Spectral Vegetation Indices to Track Senescence Dynamics in Diverse Wheat Germplasm
- PMID: 32047504
- PMCID: PMC6997566
- DOI: 10.3389/fpls.2019.01749
Spectral Vegetation Indices to Track Senescence Dynamics in Diverse Wheat Germplasm
Abstract
The ability of a genotype to stay green affects the primary target traits grain yield (GY) and grain protein concentration (GPC) in wheat. High throughput methods to assess senescence dynamics in large field trials will allow for (i) indirect selection in early breeding generations, when yield cannot yet be accurately determined and (ii) mapping of the genomic regions controlling the trait. The aim of this study was to develop a robust method to assess senescence based on hyperspectral canopy reflectance. Measurements were taken in three years throughout the grain filling phase on >300 winter wheat varieties in the spectral range from 350 to 2500 nm using a spectroradiometer. We compared the potential of spectral indices (SI) and full-spectrum models to infer visually observed senescence dynamics from repeated reflectance measurements. Parameters describing the dynamics of senescence were used to predict GY and GPC and a feature selection algorithm was used to identify the most predictive features. The three-band plant senescence reflectance index (PSRI) approximated the visually observed senescence dynamics best, whereas full-spectrum models suffered from a strong year-specificity. Feature selection identified visual scorings as most predictive for GY, but also PSRI ranked among the most predictive features while adding additional spectral features had little effect. Visually scored delayed senescence was positively correlated with GY ranging from r = 0.173 in 2018 to r = 0.365 in 2016. It appears that visual scoring remains the gold standard to quantify leaf senescence in moderately large trials. However, using appropriate phenotyping platforms, the proposed index-based parameterization of the canopy reflectance dynamics offers the critical advantage of upscaling to very large breeding trials.
Keywords: canopy reflectance; feature selection; field-based phenotyping; high-throughput phenotyping; hyperspectral remote sensing.
Copyright © 2020 Anderegg, Yu, Aasen, Walter, Liebisch and Hund.
Figures
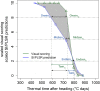
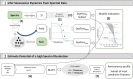
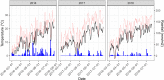

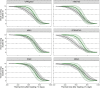
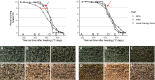
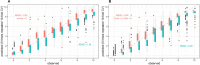
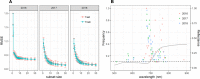
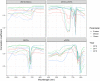
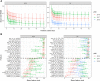
Similar articles
-
Predicting grain protein content of field-grown winter wheat with satellite images and partial least square algorithm.PLoS One. 2020 Mar 11;15(3):e0228500. doi: 10.1371/journal.pone.0228500. eCollection 2020. PLoS One. 2020. PMID: 32160185 Free PMC article.
-
High-Throughput Field Phenotyping Traits of Grain Yield Formation and Nitrogen Use Efficiency: Optimizing the Selection of Vegetation Indices and Growth Stages.Front Plant Sci. 2020 Jan 17;10:1672. doi: 10.3389/fpls.2019.01672. eCollection 2019. Front Plant Sci. 2020. PMID: 32010159 Free PMC article.
-
In-Field Detection and Quantification of Septoria Tritici Blotch in Diverse Wheat Germplasm Using Spectral-Temporal Features.Front Plant Sci. 2019 Oct 25;10:1355. doi: 10.3389/fpls.2019.01355. eCollection 2019. Front Plant Sci. 2019. PMID: 31708956 Free PMC article.
-
Identification of stay-green and early senescence phenotypes in high-yielding winter wheat, and their relationship to grain yield and grain protein concentration using high-throughput phenotyping techniques.Funct Plant Biol. 2014 Feb;41(3):227-235. doi: 10.1071/FP13221. Funct Plant Biol. 2014. PMID: 32480983
-
Applications of a Hyperspectral Imaging System Used to Estimate Wheat Grain Protein: A Review.Front Plant Sci. 2022 Apr 8;13:837200. doi: 10.3389/fpls.2022.837200. eCollection 2022. Front Plant Sci. 2022. PMID: 35463397 Free PMC article. Review.
Cited by
-
PhenoCams for Field Phenotyping: Using Very High Temporal Resolution Digital Repeated Photography to Investigate Interactions of Growth, Phenology, and Harvest Traits.Front Plant Sci. 2020 Jun 18;11:593. doi: 10.3389/fpls.2020.00593. eCollection 2020. Front Plant Sci. 2020. PMID: 32625216 Free PMC article.
-
To What Extent Does Yellow Rust Infestation Affect Remotely Sensed Nitrogen Status?Plant Phenomics. 2023 Sep 6;5:0083. doi: 10.34133/plantphenomics.0083. eCollection 2023. Plant Phenomics. 2023. PMID: 37681000 Free PMC article.
-
Characterizing stay-green in barley across diverse environments: unveiling novel haplotypes.Theor Appl Genet. 2024 May 6;137(6):120. doi: 10.1007/s00122-024-04612-1. Theor Appl Genet. 2024. PMID: 38709310 Free PMC article.
-
The spatial variability of NDVI within a wheat field: Information content and implications for yield and grain protein monitoring.PLoS One. 2022 Mar 22;17(3):e0265243. doi: 10.1371/journal.pone.0265243. eCollection 2022. PLoS One. 2022. PMID: 35316290 Free PMC article.
-
Image-based phenomic prediction can provide valuable decision support in wheat breeding.Theor Appl Genet. 2023 Jun 27;136(7):162. doi: 10.1007/s00122-023-04395-x. Theor Appl Genet. 2023. PMID: 37368140 Free PMC article.
References
-
- Aasen H., Bolten A. (2018). Multi-temporal high-resolution imaging spectroscopy with hyperspectral 2D imagers – From theory to application. Remote Sens. Environ. 205, 374–389. 10.1016/j.rse.2017.10.043 - DOI
-
- Aasen H., Honkavaara E., Lucieer A., Zarco-Tejada P. J. (2018). Quantitative remote sensing at ultra-high resolution with uav spectroscopy: a review of sensor technology, measurement procedures, and data correction workflows. Remote Sens. 10, 1091. 10.3390/rs10071091 - DOI
-
- Asrar G., Fuchs M., Kanemasu E. T., Hatfield J. L. (1984). Estimating absorbed photosynthetic radiation and leaf area index from spectral reflectance in Wheat 1. Agron. J. 76, 300–306. 10.2134/agronj1984.00021962007600020029x - DOI
LinkOut - more resources
Full Text Sources