Automated photographic analysis of inferior oblique overaction based on deep learning
- PMID: 36620142
- PMCID: PMC9816726
- DOI: 10.21037/qims-22-467
Automated photographic analysis of inferior oblique overaction based on deep learning
Abstract
Background: Inferior oblique overaction (IOOA) is a common ocular motility disorder. This study aimed to propose a novel deep learning-based approach to automatically evaluate the amount of IOOA.
Methods: This prospective study included 106 eyes of 72 consecutive patients attending the strabismus clinic in a tertiary referral hospital. Patients were eligible for inclusion if they were diagnosed with IOOA. IOOA was clinically graded from +1 to +4. Based on photograph in the adducted position, the height difference between the inferior corneal limbus of both eyes was manually measured using ImageJ and automatically measured by our deep learning-based image analysis system with human supervision. Correlation coefficients, Bland-Altman plots and mean absolute deviation (MAD) were analyzed between two different measurements of evaluating IOOA.
Results: There were significant correlations between automated photographic measurements and clinical gradings (Kendall's tau: 0.721; 95% confidence interval: 0.652 to 0.779; P<0.001), between automated and manual photographic measurements [intraclass correlation coefficients (ICCs): 0.975; 95% confidence interval: 0.963 to 0.983; P<0.001], and between two-repeated automated photographic measurements (ICCs: 0.998; 95% confidence interval: 0.997 to 0.999; P<0.001). The biases and MADs were 0.10 [95% limits of agreement (LoA): -0.45 to 0.64] mm and 0.26±0.14 mm between automated and manual photographic measurements, and 0.01 (95% LoA: -0.14 to 0.16) mm and 0.07±0.04 mm between two-repeated automated photographic measurements, respectively.
Conclusions: The automated photographic measurements of IOOA using deep learning technique were in excellent agreement with manual photographic measurements and clinical gradings. This new approach allows objective, accurate and repeatable measurement of IOOA and could be easily implemented in clinical practice using only photographs.
Keywords: Inferior oblique overaction (IOOA); automated image analysis; deep learning.
2023 Quantitative Imaging in Medicine and Surgery. All rights reserved.
Conflict of interest statement
Conflicts of Interest: All authors have completed the ICMJE uniform disclosure form (available at https://qims.amegroups.com/article/view/10.21037/qims-22-467/coif). The authors have no conflicts of interest to declare.
Figures
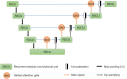
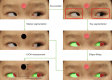
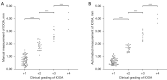
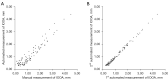
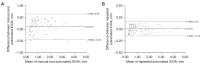
Similar articles
-
Automated Measurement of Ocular Movements Using Deep Learning-Based Image Analysis.Curr Eye Res. 2022 Sep;47(9):1346-1353. doi: 10.1080/02713683.2022.2053165. Epub 2022 Jul 27. Curr Eye Res. 2022. PMID: 35899319
-
Comparison of Different Surgical Approaches to Inferior Oblique Overaction.J Binocul Vis Ocul Motil. 2020 Jul-Sep;70(3):89-93. doi: 10.1080/2576117X.2020.1776566. Epub 2020 Jun 8. J Binocul Vis Ocul Motil. 2020. PMID: 32511077
-
Comparison of excyclotorsion following graded inferior oblique recession for primary versus secondary inferior oblique overaction.Int J Ophthalmol. 2020 Aug 18;13(8):1281-1286. doi: 10.18240/ijo.2020.08.15. eCollection 2020. Int J Ophthalmol. 2020. PMID: 32821683 Free PMC article.
-
Effect of bilateral inferior oblique partial myectomy on V pattern exotropia with inferior oblique overaction.BMC Ophthalmol. 2022 May 21;22(1):230. doi: 10.1186/s12886-022-02456-1. BMC Ophthalmol. 2022. PMID: 35597903 Free PMC article.
-
Inferior Oblique Anterior Transposition versus Myectomy for Inferior Oblique Overaction: Systematic Review and Meta Analysis.Eur J Ophthalmol. 2022 Sep 21:11206721221127769. doi: 10.1177/11206721221127769. Online ahead of print. Eur J Ophthalmol. 2022. PMID: 36131380 Review.
Cited by
-
A deep learning-based image analysis for assessing the extent of abduction in abducens nerve palsy patients before and after strabismus surgery.Adv Ophthalmol Pract Res. 2024 Jun 25;4(4):202-208. doi: 10.1016/j.aopr.2024.06.004. eCollection 2024 Nov-Dec. Adv Ophthalmol Pract Res. 2024. PMID: 39484054 Free PMC article.
-
Advances in artificial intelligence models and algorithms in the field of optometry.Front Cell Dev Biol. 2023 Apr 28;11:1170068. doi: 10.3389/fcell.2023.1170068. eCollection 2023. Front Cell Dev Biol. 2023. PMID: 37187617 Free PMC article. Review.
-
Global research of artificial intelligence in strabismus: a bibliometric analysis.Front Med (Lausanne). 2023 Sep 20;10:1244007. doi: 10.3389/fmed.2023.1244007. eCollection 2023. Front Med (Lausanne). 2023. PMID: 37799591 Free PMC article.
References
-
- Caldeira JA. Some clinical characteristics of V-pattern exotropia and surgical outcome after bilateral recession of the inferior oblique muscle: a retrospective study of 22 consecutive patients and a comparison with V-pattern esotropia. Binocul Vis Strabismus Q 2004;19:139-50. - PubMed
-
- Bowling B. Kanski's clinical ophthalmology. Edinburgh: Elsevier, 2016.
-
- Mohamed AA, Yousef H, Mounir A. Surgical outcomes of inferior oblique muscle weakening procedures for eliminating inferior oblique muscle overaction: a prospective randomized study. Delta J Ophthalmol 2019;20:88-94.
LinkOut - more resources
Full Text Sources