Developmental and evolutionary constraints on olfactory circuit selection
- PMID: 35263217
- PMCID: PMC8931209
- DOI: 10.1073/pnas.2100600119
Developmental and evolutionary constraints on olfactory circuit selection
Abstract
SignificanceIn this work, we explore the hypothesis that biological neural networks optimize their architecture, through evolution, for learning. We study early olfactory circuits of mammals and insects, which have relatively similar structure but a huge diversity in size. We approximate these circuits as three-layer networks and estimate, analytically, the scaling of the optimal hidden-layer size with input-layer size. We find that both longevity and information in the genome constrain the hidden-layer size, so a range of allometric scalings is possible. However, the experimentally observed allometric scalings in mammals and insects are consistent with biologically plausible values. This analysis should pave the way for a deeper understanding of both biological and artificial networks.
Keywords: model selection; neural circuit; olfaction; statistical learning theory.
Conflict of interest statement
The authors declare no competing interest.
Figures
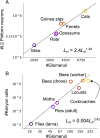
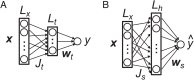
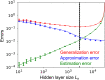
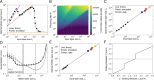
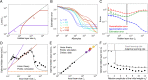
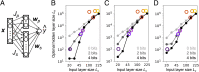
Similar articles
-
Learning classification in the olfactory system of insects.Neural Comput. 2004 Aug;16(8):1601-40. doi: 10.1162/089976604774201613. Neural Comput. 2004. PMID: 15228747
-
The maturational characteristics of the GABA input in the anterior piriform cortex may also contribute to the rapid learning of the maternal odor during the sensitive period.Learn Mem. 2020 Nov 16;27(12):493-502. doi: 10.1101/lm.052217.120. Print 2020 Dec. Learn Mem. 2020. PMID: 33199474 Free PMC article.
-
Scaling Principles of Distributed Circuits.Curr Biol. 2019 Aug 5;29(15):2533-2540.e7. doi: 10.1016/j.cub.2019.06.046. Epub 2019 Jul 18. Curr Biol. 2019. PMID: 31327712
-
Common design of mushroom bodies in bees and flies?J Comp Neurol. 2002 Aug 12;450(1):1-3. doi: 10.1002/cne.10326. J Comp Neurol. 2002. PMID: 12124763 Review. No abstract available.
-
Mushroom body memoir: from maps to models.Nat Rev Neurosci. 2003 Apr;4(4):266-75. doi: 10.1038/nrn1074. Nat Rev Neurosci. 2003. PMID: 12671643 Review. No abstract available.
Cited by
-
Input density tunes Kenyon cell sensory responses in the Drosophila mushroom body.Curr Biol. 2023 Jul 10;33(13):2742-2760.e12. doi: 10.1016/j.cub.2023.05.064. Epub 2023 Jun 21. Curr Biol. 2023. PMID: 37348501 Free PMC article.
-
Biologically plausible local synaptic learning rules robustly implement deep supervised learning.Front Neurosci. 2023 Oct 11;17:1160899. doi: 10.3389/fnins.2023.1160899. eCollection 2023. Front Neurosci. 2023. PMID: 37886676 Free PMC article.
-
High-throughput sequencing of single neuron projections reveals spatial organization in the olfactory cortex.Cell. 2022 Oct 27;185(22):4117-4134.e28. doi: 10.1016/j.cell.2022.09.038. Cell. 2022. PMID: 36306734 Free PMC article.
-
Hacking brain development to test models of sensory coding.bioRxiv [Preprint]. 2023 Jan 26:2023.01.25.525425. doi: 10.1101/2023.01.25.525425. bioRxiv. 2023. PMID: 36747712 Free PMC article. Preprint.
-
Distinctive properties of biological neural networks and recent advances in bottom-up approaches toward a better biologically plausible neural network.Front Comput Neurosci. 2023 Jun 28;17:1092185. doi: 10.3389/fncom.2023.1092185. eCollection 2023. Front Comput Neurosci. 2023. PMID: 37449083 Free PMC article. Review.
References
-
- Mathis A., Herz A. V., Stemmler M., Optimal population codes for space: Grid cells outperform place cells. Neural Comput. 24, 2280–2317 (2012). - PubMed
-
- Akaike H., A new look at the statistical model identification. IEEE Trans. Automat. Contr. 19, 716–723 (1974).
-
- Baum E. B., Haussler D., “What size net gives valid generalization?” in Advances in Neural Information Processing Systems, Touretzky D., Ed. (NIPS, 1988), vol. 1, pp. 81–90.
MeSH terms
Grants and funding
LinkOut - more resources
Full Text Sources