Deep learning interpretation of echocardiograms
- PMID: 31993508
- PMCID: PMC6981156
- DOI: 10.1038/s41746-019-0216-8
Deep learning interpretation of echocardiograms
Abstract
Echocardiography uses ultrasound technology to capture high temporal and spatial resolution images of the heart and surrounding structures, and is the most common imaging modality in cardiovascular medicine. Using convolutional neural networks on a large new dataset, we show that deep learning applied to echocardiography can identify local cardiac structures, estimate cardiac function, and predict systemic phenotypes that modify cardiovascular risk but not readily identifiable to human interpretation. Our deep learning model, EchoNet, accurately identified the presence of pacemaker leads (AUC = 0.89), enlarged left atrium (AUC = 0.86), left ventricular hypertrophy (AUC = 0.75), left ventricular end systolic and diastolic volumes ( = 0.74 and = 0.70), and ejection fraction ( = 0.50), as well as predicted systemic phenotypes of age ( = 0.46), sex (AUC = 0.88), weight ( = 0.56), and height ( = 0.33). Interpretation analysis validates that EchoNet shows appropriate attention to key cardiac structures when performing human-explainable tasks and highlights hypothesis-generating regions of interest when predicting systemic phenotypes difficult for human interpretation. Machine learning on echocardiography images can streamline repetitive tasks in the clinical workflow, provide preliminary interpretation in areas with insufficient qualified cardiologists, and predict phenotypes challenging for human evaluation.
Keywords: Cardiovascular diseases; Image processing; Machine learning.
© The Author(s) 2020.
Conflict of interest statement
Competing InterestsThe authors declare no competing interests.
Figures
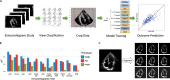
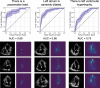
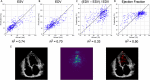
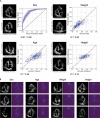
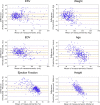
Similar articles
-
Automated interpretation of systolic and diastolic function on the echocardiogram: a multicohort study.Lancet Digit Health. 2022 Jan;4(1):e46-e54. doi: 10.1016/S2589-7500(21)00235-1. Epub 2021 Dec 1. Lancet Digit Health. 2022. PMID: 34863649
-
Fully Automated Echocardiogram Interpretation in Clinical Practice.Circulation. 2018 Oct 16;138(16):1623-1635. doi: 10.1161/CIRCULATIONAHA.118.034338. Circulation. 2018. PMID: 30354459 Free PMC article.
-
Deep learning evaluation of biomarkers from echocardiogram videos.EBioMedicine. 2021 Nov;73:103613. doi: 10.1016/j.ebiom.2021.103613. Epub 2021 Oct 14. EBioMedicine. 2021. PMID: 34656880 Free PMC article.
-
European Association of Cardiovascular Imaging/Cardiovascular Imaging Department of the Brazilian Society of Cardiology recommendations for the use of cardiac imaging to assess and follow patients after heart transplantation.Eur Heart J Cardiovasc Imaging. 2015 Sep;16(9):919-48. doi: 10.1093/ehjci/jev139. Epub 2015 Jul 2. Eur Heart J Cardiovasc Imaging. 2015. PMID: 26139361 Review.
-
Machine Learning Augmented Echocardiography for Diastolic Function Assessment.Front Cardiovasc Med. 2021 Aug 4;8:711611. doi: 10.3389/fcvm.2021.711611. eCollection 2021. Front Cardiovasc Med. 2021. PMID: 34422935 Free PMC article. Review.
Cited by
-
Prediction of Coronary Artery Calcium Using Deep Learning of Echocardiograms.J Am Soc Echocardiogr. 2023 May;36(5):474-481.e3. doi: 10.1016/j.echo.2022.12.014. Epub 2022 Dec 23. J Am Soc Echocardiogr. 2023. PMID: 36566995 Free PMC article.
-
Severe aortic stenosis detection by deep learning applied to echocardiography.Eur Heart J. 2023 Nov 14;44(43):4592-4604. doi: 10.1093/eurheartj/ehad456. Eur Heart J. 2023. PMID: 37611002 Free PMC article.
-
How AI drives innovation in cardiovascular medicine.Front Cardiovasc Med. 2024 Apr 26;11:1397921. doi: 10.3389/fcvm.2024.1397921. eCollection 2024. Front Cardiovasc Med. 2024. PMID: 38737711 Free PMC article.
-
Echo2Pheno: a deep-learning application to uncover echocardiographic phenotypes in conscious mice.Mamm Genome. 2023 Jun;34(2):200-215. doi: 10.1007/s00335-023-09996-x. Epub 2023 May 23. Mamm Genome. 2023. PMID: 37221250 Free PMC article.
-
Hybrid deep spatial and statistical feature fusion for accurate MRI brain tumor classification.Front Comput Neurosci. 2024 Jun 24;18:1423051. doi: 10.3389/fncom.2024.1423051. eCollection 2024. Front Comput Neurosci. 2024. PMID: 38978524 Free PMC article.
References
-
- Madani A, Ong JR, Tiberwal A, Mofrad MR. US hospital use of echocardiography: Insights from the nationwide inpatient sample. J. Am. Coll. Cardiol. 2016;67:502–511. - PubMed
Grants and funding
LinkOut - more resources
Full Text Sources
Other Literature Sources