Biological batch normalisation: How intrinsic plasticity improves learning in deep neural networks
- PMID: 32966302
- PMCID: PMC7511202
- DOI: 10.1371/journal.pone.0238454
Biological batch normalisation: How intrinsic plasticity improves learning in deep neural networks
Abstract
In this work, we present a local intrinsic rule that we developed, dubbed IP, inspired by the Infomax rule. Like Infomax, this rule works by controlling the gain and bias of a neuron to regulate its rate of fire. We discuss the biological plausibility of the IP rule and compare it to batch normalisation. We demonstrate that the IP rule improves learning in deep networks, and provides networks with considerable robustness to increases in synaptic learning rates. We also sample the error gradients during learning and show that the IP rule substantially increases the size of the gradients over the course of learning. This suggests that the IP rule solves the vanishing gradient problem. Supplementary analysis is provided to derive the equilibrium solutions that the neuronal gain and bias converge to using our IP rule. An analysis demonstrates that the IP rule results in neuronal information potential similar to that of Infomax, when tested on a fixed input distribution. We also show that batch normalisation also improves information potential, suggesting that this may be a cause for the efficacy of batch normalisation-an open problem at the time of this writing.
Conflict of interest statement
The authors have declared that no competing interests exist.
Figures
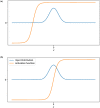
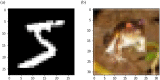
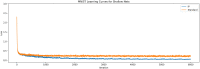
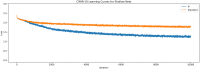
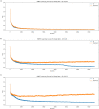
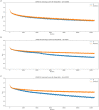
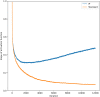
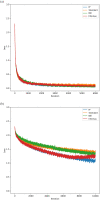
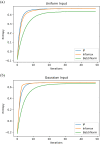
Similar articles
-
Learning in neural networks by reinforcement of irregular spiking.Phys Rev E Stat Nonlin Soft Matter Phys. 2004 Apr;69(4 Pt 1):041909. doi: 10.1103/PhysRevE.69.041909. Epub 2004 Apr 30. Phys Rev E Stat Nonlin Soft Matter Phys. 2004. PMID: 15169045
-
Synergies between intrinsic and synaptic plasticity based on information theoretic learning.PLoS One. 2013 May 9;8(5):e62894. doi: 10.1371/journal.pone.0062894. Print 2013. PLoS One. 2013. PMID: 23671642 Free PMC article.
-
Reinforcement Learning in Spiking Neural Networks with Stochastic and Deterministic Synapses.Neural Comput. 2019 Dec;31(12):2368-2389. doi: 10.1162/neco_a_01238. Epub 2019 Oct 15. Neural Comput. 2019. PMID: 31614099
-
A review of learning in biologically plausible spiking neural networks.Neural Netw. 2020 Feb;122:253-272. doi: 10.1016/j.neunet.2019.09.036. Epub 2019 Oct 11. Neural Netw. 2020. PMID: 31726331 Review.
-
How synapses in the auditory system wax and wane: theoretical perspectives.Biol Cybern. 2003 Nov;89(5):318-32. doi: 10.1007/s00422-003-0437-3. Epub 2003 Nov 28. Biol Cybern. 2003. PMID: 14669012 Review.
Cited by
-
Distinctive properties of biological neural networks and recent advances in bottom-up approaches toward a better biologically plausible neural network.Front Comput Neurosci. 2023 Jun 28;17:1092185. doi: 10.3389/fncom.2023.1092185. eCollection 2023. Front Comput Neurosci. 2023. PMID: 37449083 Free PMC article. Review.
-
Intrinsic threshold plasticity: cholinergic activation and role in the neuronal recognition of incomplete input patterns.J Physiol. 2023 Aug;601(15):3221-3239. doi: 10.1113/JP283473. Epub 2022 Aug 11. J Physiol. 2023. PMID: 35879872 Free PMC article.
-
Automatic segmentation of lower limb muscles from MR images of post-menopausal women based on deep learning and data augmentation.PLoS One. 2024 Apr 2;19(4):e0299099. doi: 10.1371/journal.pone.0299099. eCollection 2024. PLoS One. 2024. PMID: 38564618 Free PMC article.
References
-
- Hebb DO. “The organization of behavior: A neuropsychological theory.” Psychology Press; (2005) April 11.
-
- Werbos PJ. “Applications of advances in nonlinear sensitivity analysis” In System modeling and optimization (1982) (pp. 762–770). Springer, Berlin, Heidelberg.
-
- Shannon CE, Weaver W. “The mathematical theory of communication.” University of Illinois press; (1998) September 1.
MeSH terms
Grants and funding
LinkOut - more resources
Full Text Sources