Improving Data-Efficiency and Robustness of Medical Imaging Segmentation Using Inpainting-Based Self-Supervised Learning
- PMID: 36829701
- PMCID: PMC9951871
- DOI: 10.3390/bioengineering10020207
Improving Data-Efficiency and Robustness of Medical Imaging Segmentation Using Inpainting-Based Self-Supervised Learning
Abstract
We systematically evaluate the training methodology and efficacy of two inpainting-based pretext tasks of context prediction and context restoration for medical image segmentation using self-supervised learning (SSL). Multiple versions of self-supervised U-Net models were trained to segment MRI and CT datasets, each using a different combination of design choices and pretext tasks to determine the effect of these design choices on segmentation performance. The optimal design choices were used to train SSL models that were then compared with baseline supervised models for computing clinically-relevant metrics in label-limited scenarios. We observed that SSL pretraining with context restoration using 32 × 32 patches and Poission-disc sampling, transferring only the pretrained encoder weights, and fine-tuning immediately with an initial learning rate of 1 × 10-3 provided the most benefit over supervised learning for MRI and CT tissue segmentation accuracy (p < 0.001). For both datasets and most label-limited scenarios, scaling the size of unlabeled pretraining data resulted in improved segmentation performance. SSL models pretrained with this amount of data outperformed baseline supervised models in the computation of clinically-relevant metrics, especially when the performance of supervised learning was low. Our results demonstrate that SSL pretraining using inpainting-based pretext tasks can help increase the robustness of models in label-limited scenarios and reduce worst-case errors that occur with supervised learning.
Keywords: CT; MRI; deep learning; machine learning; segmentation; self-supervised learning.
Conflict of interest statement
The funders had no role in the design of the study; in the collection, analyses, or interpretation of data; in the writing of the manuscript; or in the decision to publish the results.
Figures
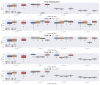
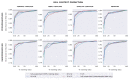
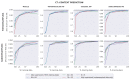
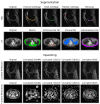
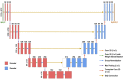
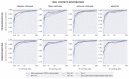
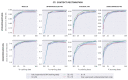
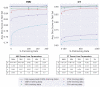
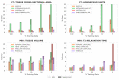
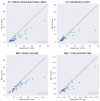
Similar articles
-
Self-supervised-RCNN for medical image segmentation with limited data annotation.Comput Med Imaging Graph. 2023 Oct;109:102297. doi: 10.1016/j.compmedimag.2023.102297. Epub 2023 Sep 9. Comput Med Imaging Graph. 2023. PMID: 37729826
-
Volumetric white matter tract segmentation with nested self-supervised learning using sequential pretext tasks.Med Image Anal. 2021 Aug;72:102094. doi: 10.1016/j.media.2021.102094. Epub 2021 Apr 30. Med Image Anal. 2021. PMID: 34004493
-
Enhancing diagnostic deep learning via self-supervised pretraining on large-scale, unlabeled non-medical images.Eur Radiol Exp. 2024 Feb 8;8(1):10. doi: 10.1186/s41747-023-00411-3. Eur Radiol Exp. 2024. PMID: 38326501 Free PMC article.
-
Survey on Self-Supervised Learning: Auxiliary Pretext Tasks and Contrastive Learning Methods in Imaging.Entropy (Basel). 2022 Apr 14;24(4):551. doi: 10.3390/e24040551. Entropy (Basel). 2022. PMID: 35455214 Free PMC article. Review.
-
Self-supervised learning framework application for medical image analysis: a review and summary.Biomed Eng Online. 2024 Oct 27;23(1):107. doi: 10.1186/s12938-024-01299-9. Biomed Eng Online. 2024. PMID: 39465395 Free PMC article. Review.
Cited by
-
Self-Supervised Learning Improves Accuracy and Data Efficiency for IMU-Based Ground Reaction Force Estimation.bioRxiv [Preprint]. 2024 Jan 25:2023.10.25.564057. doi: 10.1101/2023.10.25.564057. bioRxiv. 2024. Update in: IEEE Trans Biomed Eng. 2024 Jul;71(7):2095-2104. doi: 10.1109/TBME.2024.3361888 PMID: 38328126 Free PMC article. Updated. Preprint.
-
AI in MRI: Computational Frameworks for a Faster, Optimized, and Automated Imaging Workflow.Bioengineering (Basel). 2023 Apr 20;10(4):492. doi: 10.3390/bioengineering10040492. Bioengineering (Basel). 2023. PMID: 37106679 Free PMC article.
-
Self-Supervised Learning Improves Accuracy and Data Efficiency for IMU-Based Ground Reaction Force Estimation.IEEE Trans Biomed Eng. 2024 Jul;71(7):2095-2104. doi: 10.1109/TBME.2024.3361888. Epub 2024 Jun 19. IEEE Trans Biomed Eng. 2024. PMID: 38315597
-
A vision-language foundation model for the generation of realistic chest X-ray images.Nat Biomed Eng. 2024 Aug 26. doi: 10.1038/s41551-024-01246-y. Online ahead of print. Nat Biomed Eng. 2024. PMID: 39187663
References
-
- Campello V.M., Gkontra P., Izquierdo C., Martín-Isla C., Sojoudi A., Full P.M., Maier-Hein K., Zhang Y., He Z., Ma J., et al. Multi-Centre, Multi-Vendor and Multi-Disease Cardiac Segmentation: The M&Ms Challenge. IEEE Trans. Med. Imaging. 2021;40:3543–3554. - PubMed
-
- Desai A.D., Caliva F., Iriondo C., Mortazi A., Jambawalikar S., Bagci U., Perslev M., Igel C., Dam E.B., Gaj S., et al. The international workshop on osteoarthritis imaging knee MRI segmentation challenge: A multi-institute evaluation and analysis framework on a standardized dataset. Radiol. Artif. Intell. 2021;3:e200078. doi: 10.1148/ryai.2021200078. - DOI - PMC - PubMed
-
- Desai A.D., Gold G.E., Hargreaves B.A., Chaudhari A.S. Technical considerations for semantic segmentation in MRI using convolutional neural networks. arXiv. 20191902.01977
Grants and funding
- R01 EB002524/EB/NIBIB NIH HHS/United States
- DGE 1656518/National Science Foundation
- P41 EB027060/NH/NIH HHS/United States
- 75N92020C00021/HL/NHLBI NIH HHS/United States
- R01 AR079431/AR/NIAMS NIH HHS/United States
- NA/GE Healthcare
- CCF1563078/National Science Foundation
- R01 AR079431/NH/NIH HHS/United States
- NA/Philips (United States)
- 75N92020C00021/NH/NIH HHS/United States
- National Science and Engineering Graduate Fellowship (ARO)/United States Department of Defense
- 75N92020C00008/HL/NHLBI NIH HHS/United States
- CCF1763315/National Science Foundation
- Precision Health and Integrated Diagnostics Seed Grant/Stanford University
- R01 EB002524/NH/NIH HHS/United States
- R01 AR077604/AR/NIAMS NIH HHS/United States
- K24 AR062068/AR/NIAMS NIH HHS/United States
- P41 EB027060/EB/NIBIB NIH HHS/United States
- R01 AR077604/NH/NIH HHS/United States
- 75N92020C00008/NH/NIH HHS/United States
- K24 AR062068/NH/NIH HHS/United States
LinkOut - more resources
Full Text Sources