Synaptic metaplasticity in binarized neural networks
- PMID: 33953183
- PMCID: PMC8100137
- DOI: 10.1038/s41467-021-22768-y
Synaptic metaplasticity in binarized neural networks
Abstract
While deep neural networks have surpassed human performance in multiple situations, they are prone to catastrophic forgetting: upon training a new task, they rapidly forget previously learned ones. Neuroscience studies, based on idealized tasks, suggest that in the brain, synapses overcome this issue by adjusting their plasticity depending on their past history. However, such "metaplastic" behaviors do not transfer directly to mitigate catastrophic forgetting in deep neural networks. In this work, we interpret the hidden weights used by binarized neural networks, a low-precision version of deep neural networks, as metaplastic variables, and modify their training technique to alleviate forgetting. Building on this idea, we propose and demonstrate experimentally, in situations of multitask and stream learning, a training technique that reduces catastrophic forgetting without needing previously presented data, nor formal boundaries between datasets and with performance approaching more mainstream techniques with task boundaries. We support our approach with a theoretical analysis on a tractable task. This work bridges computational neuroscience and deep learning, and presents significant assets for future embedded and neuromorphic systems, especially when using novel nanodevices featuring physics analogous to metaplasticity.
Conflict of interest statement
The authors declare no competing interests.
Figures
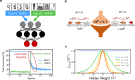
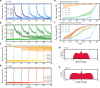
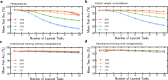
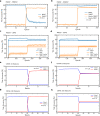
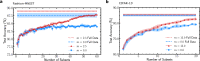
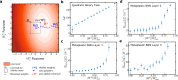
Similar articles
-
Alleviating catastrophic forgetting using context-dependent gating and synaptic stabilization.Proc Natl Acad Sci U S A. 2018 Oct 30;115(44):E10467-E10475. doi: 10.1073/pnas.1803839115. Epub 2018 Oct 12. Proc Natl Acad Sci U S A. 2018. PMID: 30315147 Free PMC article.
-
Overcoming Long-Term Catastrophic Forgetting Through Adversarial Neural Pruning and Synaptic Consolidation.IEEE Trans Neural Netw Learn Syst. 2022 Sep;33(9):4243-4256. doi: 10.1109/TNNLS.2021.3056201. Epub 2022 Aug 31. IEEE Trans Neural Netw Learn Syst. 2022. PMID: 33577459
-
Contributions by metaplasticity to solving the Catastrophic Forgetting Problem.Trends Neurosci. 2022 Sep;45(9):656-666. doi: 10.1016/j.tins.2022.06.002. Epub 2022 Jul 4. Trends Neurosci. 2022. PMID: 35798611 Review.
-
Sleep prevents catastrophic forgetting in spiking neural networks by forming a joint synaptic weight representation.PLoS Comput Biol. 2022 Nov 18;18(11):e1010628. doi: 10.1371/journal.pcbi.1010628. eCollection 2022 Nov. PLoS Comput Biol. 2022. PMID: 36399437 Free PMC article.
-
A survey on few-shot class-incremental learning.Neural Netw. 2024 Jan;169:307-324. doi: 10.1016/j.neunet.2023.10.039. Epub 2023 Oct 31. Neural Netw. 2024. PMID: 37922714 Review.
Cited by
-
Palimpsest memories stored in memristive synapses.Sci Adv. 2022 Jun 24;8(25):eabn7920. doi: 10.1126/sciadv.abn7920. Epub 2022 Jun 22. Sci Adv. 2022. PMID: 35731877 Free PMC article.
-
Eight challenges in developing theory of intelligence.Front Comput Neurosci. 2024 Jul 24;18:1388166. doi: 10.3389/fncom.2024.1388166. eCollection 2024. Front Comput Neurosci. 2024. PMID: 39114083 Free PMC article.
-
Metaplastic and energy-efficient biocompatible graphene artificial synaptic transistors for enhanced accuracy neuromorphic computing.Nat Commun. 2022 Jul 28;13(1):4386. doi: 10.1038/s41467-022-32078-6. Nat Commun. 2022. PMID: 35902599 Free PMC article.
-
Neuromorphic neuromodulation: Towards the next generation of closed-loop neurostimulation.PNAS Nexus. 2024 Oct 30;3(11):pgae488. doi: 10.1093/pnasnexus/pgae488. eCollection 2024 Nov. PNAS Nexus. 2024. PMID: 39554511 Free PMC article. Review.
-
Bayesian continual learning via spiking neural networks.Front Comput Neurosci. 2022 Nov 16;16:1037976. doi: 10.3389/fncom.2022.1037976. eCollection 2022. Front Comput Neurosci. 2022. PMID: 36465962 Free PMC article.
References
-
- Goodfellow, I. J., Mirza, M., Xiao, D., Courville, A. & Bengio, Y. An empirical investigation of catastrophic forgeting in gradientbased neural networks. In Proc. International Conference on Learning Representations ICLR (2014).
Publication types
LinkOut - more resources
Full Text Sources
Other Literature Sources
Miscellaneous