A Correspondence Between Normalization Strategies in Artificial and Biological Neural Networks
- PMID: 34474484
- PMCID: PMC8662716
- DOI: 10.1162/neco_a_01439
A Correspondence Between Normalization Strategies in Artificial and Biological Neural Networks
Abstract
A fundamental challenge at the interface of machine learning and neuroscience is to uncover computational principles that are shared between artificial and biological neural networks. In deep learning, normalization methods such as batch normalization, weight normalization, and their many variants help to stabilize hidden unit activity and accelerate network training, and these methods have been called one of the most important recent innovations for optimizing deep networks. In the brain, homeostatic plasticity represents a set of mechanisms that also stabilize and normalize network activity to lie within certain ranges, and these mechanisms are critical for maintaining normal brain function. In this article, we discuss parallels between artificial and biological normalization methods at four spatial scales: normalization of a single neuron's activity, normalization of synaptic weights of a neuron, normalization of a layer of neurons, and normalization of a network of neurons. We argue that both types of methods are functionally equivalent-that is, both push activation patterns of hidden units toward a homeostatic state, where all neurons are equally used-and we argue that such representations can improve coding capacity, discrimination, and regularization. As a proof of concept, we develop an algorithm, inspired by a neural normalization technique called synaptic scaling, and show that this algorithm performs competitively against existing normalization methods on several data sets. Overall, we hope this bidirectional connection will inspire neuroscientists and machine learners in three ways: to uncover new normalization algorithms based on established neurobiological principles; to help quantify the trade-offs of different homeostatic plasticity mechanisms used in the brain; and to offer insights about how stability may not hinder, but may actually promote, plasticity.
© 2021 Massachusetts Institute of Technology.
Figures
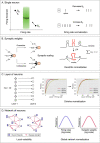
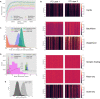
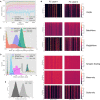
Similar articles
-
Deep convolutional neural network and IoT technology for healthcare.Digit Health. 2024 Jan 17;10:20552076231220123. doi: 10.1177/20552076231220123. eCollection 2024 Jan-Dec. Digit Health. 2024. PMID: 38250147 Free PMC article.
-
Fast deep neural correspondence for tracking and identifying neurons in C. elegans using semi-synthetic training.Elife. 2021 Jul 14;10:e66410. doi: 10.7554/eLife.66410. Elife. 2021. PMID: 34259623 Free PMC article.
-
Synaptic Scaling-An Artificial Neural Network Regularization Inspired by Nature.IEEE Trans Neural Netw Learn Syst. 2022 Jul;33(7):3094-3108. doi: 10.1109/TNNLS.2021.3050422. Epub 2022 Jul 6. IEEE Trans Neural Netw Learn Syst. 2022. PMID: 33502984
-
Modern Artificial Neural Networks: Is Evolution Cleverer?Neural Comput. 2023 Apr 18;35(5):763-806. doi: 10.1162/neco_a_01575. Neural Comput. 2023. PMID: 36944238 Review.
-
A review of learning in biologically plausible spiking neural networks.Neural Netw. 2020 Feb;122:253-272. doi: 10.1016/j.neunet.2019.09.036. Epub 2019 Oct 11. Neural Netw. 2020. PMID: 31726331 Review.
Cited by
-
Machine-Learning-Based Identification of Key Feature RNA-Signature Linked to Diagnosis of Hepatocellular Carcinoma.J Clin Exp Hepatol. 2024 Nov-Dec;14(6):101456. doi: 10.1016/j.jceh.2024.101456. Epub 2024 Jun 14. J Clin Exp Hepatol. 2024. PMID: 39055616
-
Rethinking the Role of Normalization and Residual Blocks for Spiking Neural Networks.Sensors (Basel). 2022 Apr 8;22(8):2876. doi: 10.3390/s22082876. Sensors (Basel). 2022. PMID: 35458860 Free PMC article.
-
Building transformers from neurons and astrocytes.Proc Natl Acad Sci U S A. 2023 Aug 22;120(34):e2219150120. doi: 10.1073/pnas.2219150120. Epub 2023 Aug 14. Proc Natl Acad Sci U S A. 2023. PMID: 37579149 Free PMC article.
-
Distinctive properties of biological neural networks and recent advances in bottom-up approaches toward a better biologically plausible neural network.Front Comput Neurosci. 2023 Jun 28;17:1092185. doi: 10.3389/fncom.2023.1092185. eCollection 2023. Front Comput Neurosci. 2023. PMID: 37449083 Free PMC article. Review.
References
-
- Arora, S., Li, Z., & Lyu, K. (2019). Theoretical analysis of auto rate-tuning by batch normalization. In Proceedings of the 7th International Conference on Learning Representation. OpenReview.net.
-
- Arpit, D., Zhou, Y., Kota, B. U., & Govindaraju, V. (2016). Normalization propagation: A parametric technique for removing internal covariate shift in deep networks. In Proceedings of the 33rd International Conference on Machine Learning (pp. 1168–1176).
-
- Bjorck, N., Gomes, C. P., Selman, B., & Weinberger, K. Q. (2018). Understanding batch normalization. In Bengio S., Wallach H., Larochelle H., Grauman K., Cesa-Bianchi N., & Garnett R., R. (Eds.), Advances in neural information processing systems, 31 (pp. 7694–7705). Red Hook, NY: Curran.
Publication types
MeSH terms
Grants and funding
LinkOut - more resources
Full Text Sources
Research Materials