
Using Deep Learning to Distinguish Highly Malignant Uveal Melanoma from Benign Choroidal Nevi.J Clin Med. 2024 Jul 16;13(14):4141. doi: 10.3390/jcm13144141. J Clin Med. 2024. PMID: 39064181 Free PMC article.
References
-
- Rootman D.B., Heran M.K., Rootman J., White V.A., Luemsamran P., Yucel Y.H. Cavernous venous malformations of the orbit (so-called cavernous haemangioma): A comprehensive evaluation of their clinical, imaging and histologic nature. Br. J. Ophthalmol. 2014;98:880–888. doi: 10.1136/bjophthalmol-2013-304460. - DOI - PubMed
Grants and funding
- 2019YFC0118401/the National Key Research and Development Program of China
- U20A20386/National Natural Science Foundation Regional Innovation and Development Joint Fund
- 61975178/the National Natural Science Foundation of China
- 2019C03020/Zhejiang Provincial Key Research and Development Plan
- LR20F050002/Natural Science Foundation of Zhejiang Province
LinkOut - more resources
Full Text Sources