How Does ChatGPT Perform on the United States Medical Licensing Examination (USMLE)? The Implications of Large Language Models for Medical Education and Knowledge Assessment
- PMID: 36753318
- PMCID: PMC9947764
- DOI: 10.2196/45312
How Does ChatGPT Perform on the United States Medical Licensing Examination (USMLE)? The Implications of Large Language Models for Medical Education and Knowledge Assessment
Erratum in
-
Correction: How Does ChatGPT Perform on the United States Medical Licensing Examination (USMLE)? The Implications of Large Language Models for Medical Education and Knowledge Assessment.JMIR Med Educ. 2024 Feb 27;10:e57594. doi: 10.2196/57594. JMIR Med Educ. 2024. PMID: 38412478 Free PMC article.
Abstract
Background: Chat Generative Pre-trained Transformer (ChatGPT) is a 175-billion-parameter natural language processing model that can generate conversation-style responses to user input.
Objective: This study aimed to evaluate the performance of ChatGPT on questions within the scope of the United States Medical Licensing Examination (USMLE) Step 1 and Step 2 exams, as well as to analyze responses for user interpretability.
Methods: We used 2 sets of multiple-choice questions to evaluate ChatGPT's performance, each with questions pertaining to Step 1 and Step 2. The first set was derived from AMBOSS, a commonly used question bank for medical students, which also provides statistics on question difficulty and the performance on an exam relative to the user base. The second set was the National Board of Medical Examiners (NBME) free 120 questions. ChatGPT's performance was compared to 2 other large language models, GPT-3 and InstructGPT. The text output of each ChatGPT response was evaluated across 3 qualitative metrics: logical justification of the answer selected, presence of information internal to the question, and presence of information external to the question.
Results: Of the 4 data sets, AMBOSS-Step1, AMBOSS-Step2, NBME-Free-Step1, and NBME-Free-Step2, ChatGPT achieved accuracies of 44% (44/100), 42% (42/100), 64.4% (56/87), and 57.8% (59/102), respectively. ChatGPT outperformed InstructGPT by 8.15% on average across all data sets, and GPT-3 performed similarly to random chance. The model demonstrated a significant decrease in performance as question difficulty increased (P=.01) within the AMBOSS-Step1 data set. We found that logical justification for ChatGPT's answer selection was present in 100% of outputs of the NBME data sets. Internal information to the question was present in 96.8% (183/189) of all questions. The presence of information external to the question was 44.5% and 27% lower for incorrect answers relative to correct answers on the NBME-Free-Step1 (P<.001) and NBME-Free-Step2 (P=.001) data sets, respectively.
Conclusions: ChatGPT marks a significant improvement in natural language processing models on the tasks of medical question answering. By performing at a greater than 60% threshold on the NBME-Free-Step-1 data set, we show that the model achieves the equivalent of a passing score for a third-year medical student. Additionally, we highlight ChatGPT's capacity to provide logic and informational context across the majority of answers. These facts taken together make a compelling case for the potential applications of ChatGPT as an interactive medical education tool to support learning.
Keywords: ChatGPT; GPT; MedQA; NLP; artificial intelligence; chatbot; conversational agent; education technology; generative pre-trained transformer; machine learning; medical education; natural language processing; USMLE.
©Aidan Gilson, Conrad W Safranek, Thomas Huang, Vimig Socrates, Ling Chi, Richard Andrew Taylor, David Chartash. Originally published in JMIR Medical Education (https://mededu.jmir.org), 08.02.2023.
Conflict of interest statement
Conflicts of Interest: None declared.
Figures
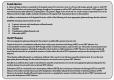
Comment in
-
Can ChatGPT be a new educational tool in medicine?Med Clin (Barc). 2023 Oct 27;161(8):363-364. doi: 10.1016/j.medcli.2023.05.018. Epub 2023 Jul 10. Med Clin (Barc). 2023. PMID: 37438191 English, Spanish. No abstract available.
Similar articles
-
Performance of ChatGPT on the Peruvian National Licensing Medical Examination: Cross-Sectional Study.JMIR Med Educ. 2023 Sep 28;9:e48039. doi: 10.2196/48039. JMIR Med Educ. 2023. PMID: 37768724 Free PMC article.
-
ChatGPT's performance in German OB/GYN exams - paving the way for AI-enhanced medical education and clinical practice.Front Med (Lausanne). 2023 Dec 13;10:1296615. doi: 10.3389/fmed.2023.1296615. eCollection 2023. Front Med (Lausanne). 2023. PMID: 38155661 Free PMC article.
-
Pure Wisdom or Potemkin Villages? A Comparison of ChatGPT 3.5 and ChatGPT 4 on USMLE Step 3 Style Questions: Quantitative Analysis.JMIR Med Educ. 2024 Jan 5;10:e51148. doi: 10.2196/51148. JMIR Med Educ. 2024. PMID: 38180782 Free PMC article.
-
Performance of ChatGPT Across Different Versions in Medical Licensing Examinations Worldwide: Systematic Review and Meta-Analysis.J Med Internet Res. 2024 Jul 25;26:e60807. doi: 10.2196/60807. J Med Internet Res. 2024. PMID: 39052324 Free PMC article. Review.
-
ChatGPT and neurosurgical education: A crossroads of innovation and opportunity.J Clin Neurosci. 2024 Nov;129:110815. doi: 10.1016/j.jocn.2024.110815. Epub 2024 Sep 4. J Clin Neurosci. 2024. PMID: 39236407
Cited by
-
Performance of Generative Pretrained Transformer on the National Medical Licensing Examination in Japan.PLOS Digit Health. 2024 Jan 23;3(1):e0000433. doi: 10.1371/journal.pdig.0000433. eCollection 2024 Jan. PLOS Digit Health. 2024. PMID: 38261580 Free PMC article.
-
Revolutionizing Dental Care: A Comprehensive Review of Artificial Intelligence Applications Among Various Dental Specialties.Cureus. 2023 Oct 14;15(10):e47033. doi: 10.7759/cureus.47033. eCollection 2023 Oct. Cureus. 2023. PMID: 37965397 Free PMC article. Review.
-
New evidence-based practice: Artificial intelligence as a barrier breaker.World J Methodol. 2023 Dec 20;13(5):384-389. doi: 10.5662/wjm.v13.i5.384. eCollection 2023 Dec 20. World J Methodol. 2023. PMID: 38229944 Free PMC article.
-
ChatGPT for scientific community: Boon or bane?Med J Armed Forces India. 2023 Sep-Oct;79(5):498-499. doi: 10.1016/j.mjafi.2023.06.009. Epub 2023 Aug 8. Med J Armed Forces India. 2023. PMID: 37719916 Free PMC article. No abstract available.
-
Assessing the Performance of ChatGPT in Medical Biochemistry Using Clinical Case Vignettes: Observational Study.JMIR Med Educ. 2023 Nov 7;9:e47191. doi: 10.2196/47191. JMIR Med Educ. 2023. PMID: 37934568 Free PMC article.
References
-
- OpenAI ChatGPT: optimizing language models for dialogue. OpenAI. 2022. Nov 30, [2022-12-22]. https://openai.com/blog/chatgpt/
-
- Scott K. Microsoft teams up with OpenAI to exclusively license GPT-3 language model. The Official Microsoft Blog. 2020. Sep 22, [2022-12-19]. https://blogs.microsoft.com/blog/2020/09/22/microsoft-teams-up-with-open...
-
- Bowman E. A new AI chatbot might do your homework for you. but it's still not an A+ student. NPR. 2022. Dec 19, [2022-12-19]. https://www.npr.org/2022/12/19/1143912956/chatgpt-ai-chatbot-homework-ac... .
-
- How good is ChatGPT? The Economist. 2022. Dec 8, [2022-12-20]. https://www.economist.com/business/2022/12/08/how-good-is-chatgpt .
-
- Chambers A Can Artificial Intelligence (Chat GPT) get a 7 on an SL Maths paper? IB Maths Resources from Intermathematics. 2022. Dec 11, [2022-12-20]. https://ibmathsresources.com/2022/12/11/can-artificial-intelligence-chat...
Grants and funding
LinkOut - more resources
Full Text Sources