Excitation-Inhibition Balanced Neural Networks for Fast Signal Detection
- PMID: 33013343
- PMCID: PMC7497310
- DOI: 10.3389/fncom.2020.00079
Excitation-Inhibition Balanced Neural Networks for Fast Signal Detection
Abstract
Excitation-inhibition (E-I) balanced neural networks are a classic model for modeling neural activities and functions in the cortex. The present study investigates the potential application of E-I balanced neural networks for fast signal detection in brain-inspired computation. We first theoretically analyze the response property of an E-I balanced network, and find that the asynchronous firing state of the network generates an optimal noise structure enabling the network to track input changes rapidly. We then extend the homogeneous connectivity of an E-I balanced neural network to include local neuronal connections, so that the network can still achieve fast response and meanwhile maintain spatial information in the face of spatially heterogeneous signal. Finally, we carry out simulations to demonstrate that our model works well.
Keywords: E-I balanced network; Fokker-Planck equation; asynchronous state; fast tracking; optimal noise structure.
Copyright © 2020 Tian, Li, Huang and Wu.
Figures
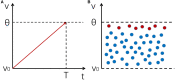
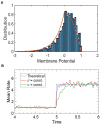
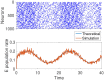
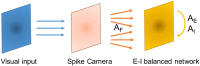
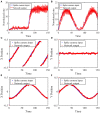
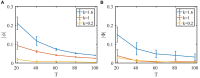
Similar articles
-
Balanced Active Core in Heterogeneous Neuronal Networks.Front Comput Neurosci. 2019 Jan 29;12:109. doi: 10.3389/fncom.2018.00109. eCollection 2018. Front Comput Neurosci. 2019. PMID: 30745868 Free PMC article.
-
Spatially extended balanced networks without translationally invariant connectivity.J Math Neurosci. 2020 May 13;10(1):8. doi: 10.1186/s13408-020-00085-w. J Math Neurosci. 2020. PMID: 32405723 Free PMC article.
-
Self-Consistent Scheme for Spike-Train Power Spectra in Heterogeneous Sparse Networks.Front Comput Neurosci. 2018 Mar 2;12:9. doi: 10.3389/fncom.2018.00009. eCollection 2018. Front Comput Neurosci. 2018. PMID: 29551968 Free PMC article.
-
Theory of the asynchronous state of structured rotator networks and its application to recurrent networks of excitatory and inhibitory units.Phys Rev E. 2023 Apr;107(4-1):044306. doi: 10.1103/PhysRevE.107.044306. Phys Rev E. 2023. PMID: 37198857
-
In Vivo Observations of Rapid Scattered Light Changes Associated with Neurophysiological Activity.In: Frostig RD, editor. In Vivo Optical Imaging of Brain Function. 2nd edition. Boca Raton (FL): CRC Press/Taylor & Francis; 2009. Chapter 5. In: Frostig RD, editor. In Vivo Optical Imaging of Brain Function. 2nd edition. Boca Raton (FL): CRC Press/Taylor & Francis; 2009. Chapter 5. PMID: 26844322 Free Books & Documents. Review.
Cited by
-
Efficient and robust coding in heterogeneous recurrent networks.PLoS Comput Biol. 2021 Apr 30;17(4):e1008673. doi: 10.1371/journal.pcbi.1008673. eCollection 2021 Apr. PLoS Comput Biol. 2021. PMID: 33930016 Free PMC article.
-
Distinctive properties of biological neural networks and recent advances in bottom-up approaches toward a better biologically plausible neural network.Front Comput Neurosci. 2023 Jun 28;17:1092185. doi: 10.3389/fncom.2023.1092185. eCollection 2023. Front Comput Neurosci. 2023. PMID: 37449083 Free PMC article. Review.
-
Dorsolateral Prefrontal Cortex Glutamate/Gamma-Aminobutyric Acid (GABA) Alterations in Clinical High Risk and First-Episode Schizophrenia: A Preliminary 7-T Magnetic Resonance Spectroscopy Imaging Study.Int J Mol Sci. 2022 Dec 13;23(24):15846. doi: 10.3390/ijms232415846. Int J Mol Sci. 2022. PMID: 36555487 Free PMC article.
-
On the Role of the Excitation/Inhibition Balance of Homeostatic Artificial Neural Networks.Entropy (Basel). 2021 Dec 14;23(12):1681. doi: 10.3390/e23121681. Entropy (Basel). 2021. PMID: 34945987 Free PMC article.
-
A disinhibitory circuit mechanism explains a general principle of peak performance during mid-level arousal.Proc Natl Acad Sci U S A. 2024 Jan 30;121(5):e2312898121. doi: 10.1073/pnas.2312898121. Epub 2024 Jan 26. Proc Natl Acad Sci U S A. 2024. PMID: 38277436 Free PMC article.
References
-
- Barrett D. G. T. (2012). Computation in balanced networks (Ph.D. thesis). University College London, London, United Kingdom.
LinkOut - more resources
Full Text Sources