Deep Learning Convolutional Neural Networks for the Estimation of Liver Fibrosis Severity from Ultrasound Texture
- PMID: 31741550
- PMCID: PMC6859455
- DOI: 10.1117/12.2512592
Deep Learning Convolutional Neural Networks for the Estimation of Liver Fibrosis Severity from Ultrasound Texture
Abstract
Diagnosis and staging of liver fibrosis is a vital prognostic marker in chronic liver diseases. Due to the inaccuracies and risk of complications associated with liver core needle biopsy, the current standard for diagnosis, other less invasive methods are sought for diagnosis. One such method that has been shown to correlate well with liver fibrosis is shear wave velocity measured by ultrasound (US) shear wave elastography; however, this technique requires specific software, hardware, and training. A current perspective in the radiology community is that the texture pattern from an US image may be predictive of the stage of liver fibrosis. We propose the use of convolutional neural networks (CNNs), a framework shown to be well suited for real world image interpretation, to test whether the texture pattern in gray scale elastography images (B-mode US with fixed, subject-agnostic acquisition settings) is predictive of the shear wave velocity (SWV). In this study, gray scale elastography images from over 300 patients including 3,500 images with corresponding SWV measurements were preprocessed and used as input to 100 different CNN architectures that were trained to regress shear wave velocity. In this study, even the best performing CNN explained only negligible variation in the shear wave velocity measures. These extensive test results suggest that the gray scale elastography image texture provides little predictive information about shear wave velocity and liver fibrosis.
Keywords: Convolutional Neural Network; Deep Learning; Liver Fibrosis; Random Search; Shear Wave Velocity; Ultrasound.
Figures
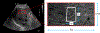
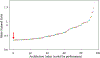
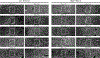
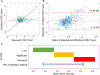
Similar articles
-
Ultrasound Shear Wave Elastography for Liver Disease. A Critical Appraisal of the Many Actors on the Stage.Ultraschall Med. 2016 Feb;37(1):1-5. doi: 10.1055/s-0035-1567037. Epub 2016 Feb 12. Ultraschall Med. 2016. PMID: 26871407
-
Temporal stability assessment in shear wave elasticity images validated by deep learning neural network for chronic liver disease fibrosis stage assessment.Med Phys. 2019 May;46(5):2298-2309. doi: 10.1002/mp.13521. Epub 2019 Apr 15. Med Phys. 2019. PMID: 30929260
-
Shear wave trajectory detection in ultra-fast M-mode images for liver fibrosis assessment: A deep learning-based line detection approach.Ultrasonics. 2024 Aug;142:107358. doi: 10.1016/j.ultras.2024.107358. Epub 2024 Jun 10. Ultrasonics. 2024. PMID: 38901149
-
Shear wave liver elastography.Abdom Radiol (NY). 2018 Apr;43(4):800-807. doi: 10.1007/s00261-017-1375-1. Abdom Radiol (NY). 2018. PMID: 29116341 Review.
-
[Ultrasound elastography].Radiologe. 2015 Nov;55(11):949-55. doi: 10.1007/s00117-015-0028-z. Radiologe. 2015. PMID: 26438090 Review. German.
Cited by
-
Viscoelastic parameter estimation using simulated shear wave motion and convolutional neural networks.Comput Biol Med. 2021 Jun;133:104382. doi: 10.1016/j.compbiomed.2021.104382. Epub 2021 Apr 11. Comput Biol Med. 2021. PMID: 33872971 Free PMC article.
-
A Hierarchical Siamese Network for Noninvasive Staging of Liver Fibrosis Based on US Image Pairs of the Liver and Spleen.Sensors (Basel). 2023 Jun 8;23(12):5450. doi: 10.3390/s23125450. Sensors (Basel). 2023. PMID: 37420617 Free PMC article.
-
Non-invasive precise staging of liver fibrosis using deep residual network model based on plain CT images.Int J Comput Assist Radiol Surg. 2022 Apr;17(4):627-637. doi: 10.1007/s11548-022-02573-8. Epub 2022 Feb 22. Int J Comput Assist Radiol Surg. 2022. PMID: 35194737
-
Current status of deep learning applications in abdominal ultrasonography.Ultrasonography. 2021 Apr;40(2):177-182. doi: 10.14366/usg.20085. Epub 2020 Sep 2. Ultrasonography. 2021. PMID: 33242931 Free PMC article.
-
An Overview of Artificial Intelligence Applications in Liver and Pancreatic Imaging.Cancers (Basel). 2021 Apr 30;13(9):2162. doi: 10.3390/cancers13092162. Cancers (Basel). 2021. PMID: 33946223 Free PMC article.
References
-
- Centers for Disease Control and Prevention. Chronic Liver Disease and Cirrhosis. Available at https://www.cdc.gov/nchs/fastats/liver-disease.htm (2016).
-
- Barr RG et al. Elastography Assessment of Liver Fibrosis: Society of Radiologists in Ultrasound Consensus Conference Statement. Radiology 276, 845–861 (2015). - PubMed
-
- Regev A et al. Sampling error and intraobserver variation in liver biopsy in patients with chronic HCV infection. The American journal of gastroenterology 97, 2614–2618 (2002). - PubMed
-
- Vicas C, Lupsor M, Socaciu M, Badea R & Nedevschi Sergiu. Liver Fibrosis detection by the means of texture analysis. Limitations and further development directions. Automat. Comput. Appl. Math 19, 397–402 (2010).
Grants and funding
LinkOut - more resources
Full Text Sources