Towards automatic diagnosis of rheumatic heart disease on echocardiographic exams through video-based deep learning
- PMID: 34279636
- PMCID: PMC8363807
- DOI: 10.1093/jamia/ocab061
Towards automatic diagnosis of rheumatic heart disease on echocardiographic exams through video-based deep learning
Abstract
Objective: Rheumatic heart disease (RHD) affects an estimated 39 million people worldwide and is the most common acquired heart disease in children and young adults. Echocardiograms are the gold standard for diagnosis of RHD, but there is a shortage of skilled experts to allow widespread screenings for early detection and prevention of the disease progress. We propose an automated RHD diagnosis system that can help bridge this gap.
Materials and methods: Experiments were conducted on a dataset with 11 646 echocardiography videos from 912 exams, obtained during screenings in underdeveloped areas of Brazil and Uganda. We address the challenges of RHD identification with a 3D convolutional neural network (C3D), comparing its performance with a 2D convolutional neural network (VGG16) that is commonly used in the echocardiogram literature. We also propose a supervised aggregation technique to combine video predictions into a single exam diagnosis.
Results: The proposed approach obtained an accuracy of 72.77% for exam diagnosis. The results for the C3D were significantly better than the ones obtained by the VGG16 network for videos, showing the importance of considering the temporal information during the diagnostic. The proposed aggregation model showed significantly better accuracy than the majority voting strategy and also appears to be capable of capturing underlying biases in the neural network output distribution, balancing them for a more correct diagnosis.
Conclusion: Automatic diagnosis of echo-detected RHD is feasible and, with further research, has the potential to reduce the workload of experts, enabling the implementation of more widespread screening programs worldwide.
Keywords: deep learning; echocardiography; low-cost imaging; meta-learning; rheumatic heart disease; screening.
© The Author(s) 2021. Published by Oxford University Press on behalf of the American Medical Informatics Association. All rights reserved. For permissions, please email: journals.permissions@oup.com.
Figures
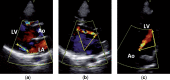
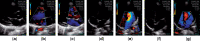
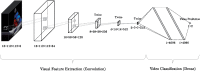
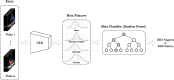
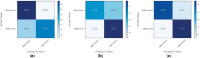
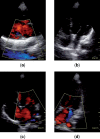
Similar articles
-
Detection of subclinical rheumatic heart disease in children using a deep learning algorithm on digital stethoscope: a study protocol.BMJ Open. 2021 Aug 5;11(8):e044070. doi: 10.1136/bmjopen-2020-044070. BMJ Open. 2021. PMID: 34353792 Free PMC article.
-
Screening for rheumatic heart disease: evaluation of a simplified echocardiography-based approach.Eur Heart J Cardiovasc Imaging. 2012 Dec;13(12):1024-9. doi: 10.1093/ehjci/jes077. Epub 2012 Apr 19. Eur Heart J Cardiovasc Imaging. 2012. PMID: 22518053
-
Echocardiographic screening of 4107 Nigerian school children for rheumatic heart disease.Trop Med Int Health. 2019 Jun;24(6):757-765. doi: 10.1111/tmi.13235. Epub 2019 Apr 8. Trop Med Int Health. 2019. PMID: 30938017 Free PMC article.
-
Rheumatic heart disease echocardiographic screening: approaching practical and affordable solutions.Heart. 2016 May;102(9):658-64. doi: 10.1136/heartjnl-2015-308635. Epub 2016 Feb 18. Heart. 2016. PMID: 26891757 Review.
-
World Heart Federation criteria for echocardiographic diagnosis of rheumatic heart disease--an evidence-based guideline.Nat Rev Cardiol. 2012 Feb 28;9(5):297-309. doi: 10.1038/nrcardio.2012.7. Nat Rev Cardiol. 2012. PMID: 22371105 Free PMC article. Review.
Cited by
-
Data harnessing to nurture the human mind for a tailored approach to the child.Pediatr Res. 2023 Jan;93(2):357-365. doi: 10.1038/s41390-022-02320-4. Epub 2022 Sep 30. Pediatr Res. 2023. PMID: 36180585 Review.
-
Reasons for disagreement between screening and standard echocardiography in primary care: data from the PROVAR + study : Disagreement between screening and standard echo.Int J Cardiovasc Imaging. 2023 May;39(5):929-937. doi: 10.1007/s10554-023-02800-6. Epub 2023 Jan 21. Int J Cardiovasc Imaging. 2023. PMID: 36680683
-
The Role of Artificial Intelligence in Echocardiography.J Imaging. 2023 Feb 20;9(2):50. doi: 10.3390/jimaging9020050. J Imaging. 2023. PMID: 36826969 Free PMC article. Review.
-
An Automated View Classification Model for Pediatric Echocardiography Using Artificial Intelligence.J Am Soc Echocardiogr. 2022 Dec;35(12):1238-1246. doi: 10.1016/j.echo.2022.08.009. Epub 2022 Aug 29. J Am Soc Echocardiogr. 2022. PMID: 36049595 Free PMC article.
-
Deep learning for automated left ventricular outflow tract diameter measurements in 2D echocardiography.Cardiovasc Ultrasound. 2023 Oct 13;21(1):19. doi: 10.1186/s12947-023-00317-5. Cardiovasc Ultrasound. 2023. PMID: 37833731 Free PMC article.
References
-
- Cohen MG, Fonarow GC, Peterson ED, et al.Racial and ethnic differences in the treatment of acute myocardial infarction. Circulation 2010; 121 (21): 2294–301. - PubMed
-
- James SL, Abate D, Abate KH, et al.Global, regional, and national incidence, prevalence, and years lived with disability for 354 diseases and injuries for 195 countries and territories, 1990–2017: a systematic analysis for the global burden of disease study 2017. Lancet 2018; 392 (10159): 1789–858. - PMC - PubMed
Publication types
MeSH terms
LinkOut - more resources
Full Text Sources
Miscellaneous