Image-Based Cardiac Diagnosis With Machine Learning: A Review
- PMID: 32039241
- PMCID: PMC6992607
- DOI: 10.3389/fcvm.2020.00001
Image-Based Cardiac Diagnosis With Machine Learning: A Review
Abstract
Cardiac imaging plays an important role in the diagnosis of cardiovascular disease (CVD). Until now, its role has been limited to visual and quantitative assessment of cardiac structure and function. However, with the advent of big data and machine learning, new opportunities are emerging to build artificial intelligence tools that will directly assist the clinician in the diagnosis of CVDs. This paper presents a thorough review of recent works in this field and provide the reader with a detailed presentation of the machine learning methods that can be further exploited to enable more automated, precise and early diagnosis of most CVDs.
Keywords: artificial intelligence; automated diagnosis; cardiac imaging; cardiovascular disease; deep learning; machine learning; radiomics.
Copyright © 2020 Martin-Isla, Campello, Izquierdo, Raisi-Estabragh, Baeßler, Petersen and Lekadir.
Figures
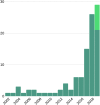
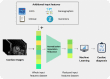
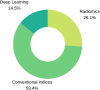
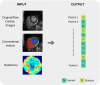
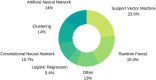
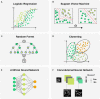
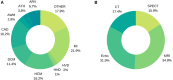
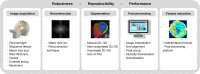
Similar articles
-
Cardiac imaging: working towards fully-automated machine analysis & interpretation.Expert Rev Med Devices. 2017 Mar;14(3):197-212. doi: 10.1080/17434440.2017.1300057. Expert Rev Med Devices. 2017. PMID: 28277804 Free PMC article. Review.
-
Radiomics in Echocardiography: Deep Learning and Echocardiographic Analysis.Curr Cardiol Rep. 2020 Jul 9;22(9):89. doi: 10.1007/s11886-020-01348-4. Curr Cardiol Rep. 2020. PMID: 32648059 Review.
-
Machine Meets Biology: a Primer on Artificial Intelligence in Cardiology and Cardiac Imaging.Curr Cardiol Rep. 2018 Oct 18;20(12):139. doi: 10.1007/s11886-018-1074-8. Curr Cardiol Rep. 2018. PMID: 30334108 Review.
-
Artificial intelligence, machine (deep) learning and radio(geno)mics: definitions and nuclear medicine imaging applications.Eur J Nucl Med Mol Imaging. 2019 Dec;46(13):2630-2637. doi: 10.1007/s00259-019-04373-w. Epub 2019 Jul 6. Eur J Nucl Med Mol Imaging. 2019. PMID: 31280350 Review.
-
Artificial intelligence and radiomics in nuclear medicine: potentials and challenges.Eur J Nucl Med Mol Imaging. 2019 Dec;46(13):2731-2736. doi: 10.1007/s00259-019-04593-0. Eur J Nucl Med Mol Imaging. 2019. PMID: 31673788
Cited by
-
Machine learning phenotyping of scarred myocardium from cine in hypertrophic cardiomyopathy.Eur Heart J Cardiovasc Imaging. 2022 Mar 22;23(4):532-542. doi: 10.1093/ehjci/jeab056. Eur Heart J Cardiovasc Imaging. 2022. PMID: 33779725 Free PMC article.
-
Rethinking Breast Cancer Diagnosis through Deep Learning Based Image Recognition.Sensors (Basel). 2023 Feb 19;23(4):2307. doi: 10.3390/s23042307. Sensors (Basel). 2023. PMID: 36850906 Free PMC article.
-
Shallow and deep learning classifiers in medical image analysis.Eur Radiol Exp. 2024 Mar 5;8(1):26. doi: 10.1186/s41747-024-00428-2. Eur Radiol Exp. 2024. PMID: 38438821 Free PMC article. Review.
-
Real-World and Regulatory Perspectives of Artificial Intelligence in Cardiovascular Imaging.Front Cardiovasc Med. 2022 Jul 22;9:890809. doi: 10.3389/fcvm.2022.890809. eCollection 2022. Front Cardiovasc Med. 2022. PMID: 35935648 Free PMC article.
-
Predicting left ventricular hypertrophy from the 12-lead electrocardiogram in the UK Biobank imaging study using machine learning.Eur Heart J Digit Health. 2023 Jun 1;4(4):316-324. doi: 10.1093/ehjdh/ztad037. eCollection 2023 Aug. Eur Heart J Digit Health. 2023. PMID: 37538142 Free PMC article.
References
-
- Wilkins E, Wilson L, Wickramasinghe K, Bhatnagar P, Leal J, Luengo-Fernandez R, et al. . European Cardiovascular Disease Statistics 2017. Brussels: European Heart Network; (2017).
-
- Ritchie H, Roser M. Our world in data. In: Causes of Death (2018). Retrieved from: https://ourworldindata.org/causes-of-death.
-
- Khened M, Alex V, Krishnamurthi G. Densely connected fully convolutional network for short-axis cardiac cine MR image segmentation and heart diagnosis using random forest. In: International Workshop on Statistical Atlases and Computational Models of the Heart. Quebec City, QC: Springer; (2017). p. 140–51.
Publication types
Grants and funding
LinkOut - more resources
Full Text Sources