AD risk score for the early phases of disease based on unsupervised machine learning
- PMID: 32729964
- PMCID: PMC7666001
- DOI: 10.1002/alz.12140
AD risk score for the early phases of disease based on unsupervised machine learning
Abstract
Introduction: Identifying cognitively normal individuals at high risk for progression to symptomatic Alzheimer's disease (AD) is critical for early intervention.
Methods: An AD risk score was derived using unsupervised machine learning. The score was developed using data from 226 cognitively normal individuals and included cerebrospinal fluid, magnetic resonance imaging, and cognitive measures, and validated in an independent cohort.
Results: Higher baseline AD progression risk scores (hazard ratio = 2.70, P < 0.001) were associated with greater risks of progression to clinical symptoms of mild cognitive impairment (MCI). Baseline scores had an area under the curve of 0.83 (95% confidence interval: 0.75 to 0.91) for identifying subjects who progressed to MCI/dementia within 5 years. The validation procedure, using data from the Alzheimer's Disease Neuroimaging Initiative, demonstrated accuracy of prediction across the AD spectrum.
Discussion: The derived risk score provides high predictive accuracy for identifying which individuals with normal cognition are likely to show clinical decline due to AD within 5 years.
Keywords: Alzheimer's disease; cognitive testing; latent variable; machine learning; multidomain biomarkers; progression; risk score; unsupervised learning.
© 2020 the Alzheimer's Association.
Conflict of interest statement
Figures
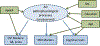
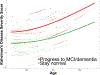
Similar articles
-
A parameter-efficient deep learning approach to predict conversion from mild cognitive impairment to Alzheimer's disease.Neuroimage. 2019 Apr 1;189:276-287. doi: 10.1016/j.neuroimage.2019.01.031. Epub 2019 Jan 14. Neuroimage. 2019. PMID: 30654174
-
Optimizing Machine Learning Methods to Improve Predictive Models of Alzheimer's Disease.J Alzheimers Dis. 2019;71(3):1027-1036. doi: 10.3233/JAD-190262. J Alzheimers Dis. 2019. PMID: 31476152 Free PMC article.
-
A Machine Learning-Based Holistic Approach to Predict the Clinical Course of Patients within the Alzheimer's Disease Spectrum.J Alzheimers Dis. 2022;85(4):1639-1655. doi: 10.3233/JAD-210573. J Alzheimers Dis. 2022. PMID: 34958014
-
Potential neuroimaging biomarkers of pathologic brain changes in Mild Cognitive Impairment and Alzheimer's disease: a systematic review.BMC Geriatr. 2016 May 16;16:104. doi: 10.1186/s12877-016-0281-7. BMC Geriatr. 2016. PMID: 27184250 Free PMC article. Review.
-
2014 Update of the Alzheimer's Disease Neuroimaging Initiative: A review of papers published since its inception.Alzheimers Dement. 2015 Jun;11(6):e1-120. doi: 10.1016/j.jalz.2014.11.001. Alzheimers Dement. 2015. PMID: 26073027 Free PMC article. Review.
Cited by
-
Machine learning-based clustering in cervical spondylotic myelopathy patients to identify heterogeneous clinical characteristics.Front Surg. 2022 Jul 25;9:935656. doi: 10.3389/fsurg.2022.935656. eCollection 2022. Front Surg. 2022. PMID: 35959114 Free PMC article.
-
Machine Learning Approach Predicts Probability of Time to Stage-Specific Conversion of Alzheimer's Disease.J Alzheimers Dis. 2022;90(2):891-903. doi: 10.3233/JAD-220590. J Alzheimers Dis. 2022. PMID: 36189595 Free PMC article.
-
Construction of a novel lower-extremity peripheral artery disease subtype prediction model using unsupervised machine learning and neutrophil-related biomarkers.Heliyon. 2024 Jan 6;10(2):e24189. doi: 10.1016/j.heliyon.2024.e24189. eCollection 2024 Jan 30. Heliyon. 2024. PMID: 38293541 Free PMC article.
-
Identification of spinal tuberculosis subphenotypes using routine clinical data: a study based on unsupervised machine learning.Ann Med. 2023;55(2):2249004. doi: 10.1080/07853890.2023.2249004. Ann Med. 2023. PMID: 37611242 Free PMC article.
-
Few-shot prediction of amyloid β accumulation from mainly unpaired data on biomarker candidates.NPJ Syst Biol Appl. 2023 Nov 23;9(1):59. doi: 10.1038/s41540-023-00321-5. NPJ Syst Biol Appl. 2023. PMID: 37993458 Free PMC article.
References
-
- Sperling RA, Aisen PS, Beckett LA, Bennett DA, Craft S, Fagan AM, Iwatsubo T, Jack CR Jr, Kaye J, Montine TJ and Park DC, 2011. Toward defining the preclinical stages of Alzheimer’s disease: Recommendations from the National Institute on Aging-Alzheimer’s Association workgroups on diagnostic guidelines for Alzheimer’s disease. Alzheimer’s & dementia, 7(3), pp.280–292. - PMC - PubMed
-
- Gauthier S, Albert M, Fox N, Goedert M, Kivipelto M, Mestre-Ferrandiz J and Middleton LT, 2016. Why has therapy development for dementia failed in the last two decades?. Alzheimer’s & Dementia, 12(1), pp.60–64. - PubMed
-
- Jack CR Jr, Knopman DS, Jagust WJ, Petersen RC, Weiner MW, Aisen PS, Shaw LM, Vemuri P, Wiste HJ, Weigand SD and Lesnick TG, 2013. Tracking pathophysiological processes in Alzheimer’s disease: an updated hypothetical model of dynamic biomarkers. The Lancet Neurology, 12(2), pp.207–216. - PMC - PubMed
-
- De Meyer G, Shapiro F, Vanderstichele H, Vanmechelen E, Engelborghs S, De Deyn PP, Coart E, Hansson O, Minthon L, Zetterberg H and Blennow K, 2010. Diagnosis-independent Alzheimer disease biomarker signature in cognitively normal elderly people. Archives of neurology, 67(8), pp.949–956. - PMC - PubMed
-
- Zhou XH, McClish DK and Obuchowski NA, 2009. Statistical methods in diagnostic medicine (Vol. 569). John Wiley & Sons.
Publication types
MeSH terms
Grants and funding
LinkOut - more resources
Full Text Sources
Medical