Impact of artificial intelligence support on accuracy and reading time in breast tomosynthesis image interpretation: a multi-reader multi-case study
- PMID: 33948701
- PMCID: PMC8523448
- DOI: 10.1007/s00330-021-07992-w
Impact of artificial intelligence support on accuracy and reading time in breast tomosynthesis image interpretation: a multi-reader multi-case study
Abstract
Objectives: Digital breast tomosynthesis (DBT) increases sensitivity of mammography and is increasingly implemented in breast cancer screening. However, the large volume of images increases the risk of reading errors and reading time. This study aims to investigate whether the accuracy of breast radiologists reading wide-angle DBT increases with the aid of an artificial intelligence (AI) support system. Also, the impact on reading time was assessed and the stand-alone performance of the AI system in the detection of malignancies was compared to the average radiologist.
Methods: A multi-reader multi-case study was performed with 240 bilateral DBT exams (71 breasts with cancer lesions, 70 breasts with benign findings, 339 normal breasts). Exams were interpreted by 18 radiologists, with and without AI support, providing cancer suspicion scores per breast. Using AI support, radiologists were shown examination-based and region-based cancer likelihood scores. Area under the receiver operating characteristic curve (AUC) and reading time per exam were compared between reading conditions using mixed-models analysis of variance.
Results: On average, the AUC was higher using AI support (0.863 vs 0.833; p = 0.0025). Using AI support, reading time per DBT exam was reduced (p < 0.001) from 41 (95% CI = 39-42 s) to 36 s (95% CI = 35- 37 s). The AUC of the stand-alone AI system was non-inferior to the AUC of the average radiologist (+0.007, p = 0.8115).
Conclusions: Radiologists improved their cancer detection and reduced reading time when evaluating DBT examinations using an AI reading support system.
Key points: • Radiologists improved their cancer detection accuracy in digital breast tomosynthesis (DBT) when using an AI system for support, while simultaneously reducing reading time. • The stand-alone breast cancer detection performance of an AI system is non-inferior to the average performance of radiologists for reading digital breast tomosynthesis exams. • The use of an AI support system could make advanced and more reliable imaging techniques more accessible and could allow for more cost-effective breast screening programs with DBT.
Keywords: Artificial intelligence (AI); Breast cancer; Digital breast tomosynthesis (DBT); Mammography; Mass screening.
© 2021. The Author(s).
Conflict of interest statement
The authors of this manuscript declare relationships with the following companies:
The AI support system under investigation (Transpara™) in this study was developed by ScreenPoint Medical (Nijmegen, The Netherlands), a spin-off company of the Department of Medical Imaging, Radboud University Medical Center. Several authors are employees of this company (Alejandro Rodriguez-Ruiz, PhD; Albert Gubern-Merida, PhD; Nico Karssemeijer, PhD). The content of this study was also used for FDA approval. All data was generated by a fully independent clinical research organization (Radboudumc; Radboud University Medical Center, Nijmegen, The Netherlands). Readers were not affiliated with ScreenPoint Medical in any way. Data was handled and controlled at all times by the non-ScreenPoint employee authors.
Figures
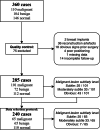
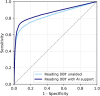
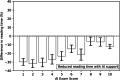
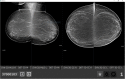
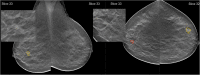
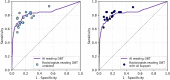
Similar articles
-
Impact of Artificial Intelligence Decision Support Using Deep Learning on Breast Cancer Screening Interpretation with Single-View Wide-Angle Digital Breast Tomosynthesis.Radiology. 2021 Sep;300(3):529-536. doi: 10.1148/radiol.2021204432. Epub 2021 Jul 6. Radiology. 2021. PMID: 34227882
-
Stand-Alone Use of Artificial Intelligence for Digital Mammography and Digital Breast Tomosynthesis Screening: A Retrospective Evaluation.Radiology. 2022 Mar;302(3):535-542. doi: 10.1148/radiol.211590. Epub 2021 Dec 14. Radiology. 2022. PMID: 34904872
-
Impact of AI for Digital Breast Tomosynthesis on Breast Cancer Detection and Interpretation Time.Radiol Artif Intell. 2024 May;6(3):e230318. doi: 10.1148/ryai.230318. Radiol Artif Intell. 2024. PMID: 38568095 Free PMC article.
-
Artificial intelligence for digital breast tomosynthesis: Impact on diagnostic performance, reading times, and workload in the era of personalized screening.Eur J Radiol. 2023 Jan;158:110631. doi: 10.1016/j.ejrad.2022.110631. Epub 2022 Dec 2. Eur J Radiol. 2023. PMID: 36481480 Review.
-
Supplemental Screening for Breast Cancer in Women With Dense Breasts: A Systematic Review for the U.S. Preventive Service Task Force [Internet].Rockville (MD): Agency for Healthcare Research and Quality (US); 2016 Jan. Report No.: 14-05201-EF-3. Rockville (MD): Agency for Healthcare Research and Quality (US); 2016 Jan. Report No.: 14-05201-EF-3. PMID: 26866210 Free Books & Documents. Review.
Cited by
-
Improving breast cancer diagnostics with deep learning for MRI.Sci Transl Med. 2022 Sep 28;14(664):eabo4802. doi: 10.1126/scitranslmed.abo4802. Epub 2022 Sep 28. Sci Transl Med. 2022. PMID: 36170446 Free PMC article.
-
Unassisted Clinicians Versus Deep Learning-Assisted Clinicians in Image-Based Cancer Diagnostics: Systematic Review With Meta-analysis.J Med Internet Res. 2023 Mar 2;25:e43832. doi: 10.2196/43832. J Med Internet Res. 2023. PMID: 36862499 Free PMC article. Review.
-
Artificial intelligence in breast cancer imaging: risk stratification, lesion detection and classification, treatment planning and prognosis-a narrative review.Explor Target Antitumor Ther. 2022;3(6):795-816. doi: 10.37349/etat.2022.00113. Epub 2022 Dec 27. Explor Target Antitumor Ther. 2022. PMID: 36654817 Free PMC article. Review.
-
Can a Computer-Aided Mass Diagnosis Model Based on Perceptive Features Learned From Quantitative Mammography Radiology Reports Improve Junior Radiologists' Diagnosis Performance? An Observer Study.Front Oncol. 2021 Dec 17;11:773389. doi: 10.3389/fonc.2021.773389. eCollection 2021. Front Oncol. 2021. PMID: 34976817 Free PMC article.
-
Impact of real-life use of artificial intelligence as support for human reading in a population-based breast cancer screening program with mammography and tomosynthesis.Eur Radiol. 2024 Jun;34(6):3958-3966. doi: 10.1007/s00330-023-10426-4. Epub 2023 Nov 17. Eur Radiol. 2024. PMID: 37975920 Free PMC article.
References
-
- Zackrisson S, Lång K, Rosso A, et al. One-view breast tomosynthesis versus two-view mammography in the Malmö Breast Tomosynthesis Screening Trial (MBTST): a prospective, population-based, diagnostic accuracy study. Lancet Oncol. 2018;19(11):1493–1503. doi: 10.1016/S1470-2045(18)30521-7. - DOI - PubMed
-
- Bernardi D, Gentilini MA, De Nisi M, et al. Effect of implementing digital breast tomosynthesis (DBT) instead of mammography on population screening outcomes including interval cancer rates: Results of the Trento DBT pilot evaluation. Breast. 2019;50:135–140. doi: 10.1016/j.breast.2019.09.012. - DOI - PMC - PubMed
MeSH terms
LinkOut - more resources
Full Text Sources
Other Literature Sources
Medical