BETA: A Large Benchmark Database Toward SSVEP-BCI Application
- PMID: 32655358
- PMCID: PMC7324867
- DOI: 10.3389/fnins.2020.00627
BETA: A Large Benchmark Database Toward SSVEP-BCI Application
Abstract
The brain-computer interface (BCI) provides an alternative means to communicate and it has sparked growing interest in the past two decades. Specifically, for Steady-State Visual Evoked Potential (SSVEP) based BCI, marked improvement has been made in the frequency recognition method and data sharing. However, the number of pubic databases is still limited in this field. Therefore, we present a BEnchmark database Towards BCI Application (BETA) in the study. The BETA database is composed of 64-channel Electroencephalogram (EEG) data of 70 subjects performing a 40-target cued-spelling task. The design and the acquisition of the BETA are in pursuit of meeting the demand from real-world applications and it can be used as a test-bed for these scenarios. We validate the database by a series of analyses and conduct the classification analysis of eleven frequency recognition methods on BETA. We recommend using the metric of wide-band signal-to-noise ratio (SNR) and BCI quotient to characterize the SSVEP at the single-trial and population levels, respectively. The BETA database can be downloaded from the following link http://bci.med.tsinghua.edu.cn/download.html.
Keywords: brain-computer interface (BCI); classification algorithms; electroencephalogram (EEG); frequency recognition; public database; signal-to-noise ratio (SNR); steady-state visual evoked potential (SSVEP).
Copyright © 2020 Liu, Huang, Wang, Chen and Gao.
Figures
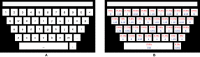
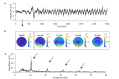
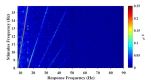
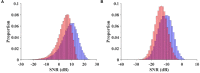
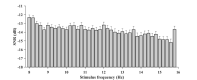
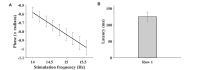
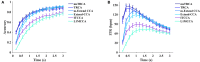
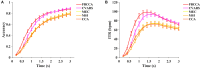
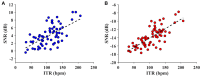
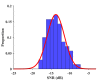
Similar articles
-
eldBETA: A Large Eldercare-oriented Benchmark Database of SSVEP-BCI for the Aging Population.Sci Data. 2022 May 31;9(1):252. doi: 10.1038/s41597-022-01372-9. Sci Data. 2022. PMID: 35641547 Free PMC article.
-
A Benchmark Dataset for SSVEP-Based Brain-Computer Interfaces.IEEE Trans Neural Syst Rehabil Eng. 2017 Oct;25(10):1746-1752. doi: 10.1109/TNSRE.2016.2627556. Epub 2016 Nov 10. IEEE Trans Neural Syst Rehabil Eng. 2017. PMID: 27849543
-
Implementing a calibration-free SSVEP-based BCI system with 160 targets.J Neural Eng. 2021 Jul 2;18(4). doi: 10.1088/1741-2552/ac0bfa. J Neural Eng. 2021. PMID: 34134091
-
Brain-Computer Interface Speller Based on Steady-State Visual Evoked Potential: A Review Focusing on the Stimulus Paradigm and Performance.Brain Sci. 2021 Apr 1;11(4):450. doi: 10.3390/brainsci11040450. Brain Sci. 2021. PMID: 33916189 Free PMC article. Review.
-
A Systematic Review of Using Deep Learning Technology in the Steady-State Visually Evoked Potential-Based Brain-Computer Interface Applications: Current Trends and Future Trust Methodology.Int J Telemed Appl. 2023 Apr 30;2023:7741735. doi: 10.1155/2023/7741735. eCollection 2023. Int J Telemed Appl. 2023. PMID: 37168809 Free PMC article. Review.
Cited by
-
An open dataset for human SSVEPs in the frequency range of 1-60 Hz.Sci Data. 2024 Feb 13;11(1):196. doi: 10.1038/s41597-024-03023-7. Sci Data. 2024. PMID: 38351064 Free PMC article.
-
Frequency set selection for multi-frequency steady-state visual evoked potential-based brain-computer interfaces.Front Neurosci. 2022 Dec 21;16:1057010. doi: 10.3389/fnins.2022.1057010. eCollection 2022. Front Neurosci. 2022. PMID: 36620442 Free PMC article.
-
Multi-frequency steady-state visual evoked potential dataset.Sci Data. 2024 Jan 4;11(1):26. doi: 10.1038/s41597-023-02841-5. Sci Data. 2024. PMID: 38177151 Free PMC article.
-
An Analysis of Deep Learning Models in SSVEP-Based BCI: A Survey.Brain Sci. 2023 Mar 13;13(3):483. doi: 10.3390/brainsci13030483. Brain Sci. 2023. PMID: 36979293 Free PMC article. Review.
-
SSVEP detection assessment by combining visual stimuli paradigms and no-training detection methods.Front Neurosci. 2023 May 18;17:1142892. doi: 10.3389/fnins.2023.1142892. eCollection 2023. Front Neurosci. 2023. PMID: 37274188 Free PMC article.
References
-
- Ang K. K., Guan C. (2013). Brain-computer interface in stroke rehabilitation. J. Comput. Sci. Eng. 7, 139–146. 10.5626/JCSE.2013.7.2.139 - DOI
LinkOut - more resources
Full Text Sources
Miscellaneous