Neural Coding in Spiking Neural Networks: A Comparative Study for Robust Neuromorphic Systems
- PMID: 33746705
- PMCID: PMC7970006
- DOI: 10.3389/fnins.2021.638474
Neural Coding in Spiking Neural Networks: A Comparative Study for Robust Neuromorphic Systems
Abstract
Various hypotheses of information representation in brain, referred to as neural codes, have been proposed to explain the information transmission between neurons. Neural coding plays an essential role in enabling the brain-inspired spiking neural networks (SNNs) to perform different tasks. To search for the best coding scheme, we performed an extensive comparative study on the impact and performance of four important neural coding schemes, namely, rate coding, time-to-first spike (TTFS) coding, phase coding, and burst coding. The comparative study was carried out using a biological 2-layer SNN trained with an unsupervised spike-timing-dependent plasticity (STDP) algorithm. Various aspects of network performance were considered, including classification accuracy, processing latency, synaptic operations (SOPs), hardware implementation, network compression efficacy, input and synaptic noise resilience, and synaptic fault tolerance. The classification tasks on Modified National Institute of Standards and Technology (MNIST) and Fashion-MNIST datasets were applied in our study. For hardware implementation, area and power consumption were estimated for these coding schemes, and the network compression efficacy was analyzed using pruning and quantization techniques. Different types of input noise and noise variations in the datasets were considered and applied. Furthermore, the robustness of each coding scheme to the non-ideality-induced synaptic noise and fault in analog neuromorphic systems was studied and compared. Our results show that TTFS coding is the best choice in achieving the highest computational performance with very low hardware implementation overhead. TTFS coding requires 4x/7.5x lower processing latency and 3.5x/6.5x fewer SOPs than rate coding during the training/inference process. Phase coding is the most resilient scheme to input noise. Burst coding offers the highest network compression efficacy and the best overall robustness to hardware non-idealities for both training and inference processes. The study presented in this paper reveals the design space created by the choice of each coding scheme, allowing designers to frame each scheme in terms of its strength and weakness given a designs' constraints and considerations in neuromorphic systems.
Keywords: burst coding; neural codes; neuromorphic computing; phase coding; rate coding; spiking neural networks; time to first spike coding; unsupervised learning.
Copyright © 2021 Guo, Fouda, Eltawil and Salama.
Conflict of interest statement
The authors declare that the research was conducted in the absence of any commercial or financial relationships that could be construed as a potential conflict of interest.
Figures
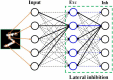
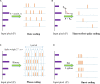
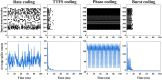
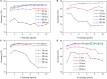
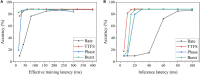
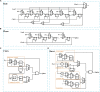
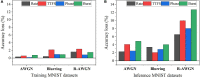
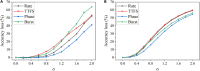
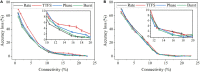
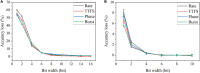
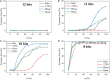
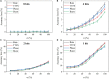
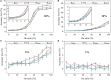
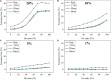
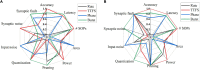
Similar articles
-
A TTFS-based energy and utilization efficient neuromorphic CNN accelerator.Front Neurosci. 2023 May 5;17:1121592. doi: 10.3389/fnins.2023.1121592. eCollection 2023. Front Neurosci. 2023. PMID: 37214405 Free PMC article.
-
SSTDP: Supervised Spike Timing Dependent Plasticity for Efficient Spiking Neural Network Training.Front Neurosci. 2021 Nov 4;15:756876. doi: 10.3389/fnins.2021.756876. eCollection 2021. Front Neurosci. 2021. PMID: 34803591 Free PMC article.
-
Unsupervised Adaptive Weight Pruning for Energy-Efficient Neuromorphic Systems.Front Neurosci. 2020 Nov 12;14:598876. doi: 10.3389/fnins.2020.598876. eCollection 2020. Front Neurosci. 2020. PMID: 33281549 Free PMC article.
-
Deep Learning With Spiking Neurons: Opportunities and Challenges.Front Neurosci. 2018 Oct 25;12:774. doi: 10.3389/fnins.2018.00774. eCollection 2018. Front Neurosci. 2018. PMID: 30410432 Free PMC article. Review.
-
Advancements in Algorithms and Neuromorphic Hardware for Spiking Neural Networks.Neural Comput. 2022 May 19;34(6):1289-1328. doi: 10.1162/neco_a_01499. Neural Comput. 2022. PMID: 35534005 Review.
Cited by
-
Direct training high-performance deep spiking neural networks: a review of theories and methods.Front Neurosci. 2024 Jul 31;18:1383844. doi: 10.3389/fnins.2024.1383844. eCollection 2024. Front Neurosci. 2024. PMID: 39145295 Free PMC article. Review.
-
Optimizing event-based neural networks on digital neuromorphic architecture: a comprehensive design space exploration.Front Neurosci. 2024 Mar 28;18:1335422. doi: 10.3389/fnins.2024.1335422. eCollection 2024. Front Neurosci. 2024. PMID: 38606307 Free PMC article.
-
Brain-inspired neural circuit evolution for spiking neural networks.Proc Natl Acad Sci U S A. 2023 Sep 26;120(39):e2218173120. doi: 10.1073/pnas.2218173120. Epub 2023 Sep 20. Proc Natl Acad Sci U S A. 2023. PMID: 37729206 Free PMC article.
-
SNN4Agents: a framework for developing energy-efficient embodied spiking neural networks for autonomous agents.Front Robot AI. 2024 Jul 26;11:1401677. doi: 10.3389/frobt.2024.1401677. eCollection 2024. Front Robot AI. 2024. PMID: 39131197 Free PMC article.
-
Learnable Leakage and Onset-Spiking Self-Attention in SNNs with Local Error Signals.Sensors (Basel). 2023 Dec 12;23(24):9781. doi: 10.3390/s23249781. Sensors (Basel). 2023. PMID: 38139626 Free PMC article.
References
-
- Basu S., Karki M., Ganguly S., DiBiano R., Mukhopadhyay S., Gayaka S., et al. (2017). Learning sparse feature representations using probabilistic quadtrees and deep belief nets. Neural Process. Lett. 45 855–867. 10.1007/s11063-016-9556-4 - DOI
LinkOut - more resources
Full Text Sources
Other Literature Sources