Performance of ChatGPT on USMLE: Potential for AI-assisted medical education using large language models
- PMID: 36812645
- PMCID: PMC9931230
- DOI: 10.1371/journal.pdig.0000198
Performance of ChatGPT on USMLE: Potential for AI-assisted medical education using large language models
Abstract
We evaluated the performance of a large language model called ChatGPT on the United States Medical Licensing Exam (USMLE), which consists of three exams: Step 1, Step 2CK, and Step 3. ChatGPT performed at or near the passing threshold for all three exams without any specialized training or reinforcement. Additionally, ChatGPT demonstrated a high level of concordance and insight in its explanations. These results suggest that large language models may have the potential to assist with medical education, and potentially, clinical decision-making.
Copyright: © 2023 Kung et al. This is an open access article distributed under the terms of the Creative Commons Attribution License, which permits unrestricted use, distribution, and reproduction in any medium, provided the original author and source are credited.
Conflict of interest statement
The authors have declared that no competing interests exist.
Figures
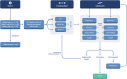
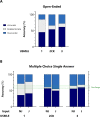
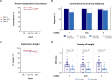
Similar articles
-
ChatGPT-4: An assessment of an upgraded artificial intelligence chatbot in the United States Medical Licensing Examination.Med Teach. 2024 Mar;46(3):366-372. doi: 10.1080/0142159X.2023.2249588. Epub 2023 Oct 15. Med Teach. 2024. PMID: 37839017
-
How Does ChatGPT Perform on the United States Medical Licensing Examination (USMLE)? The Implications of Large Language Models for Medical Education and Knowledge Assessment.JMIR Med Educ. 2023 Feb 8;9:e45312. doi: 10.2196/45312. JMIR Med Educ. 2023. PMID: 36753318 Free PMC article.
-
Pure Wisdom or Potemkin Villages? A Comparison of ChatGPT 3.5 and ChatGPT 4 on USMLE Step 3 Style Questions: Quantitative Analysis.JMIR Med Educ. 2024 Jan 5;10:e51148. doi: 10.2196/51148. JMIR Med Educ. 2024. PMID: 38180782 Free PMC article.
-
Performance of ChatGPT Across Different Versions in Medical Licensing Examinations Worldwide: Systematic Review and Meta-Analysis.J Med Internet Res. 2024 Jul 25;26:e60807. doi: 10.2196/60807. J Med Internet Res. 2024. PMID: 39052324 Free PMC article. Review.
-
Application of artificial intelligence chatbots, including ChatGPT, in education, scholarly work, programming, and content generation and its prospects: a narrative review.J Educ Eval Health Prof. 2023;20:38. doi: 10.3352/jeehp.2023.20.38. Epub 2023 Dec 27. J Educ Eval Health Prof. 2023. PMID: 38148495 Review.
Cited by
-
Evaluating the Capabilities of Generative AI Tools in Understanding Medical Papers: Qualitative Study.JMIR Med Inform. 2024 Sep 4;12:e59258. doi: 10.2196/59258. JMIR Med Inform. 2024. PMID: 39230947 Free PMC article.
-
Heart-to-heart with ChatGPT: the impact of patients consulting AI for cardiovascular health advice.Open Heart. 2023 Nov;10(2):e002455. doi: 10.1136/openhrt-2023-002455. Open Heart. 2023. PMID: 37945282 Free PMC article. Review.
-
Based on Medicine, The Now and Future of Large Language Models.Cell Mol Bioeng. 2024 Sep 16;17(4):263-277. doi: 10.1007/s12195-024-00820-3. eCollection 2024 Aug. Cell Mol Bioeng. 2024. PMID: 39372551 Review.
-
The Role of ChatGPT in the Advancement of Diagnosis, Management, and Prognosis of Cardiovascular and Cerebrovascular Disease.Healthcare (Basel). 2023 Nov 6;11(21):2906. doi: 10.3390/healthcare11212906. Healthcare (Basel). 2023. PMID: 37958050 Free PMC article. Review.
-
Advancement of Generative Pre-trained Transformer Chatbots in Answering Clinical Questions in the Practical Rhinoplasty Guideline.Aesthetic Plast Surg. 2024 Sep 25. doi: 10.1007/s00266-024-04377-4. Online ahead of print. Aesthetic Plast Surg. 2024. PMID: 39322837
References
-
- Szegedy C, Vanhoucke V, Ioffe S, Shlens J, Wojna Z. Rethinking the inception architecture for computer vision. 2016 IEEE Conference on Computer Vision and Pattern Recognition (CVPR). IEEE; 2016. doi: 10.1109/cvpr.2016.308 - DOI
-
- Zhang W, Feng Y, Meng F, You D, Liu Q. Bridging the gap between training and inference for neural machine translation. Proceedings of the 57th Annual Meeting of the Association for Computational Linguistics. Stroudsburg, PA, USA: Association for Computational Linguistics; 2019. doi: 10.18653/v1/p19-1426 - DOI
-
- Bhatia Y, Bajpayee A, Raghuvanshi D, Mittal H. Image captioning using Google’s inception-resnet-v2 and recurrent neural network. 2019 Twelfth International Conference on Contemporary Computing (IC3). IEEE; 2019. doi: 10.1109/ic3.2019.8844921 - DOI
Grants and funding
LinkOut - more resources
Full Text Sources