Public attitudes value interpretability but prioritize accuracy in Artificial Intelligence
- PMID: 36192416
- PMCID: PMC9528860
- DOI: 10.1038/s41467-022-33417-3
Public attitudes value interpretability but prioritize accuracy in Artificial Intelligence
Abstract
As Artificial Intelligence (AI) proliferates across important social institutions, many of the most powerful AI systems available are difficult to interpret for end-users and engineers alike. Here, we sought to characterize public attitudes towards AI interpretability. Across seven studies (N = 2475), we demonstrate robust and positive attitudes towards interpretable AI among non-experts that generalize across a variety of real-world applications and follow predictable patterns. Participants value interpretability positively across different levels of AI autonomy and accuracy, and rate interpretability as more important for AI decisions involving high stakes and scarce resources. Crucially, when AI interpretability trades off against AI accuracy, participants prioritize accuracy over interpretability under the same conditions driving positive attitudes towards interpretability in the first place: amidst high stakes and scarce resources. These attitudes could drive a proliferation of AI systems making high-impact ethical decisions that are difficult to explain and understand.
© 2022. The Author(s).
Conflict of interest statement
The authors declare no competing interests.
Figures
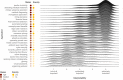
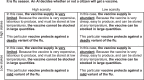
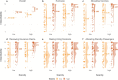
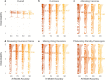
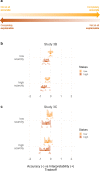
Similar articles
-
Public Perception of Artificial Intelligence in Medical Care: Content Analysis of Social Media.J Med Internet Res. 2020 Jul 13;22(7):e16649. doi: 10.2196/16649. J Med Internet Res. 2020. PMID: 32673231 Free PMC article.
-
Should AI allocate livers for transplant? Public attitudes and ethical considerations.BMC Med Ethics. 2023 Nov 27;24(1):102. doi: 10.1186/s12910-023-00983-0. BMC Med Ethics. 2023. PMID: 38012660 Free PMC article.
-
Responsible AI for cardiovascular disease detection: Towards a privacy-preserving and interpretable model.Comput Methods Programs Biomed. 2024 Sep;254:108289. doi: 10.1016/j.cmpb.2024.108289. Epub 2024 Jun 17. Comput Methods Programs Biomed. 2024. PMID: 38905988
-
Exploring stakeholder attitudes towards AI in clinical practice.BMJ Health Care Inform. 2021 Dec;28(1):e100450. doi: 10.1136/bmjhci-2021-100450. BMJ Health Care Inform. 2021. PMID: 34887331 Free PMC article. Review.
-
Surveying Public Perceptions of Artificial Intelligence in Health Care in the United States: Systematic Review.J Med Internet Res. 2023 Apr 4;25:e40337. doi: 10.2196/40337. J Med Internet Res. 2023. PMID: 37014676 Free PMC article. Review.
Cited by
-
"Just" accuracy? Procedural fairness demands explainability in AI-based medical resource allocations.AI Soc. 2022 Dec 21:1-12. doi: 10.1007/s00146-022-01614-9. Online ahead of print. AI Soc. 2022. PMID: 36573157 Free PMC article.
-
Informing antimicrobial stewardship with explainable AI.PLOS Digit Health. 2023 Jan 5;2(1):e0000162. doi: 10.1371/journal.pdig.0000162. eCollection 2023 Jan. PLOS Digit Health. 2023. PMID: 36812617 Free PMC article.
-
Targeting Machine Learning and Artificial Intelligence Algorithms in Health Care to Reduce Bias and Improve Population Health.Milbank Q. 2024 Sep;102(3):577-604. doi: 10.1111/1468-0009.12712. Epub 2024 Aug 8. Milbank Q. 2024. PMID: 39116187
-
The limitations of machine learning models for predicting scientific replicability.Proc Natl Acad Sci U S A. 2023 Aug 15;120(33):e2307596120. doi: 10.1073/pnas.2307596120. Epub 2023 Aug 7. Proc Natl Acad Sci U S A. 2023. PMID: 37549293 Free PMC article. No abstract available.
-
Psychological factors underlying attitudes toward AI tools.Nat Hum Behav. 2023 Nov;7(11):1845-1854. doi: 10.1038/s41562-023-01734-2. Epub 2023 Nov 20. Nat Hum Behav. 2023. PMID: 37985913 Review.
References
-
- Artificial intelligence in transport: Current and future developments, opportunities and challenges. Think Tank, European Parliament [Policy Briefing, 2019]. https://www.europarl.europa.eu/thinktank/en/document/EPRS_BRI(2019)635609.
-
- Aletras N, Tsarapatsanis D, Preoţiuc-Pietro D, Lampos V. Predicting judicial decisions of the European Court of Human Rights: a natural language processing perspective. PeerJ Comput. Sci. 2016;2:e93. doi: 10.7717/peerj-cs.93. - DOI
Publication types
MeSH terms
LinkOut - more resources
Full Text Sources
Research Materials