Deep learning-based automated detection for diabetic retinopathy and diabetic macular oedema in retinal fundus photographs
- PMID: 34211137
- PMCID: PMC9232645
- DOI: 10.1038/s41433-021-01552-8
Deep learning-based automated detection for diabetic retinopathy and diabetic macular oedema in retinal fundus photographs
Abstract
Objectives: To present and validate a deep ensemble algorithm to detect diabetic retinopathy (DR) and diabetic macular oedema (DMO) using retinal fundus images.
Methods: A total of 8739 retinal fundus images were collected from a retrospective cohort of 3285 patients. For detecting DR and DMO, a multiple improved Inception-v4 ensembling approach was developed. We measured the algorithm's performance and made a comparison with that of human experts on our primary dataset, while its generalization was assessed on the publicly available Messidor-2 dataset. Also, we investigated systematically the impact of the size and number of input images used in training on model's performance, respectively. Further, the time budget of training/inference versus model performance was analyzed.
Results: On our primary test dataset, the model achieved an 0.992 (95% CI, 0.989-0.995) AUC corresponding to 0.925 (95% CI, 0.916-0.936) sensitivity and 0.961 (95% CI, 0.950-0.972) specificity for referable DR, while the sensitivity and specificity for ophthalmologists ranged from 0.845 to 0.936, and from 0.912 to 0.971, respectively. For referable DMO, our model generated an AUC of 0.994 (95% CI, 0.992-0.996) with a 0.930 (95% CI, 0.919-0.941) sensitivity and 0.971 (95% CI, 0.965-0.978) specificity, whereas ophthalmologists obtained sensitivities ranging between 0.852 and 0.946, and specificities ranging between 0.926 and 0.985.
Conclusion: This study showed that the deep ensemble model exhibited excellent performance in detecting DR and DMO, and had good robustness and generalization, which could potentially help support and expand DR/DMO screening programs.
© 2021. The Author(s), under exclusive licence to The Royal College of Ophthalmologists.
Conflict of interest statement
The authors declare no competing interests.
Figures
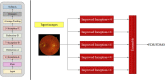
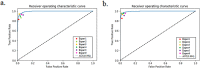
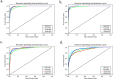
Similar articles
-
Development and Validation of a Deep Learning Algorithm for Detection of Diabetic Retinopathy in Retinal Fundus Photographs.JAMA. 2016 Dec 13;316(22):2402-2410. doi: 10.1001/jama.2016.17216. JAMA. 2016. PMID: 27898976
-
Optical coherence tomography (OCT) for detection of macular oedema in patients with diabetic retinopathy.Cochrane Database Syst Rev. 2011 Jul 6;(7):CD008081. doi: 10.1002/14651858.CD008081.pub2. Cochrane Database Syst Rev. 2011. Update in: Cochrane Database Syst Rev. 2015 Jan 07;1:CD008081. doi: 10.1002/14651858.CD008081.pub3 PMID: 21735421 Updated. Review.
-
Artificial intelligence using deep learning to screen for referable and vision-threatening diabetic retinopathy in Africa: a clinical validation study.Lancet Digit Health. 2019 May;1(1):e35-e44. doi: 10.1016/S2589-7500(19)30004-4. Epub 2019 May 2. Lancet Digit Health. 2019. PMID: 33323239
-
Evaluation of a deep learning system for the joint automated detection of diabetic retinopathy and age-related macular degeneration.Acta Ophthalmol. 2020 Jun;98(4):368-377. doi: 10.1111/aos.14306. Epub 2019 Nov 26. Acta Ophthalmol. 2020. PMID: 31773912 Free PMC article.
-
Deep learning-based detection of diabetic macular edema using optical coherence tomography and fundus images: A meta-analysis.Indian J Ophthalmol. 2023 May;71(5):1783-1796. doi: 10.4103/IJO.IJO_2614_22. Indian J Ophthalmol. 2023. PMID: 37203031 Free PMC article. Review.
Cited by
-
Ensemble of deep convolutional neural networks is more accurate and reliable than board-certified ophthalmologists at detecting multiple diseases in retinal fundus photographs.Br J Ophthalmol. 2024 Feb 21;108(3):417-423. doi: 10.1136/bjo-2022-322183. Br J Ophthalmol. 2024. PMID: 36720585 Free PMC article.
-
RETFound-enhanced community-based fundus disease screening: real-world evidence and decision curve analysis.NPJ Digit Med. 2024 Apr 30;7(1):108. doi: 10.1038/s41746-024-01109-5. NPJ Digit Med. 2024. PMID: 38693205 Free PMC article.
-
Research progress on diagnosing retinal vascular diseases based on artificial intelligence and fundus images.Front Cell Dev Biol. 2023 Mar 28;11:1168327. doi: 10.3389/fcell.2023.1168327. eCollection 2023. Front Cell Dev Biol. 2023. PMID: 37056999 Free PMC article. Review.
-
Diabetic Macular Edema Detection Using End-to-End Deep Fusion Model and Anatomical Landmark Visualization on an Edge Computing Device.Front Med (Lausanne). 2022 Apr 4;9:851644. doi: 10.3389/fmed.2022.851644. eCollection 2022. Front Med (Lausanne). 2022. PMID: 35445051 Free PMC article.
-
Efficient labeling of retinal fundus photographs using deep active learning.J Med Imaging (Bellingham). 2022 Nov;9(6):064001. doi: 10.1117/1.JMI.9.6.064001. Epub 2022 Nov 16. J Med Imaging (Bellingham). 2022. PMID: 36405815 Free PMC article.
References
-
- Gadekallu TR, Khare N, Bhattacharya S, Singh S, Maddikunta PKR, Srivastava G. Deep neural networks to predict diabetic retinopathy. J Amb Intel Hum Comp. 2020;13:1–14.
MeSH terms
LinkOut - more resources
Full Text Sources
Medical