Utilizing Synthetic Nodules for Improving Nodule Detection in Chest Radiographs
- PMID: 35304676
- PMCID: PMC9485384
- DOI: 10.1007/s10278-022-00608-9
Utilizing Synthetic Nodules for Improving Nodule Detection in Chest Radiographs
Abstract
Algorithms that automatically identify nodular patterns in chest X-ray (CXR) images could benefit radiologists by reducing reading time and improving accuracy. A promising approach is to use deep learning, where a deep neural network (DNN) is trained to classify and localize nodular patterns (including mass) in CXR images. Such algorithms, however, require enough abnormal cases to learn representations of nodular patterns arising in practical clinical settings. Obtaining large amounts of high-quality data is impractical in medical imaging where (1) acquiring labeled images is extremely expensive, (2) annotations are subject to inaccuracies due to the inherent difficulty in interpreting images, and (3) normal cases occur far more frequently than abnormal cases. In this work, we devise a framework to generate realistic nodules and demonstrate how they can be used to train a DNN identify and localize nodular patterns in CXR images. While most previous research applying generative models to medical imaging are limited to generating visually plausible abnormalities and using these patterns for augmentation, we go a step further to show how the training algorithm can be adjusted accordingly to maximally benefit from synthetic abnormal patterns. A high-precision detection model was first developed and tested on internal and external datasets, and the proposed method was shown to enhance the model's recall while retaining the low level of false positives.
Keywords: Chest radiographs; Computer-aided detection; Generative adversarial networks; Online data augmentation.
© 2022. The Author(s) under exclusive licence to Society for Imaging Informatics in Medicine.
Conflict of interest statement
Minki Chung, Seo Taek Kong, Beomhee Park, Younjoon Chung and Kyu-Hwan Jung are employees of VUNO Inc. Kyu-Hwan Jung is an equity holder of VUNO Inc.
Figures
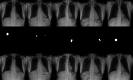
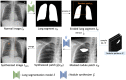
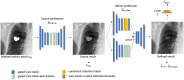
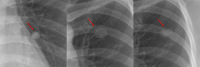
Similar articles
-
Computer-aided diagnostic scheme for the detection of lung nodules on chest radiographs: localized search method based on anatomical classification.Med Phys. 2006 Jul;33(7):2642-53. doi: 10.1118/1.2208739. Med Phys. 2006. PMID: 16898468
-
Optimal matrix size of chest radiographs for computer-aided detection on lung nodule or mass with deep learning.Eur Radiol. 2020 Sep;30(9):4943-4951. doi: 10.1007/s00330-020-06892-9. Epub 2020 Apr 29. Eur Radiol. 2020. PMID: 32350657
-
Better performance of deep learning pulmonary nodule detection using chest radiography with pixel level labels in reference to computed tomography: data quality matters.Sci Rep. 2024 Jul 10;14(1):15967. doi: 10.1038/s41598-024-66530-y. Sci Rep. 2024. PMID: 38987309 Free PMC article.
-
A review on lung boundary detection in chest X-rays.Int J Comput Assist Radiol Surg. 2019 Apr;14(4):563-576. doi: 10.1007/s11548-019-01917-1. Epub 2019 Feb 7. Int J Comput Assist Radiol Surg. 2019. PMID: 30730032 Free PMC article. Review.
-
Is there an advantage to using computer aided detection for the early detection of pulmonary nodules within chest X-Ray imaging?Radiography (Lond). 2020 Aug;26(3):e170-e178. doi: 10.1016/j.radi.2020.01.002. Epub 2020 Jan 29. Radiography (Lond). 2020. PMID: 32052750 Review.
Cited by
-
Updated Primer on Generative Artificial Intelligence and Large Language Models in Medical Imaging for Medical Professionals.Korean J Radiol. 2024 Mar;25(3):224-242. doi: 10.3348/kjr.2023.0818. Korean J Radiol. 2024. PMID: 38413108 Free PMC article. Review.
-
Detection of pulmonary nodules in chest radiographs: novel cost function for effective network training with purely synthesized datasets.Int J Comput Assist Radiol Surg. 2024 Oct;19(10):1991-2000. doi: 10.1007/s11548-024-03227-7. Epub 2024 Jul 13. Int J Comput Assist Radiol Surg. 2024. PMID: 39003437 Free PMC article.
-
Overcoming the Challenges in the Development and Implementation of Artificial Intelligence in Radiology: A Comprehensive Review of Solutions Beyond Supervised Learning.Korean J Radiol. 2023 Nov;24(11):1061-1080. doi: 10.3348/kjr.2023.0393. Epub 2023 Aug 28. Korean J Radiol. 2023. PMID: 37724586 Free PMC article. Review.
-
A systematic approach to deep learning-based nodule detection in chest radiographs.Sci Rep. 2023 Jun 21;13(1):10120. doi: 10.1038/s41598-023-37270-2. Sci Rep. 2023. PMID: 37344565 Free PMC article.
References
-
- Irvin, J., Rajpurkar, P., Ko, M., Yu, Y., Ciurea-Ilcus, S., Chute, C., Marklund, H., Haghgoo, B., Ball, R., Shpanskaya, K., et al.: Chexpert: A large chest radiograph dataset with uncertainty labels and expert comparison. In: Proceedings of the AAAI Conference on Artificial Intelligence. vol. 33, pp. 590–597 (2019)
-
- Wang, X., Peng, Y., Lu, L., Lu, Z., Bagheri, M., Summers, R.M.: Chestx-ray8: Hospital-scale chest x-ray database and benchmarks on weakly-supervised classification and localization of common thorax diseases. In: Proceedings of the IEEE conference on computer vision and pattern recognition. pp. 2097–2106 (2017)
-
- Berthelot, D., Carlini, N., Cubuk, E.D., Kurakin, A., Sohn, K., Zhang, H., Raffel, C.: Remixmatch: Semi-supervised learning with distribution matching and augmentation anchoring. In: International Conference on Learning Representations (2020)
-
- Cubuk, E.D., Zoph, B., Shlens, J., Le, Q.V.: Randaugment: Practical automated data augmentation with a reduced search space. In: Proceedings of the IEEE/CVF Conference on Computer Vision and Pattern Recognition Workshops. pp. 702–703 (2020)
MeSH terms
LinkOut - more resources
Full Text Sources