Distributed coding of choice, action and engagement across the mouse brain
- PMID: 31776518
- PMCID: PMC6913580
- DOI: 10.1038/s41586-019-1787-x
Distributed coding of choice, action and engagement across the mouse brain
Abstract
Vision, choice, action and behavioural engagement arise from neuronal activity that may be distributed across brain regions. Here we delineate the spatial distribution of neurons underlying these processes. We used Neuropixels probes1,2 to record from approximately 30,000 neurons in 42 brain regions of mice performing a visual discrimination task3. Neurons in nearly all regions responded non-specifically when the mouse initiated an action. By contrast, neurons encoding visual stimuli and upcoming choices occupied restricted regions in the neocortex, basal ganglia and midbrain. Choice signals were rare and emerged with indistinguishable timing across regions. Midbrain neurons were activated before contralateral choices and were suppressed before ipsilateral choices, whereas forebrain neurons could prefer either side. Brain-wide pre-stimulus activity predicted engagement in individual trials and in the overall task, with enhanced subcortical but suppressed neocortical activity during engagement. These results reveal organizing principles for the distribution of neurons encoding behaviourally relevant variables across the mouse brain.
Conflict of interest statement
The authors declare no competing interests.
Figures
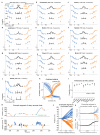
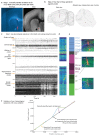
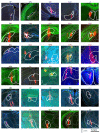
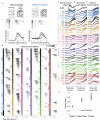
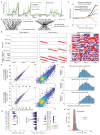
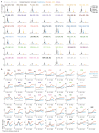
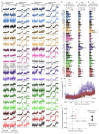
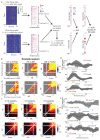
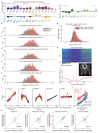
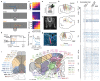
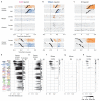
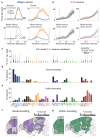
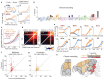
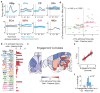
Similar articles
-
Brain-wide neural activity underlying memory-guided movement.Cell. 2024 Feb 1;187(3):676-691.e16. doi: 10.1016/j.cell.2023.12.035. Cell. 2024. PMID: 38306983
-
Responses of monkey dopamine neurons to reward and conditioned stimuli during successive steps of learning a delayed response task.J Neurosci. 1993 Mar;13(3):900-13. doi: 10.1523/JNEUROSCI.13-03-00900.1993. J Neurosci. 1993. PMID: 8441015 Free PMC article.
-
Evaluating Visual Cues Modulates Their Representation in Mouse Visual and Cingulate Cortex.J Neurosci. 2021 Apr 14;41(15):3531-3544. doi: 10.1523/JNEUROSCI.1828-20.2021. Epub 2021 Mar 9. J Neurosci. 2021. PMID: 33687964 Free PMC article.
-
Multiple Mechanisms for Processing Reward Uncertainty in the Primate Basal Forebrain.J Neurosci. 2016 Jul 27;36(30):7852-64. doi: 10.1523/JNEUROSCI.1123-16.2016. J Neurosci. 2016. PMID: 27466331 Free PMC article.
-
Subjective neuronal coding of reward: temporal value discounting and risk.Eur J Neurosci. 2010 Jun;31(12):2124-35. doi: 10.1111/j.1460-9568.2010.07282.x. Epub 2010 May 24. Eur J Neurosci. 2010. PMID: 20497474 Review.
Cited by
-
Neural dynamics of causal inference in the macaque frontoparietal circuit.Elife. 2022 Oct 24;11:e76145. doi: 10.7554/eLife.76145. Elife. 2022. PMID: 36279158 Free PMC article.
-
ACC neural ensemble dynamics are structured by strategy prevalence.Elife. 2023 Nov 22;12:e84897. doi: 10.7554/eLife.84897. Elife. 2023. PMID: 37991007 Free PMC article.
-
Triple dissociation of visual, auditory and motor processing in mouse primary visual cortex.Nat Neurosci. 2024 Apr;27(4):758-771. doi: 10.1038/s41593-023-01564-5. Epub 2024 Feb 2. Nat Neurosci. 2024. PMID: 38307971
-
To be and not to be: wide-field Ca2+ imaging reveals neocortical functional segmentation combines stability and flexibility.Cereb Cortex. 2023 May 24;33(11):6543-6558. doi: 10.1093/cercor/bhac523. Cereb Cortex. 2023. PMID: 36734268 Free PMC article.
-
Mice alternate between discrete strategies during perceptual decision-making.Nat Neurosci. 2022 Feb;25(2):201-212. doi: 10.1038/s41593-021-01007-z. Epub 2022 Feb 7. Nat Neurosci. 2022. PMID: 35132235 Free PMC article.
References
Publication types
MeSH terms
Grants and funding
LinkOut - more resources
Full Text Sources
Molecular Biology Databases