Cell-type-specific neuromodulation guides synaptic credit assignment in a spiking neural network
- PMID: 34916291
- PMCID: PMC8713766
- DOI: 10.1073/pnas.2111821118
Cell-type-specific neuromodulation guides synaptic credit assignment in a spiking neural network
Abstract
Brains learn tasks via experience-driven differential adjustment of their myriad individual synaptic connections, but the mechanisms that target appropriate adjustment to particular connections remain deeply enigmatic. While Hebbian synaptic plasticity, synaptic eligibility traces, and top-down feedback signals surely contribute to solving this synaptic credit-assignment problem, alone, they appear to be insufficient. Inspired by new genetic perspectives on neuronal signaling architectures, here, we present a normative theory for synaptic learning, where we predict that neurons communicate their contribution to the learning outcome to nearby neurons via cell-type-specific local neuromodulation. Computational tests suggest that neuron-type diversity and neuron-type-specific local neuromodulation may be critical pieces of the biological credit-assignment puzzle. They also suggest algorithms for improved artificial neural network learning efficiency.
Keywords: cell types; credit assignment; neuromodulation; neuropeptides; spiking neural network.
Copyright © 2021 the Author(s). Published by PNAS.
Conflict of interest statement
The authors declare no competing interest.
Figures
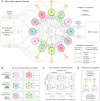
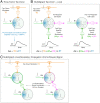
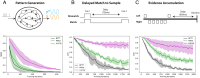
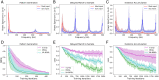
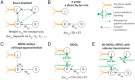
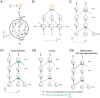
Similar articles
-
Spatio-temporal credit assignment in neuronal population learning.PLoS Comput Biol. 2011 Jun;7(6):e1002092. doi: 10.1371/journal.pcbi.1002092. Epub 2011 Jun 30. PLoS Comput Biol. 2011. PMID: 21738460 Free PMC article.
-
Synaptic dynamics: linear model and adaptation algorithm.Neural Netw. 2014 Aug;56:49-68. doi: 10.1016/j.neunet.2014.04.001. Epub 2014 Apr 28. Neural Netw. 2014. PMID: 24867390
-
Reinforcement learning of targeted movement in a spiking neuronal model of motor cortex.PLoS One. 2012;7(10):e47251. doi: 10.1371/journal.pone.0047251. Epub 2012 Oct 19. PLoS One. 2012. PMID: 23094042 Free PMC article.
-
Introduction to spiking neural networks: Information processing, learning and applications.Acta Neurobiol Exp (Wars). 2011;71(4):409-33. doi: 10.55782/ane-2011-1862. Acta Neurobiol Exp (Wars). 2011. PMID: 22237491 Review.
-
Synaptic Plasticity Forms and Functions.Annu Rev Neurosci. 2020 Jul 8;43:95-117. doi: 10.1146/annurev-neuro-090919-022842. Epub 2020 Feb 19. Annu Rev Neurosci. 2020. PMID: 32075520 Review.
Cited by
-
Transition to chaos separates learning regimes and relates to measure of consciousness in recurrent neural networks.bioRxiv [Preprint]. 2024 May 15:2024.05.15.594236. doi: 10.1101/2024.05.15.594236. bioRxiv. 2024. PMID: 38798582 Free PMC article. Preprint.
-
The reciprocity of skeletal muscle and bone: an evolving view from mechanical coupling, secretory crosstalk to stem cell exchange.Front Physiol. 2024 Mar 4;15:1349253. doi: 10.3389/fphys.2024.1349253. eCollection 2024. Front Physiol. 2024. PMID: 38505709 Free PMC article. Review.
-
A role for cortical interneurons as adversarial discriminators.PLoS Comput Biol. 2023 Sep 28;19(9):e1011484. doi: 10.1371/journal.pcbi.1011484. eCollection 2023 Sep. PLoS Comput Biol. 2023. PMID: 37768890 Free PMC article.
-
Information Transmission in G Protein-Coupled Receptors.Int J Mol Sci. 2024 Jan 28;25(3):1621. doi: 10.3390/ijms25031621. Int J Mol Sci. 2024. PMID: 38338905 Free PMC article. Review.
-
A Molecular Landscape of Mouse Hippocampal Neuromodulation.Front Neural Circuits. 2022 May 6;16:836930. doi: 10.3389/fncir.2022.836930. eCollection 2022. Front Neural Circuits. 2022. PMID: 35601530 Free PMC article.
References
-
- LeCun Y., Bengio Y., Hinton G., Deep learning. Nature 521, 436–444 (2015). - PubMed
-
- Sejnowski T. J., The Deep Learning Revolution (MIT Press, Cambridge, MA, 2018).
-
- Williams R. J., Zipser D., “Gradient-based learning algorithms for recurrent networks and their computational complexity” in Back-Propagation: Theory, Architectures and Applications, Chauvin Y., Rumelhart D. E., Eds. (Erlbaum, Hillsdale, NJ, 1995), pp. 433–486.
-
- Marschall O., Cho K., Savin C., A unified framework of online learning algorithms for training recurrent neural networks. J. Mach. Learn. Res. 21, 1–34 (2020). - PubMed
-
- Mujika A., Meier F., Steger A., “Approximating real-time recurrent learning with random Kronecker factors” in 32nd Conference on Neural Information Processing Systems, Bengio S., Wallach H. M., Larochelle H., Grauman K., Cesa-Bianchi N., Eds. (Curran Associates Inc., Red Hook, NY, 2018), pp. 6594–6603.
Publication types
MeSH terms
Substances
LinkOut - more resources
Full Text Sources