Bias and fairness assessment of a natural language processing opioid misuse classifier: detection and mitigation of electronic health record data disadvantages across racial subgroups
- PMID: 34383925
- PMCID: PMC8510285
- DOI: 10.1093/jamia/ocab148
Bias and fairness assessment of a natural language processing opioid misuse classifier: detection and mitigation of electronic health record data disadvantages across racial subgroups
Abstract
Objectives: To assess fairness and bias of a previously validated machine learning opioid misuse classifier.
Materials & methods: Two experiments were conducted with the classifier's original (n = 1000) and external validation (n = 53 974) datasets from 2 health systems. Bias was assessed via testing for differences in type II error rates across racial/ethnic subgroups (Black, Hispanic/Latinx, White, Other) using bootstrapped 95% confidence intervals. A local surrogate model was estimated to interpret the classifier's predictions by race and averaged globally from the datasets. Subgroup analyses and post-hoc recalibrations were conducted to attempt to mitigate biased metrics.
Results: We identified bias in the false negative rate (FNR = 0.32) of the Black subgroup compared to the FNR (0.17) of the White subgroup. Top features included "heroin" and "substance abuse" across subgroups. Post-hoc recalibrations eliminated bias in FNR with minimal changes in other subgroup error metrics. The Black FNR subgroup had higher risk scores for readmission and mortality than the White FNR subgroup, and a higher mortality risk score than the Black true positive subgroup (P < .05).
Discussion: The Black FNR subgroup had the greatest severity of disease and risk for poor outcomes. Similar features were present between subgroups for predicting opioid misuse, but inequities were present. Post-hoc mitigation techniques mitigated bias in type II error rate without creating substantial type I error rates. From model design through deployment, bias and data disadvantages should be systematically addressed.
Conclusion: Standardized, transparent bias assessments are needed to improve trustworthiness in clinical machine learning models.
Keywords: bias and fairness; interpretability; machine learning; natural language processing; opioid use disorder; structural racism.
© The Author(s) 2021. Published by Oxford University Press on behalf of the American Medical Informatics Association.
Figures
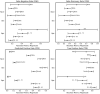
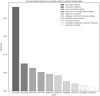
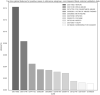
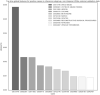
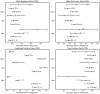
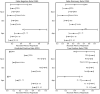
Similar articles
-
Fairness in Predicting Cancer Mortality Across Racial Subgroups.JAMA Netw Open. 2024 Jul 1;7(7):e2421290. doi: 10.1001/jamanetworkopen.2024.21290. JAMA Netw Open. 2024. PMID: 38985468 Free PMC article.
-
Publicly available machine learning models for identifying opioid misuse from the clinical notes of hospitalized patients.BMC Med Inform Decis Mak. 2020 Apr 29;20(1):79. doi: 10.1186/s12911-020-1099-y. BMC Med Inform Decis Mak. 2020. PMID: 32349766 Free PMC article.
-
Assessing fairness in machine learning models: A study of racial bias using matched counterparts in mortality prediction for patients with chronic diseases.J Biomed Inform. 2024 Aug;156:104677. doi: 10.1016/j.jbi.2024.104677. Epub 2024 Jun 13. J Biomed Inform. 2024. PMID: 38876453
-
Impact of Healthcare Algorithms on Racial and Ethnic Disparities in Health and Healthcare [Internet].Rockville (MD): Agency for Healthcare Research and Quality (US); 2023 Dec. Report No.: 24-EHC004. Rockville (MD): Agency for Healthcare Research and Quality (US); 2023 Dec. Report No.: 24-EHC004. PMID: 38147523 Free Books & Documents. Review.
-
Evaluation and Mitigation of Racial Bias in Clinical Machine Learning Models: Scoping Review.JMIR Med Inform. 2022 May 31;10(5):e36388. doi: 10.2196/36388. JMIR Med Inform. 2022. PMID: 35639450 Free PMC article. Review.
Cited by
-
Evaluating and mitigating unfairness in multimodal remote mental health assessments.PLOS Digit Health. 2024 Jul 24;3(7):e0000413. doi: 10.1371/journal.pdig.0000413. eCollection 2024 Jul. PLOS Digit Health. 2024. PMID: 39046989 Free PMC article.
-
Characterizing subgroup performance of probabilistic phenotype algorithms within older adults: a case study for dementia, mild cognitive impairment, and Alzheimer's and Parkinson's diseases.JAMIA Open. 2023 Jun 28;6(2):ooad043. doi: 10.1093/jamiaopen/ooad043. eCollection 2023 Jul. JAMIA Open. 2023. PMID: 37397506 Free PMC article.
-
Disparities in seizure outcomes revealed by large language models.J Am Med Inform Assoc. 2024 May 20;31(6):1348-1355. doi: 10.1093/jamia/ocae047. J Am Med Inform Assoc. 2024. PMID: 38481027
-
Sex-Based Performance Disparities in Machine Learning Algorithms for Cardiac Disease Prediction: Exploratory Study.J Med Internet Res. 2024 Aug 26;26:e46936. doi: 10.2196/46936. J Med Internet Res. 2024. PMID: 39186324 Free PMC article.
-
Racial Disparities in the Ascertainment of Cancer Recurrence in Electronic Health Records.JCO Clin Cancer Inform. 2023 Jun;7:e2300004. doi: 10.1200/CCI.23.00004. JCO Clin Cancer Inform. 2023. PMID: 37267516 Free PMC article.
References
-
- Allen B, Agarwal S, Kalpathy-Cramer J, et al.Democratizing AI. J Am Coll Radiol 2019; 16 (7): 961–3. - PubMed
-
- Gupta V, Roth H, Buch V, et al. Democratizing artificial intelligence in healthcare: a study of model development across 2 institutions incorporating transfer learning. arXiv[eess.IV], http://arxiv.org/abs/2009.12437, 2020, preprint: not peer reviewed.
Publication types
MeSH terms
Grants and funding
- R01LM012973/LM/NLM NIH HHS/United States
- R01DA051464/DA/NIDA NIH HHS/United States
- UG1 DA049467/DA/NIDA NIH HHS/United States
- R25DA035692/DA/NIDA NIH HHS/United States
- K12 HS026385/HS/AHRQ HHS/United States
- R01 DA051464/DA/NIDA NIH HHS/United States
- R25 DA035692/DA/NIDA NIH HHS/United States
- R01DA04171/DA/NIDA NIH HHS/United States
- R01GM123193/GM/NIGMS NIH HHS/United States
- K23AA024503/AA/NIAAA NIH HHS/United States
- R01 LM012973/LM/NLM NIH HHS/United States
- R01 LM010090/LM/NLM NIH HHS/United States
- K12-HS-026385/HS/AHRQ HHS/United States
- R01 GM123193/GM/NIGMS NIH HHS/United States
- U01 TR002398/TR/NCATS NIH HHS/United States
- K23 AA024503/AA/NIAAA NIH HHS/United States
- UL1-TR-002398/TR/NCATS NIH HHS/United States
LinkOut - more resources
Full Text Sources
Medical