Multi-Feature Intelligent Oral English Error Correction Based on Few-Shot Learning Technology
- PMID: 35785085
- PMCID: PMC9246633
- DOI: 10.1155/2022/2501693
Multi-Feature Intelligent Oral English Error Correction Based on Few-Shot Learning Technology
Abstract
The computer-aided language teaching system is maturing thanks to the advancement of few-shot learning technologies. In order to support teachers and increase students' learning efficiency, more computer-aided language teaching systems are being used in teaching and examinations. This study focuses on a multifeature fusion-based evaluation method for oral English learning, completely evaluating specific grammar, and assisting oral learners in improving their oral pronunciation skills. This study proposes an improved method based on HMM a posteriori probability scoring, in which the only standard reference model is no longer used as the basis for scoring and error determination, and instead, the average level of standard pronunciation in the entire corpus is introduced as another judgment basis, based on a preliminary study of speech recognition technology, scoring methods, and relevant theoretical knowledge of information feedback. This strategy can reduce the score limitation caused by standard pronunciation personal differences, lower the system's misjudgment rate in detecting pronunciation errors, and improve the usefulness of error correction information. An expert opinion database has been created based on the most prevalent forms of spoken pronunciation problems, which can successfully assist learners improve their spoken English level by combining the database's corrected information. Finally, this study proposes an artificial scoring system for spoken English that performs activities such as identification, scoring, error judgment, and correction opinion feedback, among others. Finally, it has been demonstrated through trials and tests that adding the average pronunciation level to the system improves the system's scoring performance and has a certain effect on increasing users' oral pronunciation level.
Copyright © 2022 Fengxiang Zhang and Jieli Sun.
Conflict of interest statement
The authors do not have any possible conflicts of interest.
Figures
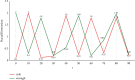
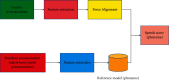
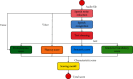
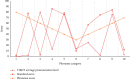
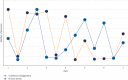
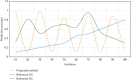
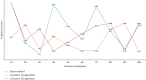
Similar articles
-
English Speech Recognition System Model Based on Computer-Aided Function and Neural Network Algorithm.Comput Intell Neurosci. 2022 Apr 22;2022:7846877. doi: 10.1155/2022/7846877. eCollection 2022. Comput Intell Neurosci. 2022. Retraction in: Comput Intell Neurosci. 2023 Jul 26;2023:9845318. doi: 10.1155/2023/9845318 PMID: 35498214 Free PMC article. Retracted.
-
Research on Open Oral English Scoring System Based on Neural Network.Comput Intell Neurosci. 2022 Apr 23;2022:1346543. doi: 10.1155/2022/1346543. eCollection 2022. Comput Intell Neurosci. 2022. Retraction in: Comput Intell Neurosci. 2023 Jul 12;2023:9847201. doi: 10.1155/2023/9847201 PMID: 35502353 Free PMC article. Retracted.
-
The impact of automatic speech recognition technology on second language pronunciation and speaking skills of EFL learners: a mixed methods investigation.Front Psychol. 2023 Aug 16;14:1210187. doi: 10.3389/fpsyg.2023.1210187. eCollection 2023. Front Psychol. 2023. PMID: 37663357 Free PMC article.
-
Application of Ontology Matching Algorithm Based on Linguistic Features in English Pronunciation Quality Evaluation.Occup Ther Int. 2022 Jun 28;2022:2734672. doi: 10.1155/2022/2734672. eCollection 2022. Occup Ther Int. 2022. Retraction in: Occup Ther Int. 2023 Aug 16;2023:9864918. doi: 10.1155/2023/9864918 PMID: 35844241 Free PMC article. Retracted.
-
Does a simulation-based learning programme assist with the development of speech-language pathology students' clinical skills in stuttering management?Int J Lang Commun Disord. 2021 Nov;56(6):1334-1346. doi: 10.1111/1460-6984.12670. Epub 2021 Sep 14. Int J Lang Commun Disord. 2021. PMID: 34519389 Review.
References
-
- Hai Y. Computer-aided teaching mode of oral English intelligent learning based on speech recognition and network assistance. Journal of Intelligent and Fuzzy Systems . 2020;39(4):5749–5760. doi: 10.3233/jifs-189052. - DOI
-
- Duan R., Wang Y., Qin H. Artificial intelligence speech recognition model for correcting spoken English teaching. Journal of Intelligent and Fuzzy Systems . 2020;40(1):1–12. doi: 10.3233/JIFS-189388. - DOI
-
- Herbert D., Kang B. H. Intelligent conversation system using multiple classification ripple down rules and conversational context. Expert Systems with Applications . 2018;112(DEC):342–352. doi: 10.1016/j.eswa.2018.06.049. - DOI
-
- Lee S., Noh H., Lee J., Lee K., Lee G. G. Foreign language tutoring in oral conversations using spoken dialog systems. IEICE - Transactions on Info and Systems . 2017;95(5):1216–1228. doi: 10.1587/transinf.E95.D.1216. - DOI
MeSH terms
LinkOut - more resources
Full Text Sources