Data augmentation using generative adversarial networks (CycleGAN) to improve generalizability in CT segmentation tasks
- PMID: 31729403
- PMCID: PMC6858365
- DOI: 10.1038/s41598-019-52737-x
Data augmentation using generative adversarial networks (CycleGAN) to improve generalizability in CT segmentation tasks
Abstract
Labeled medical imaging data is scarce and expensive to generate. To achieve generalizable deep learning models large amounts of data are needed. Standard data augmentation is a method to increase generalizability and is routinely performed. Generative adversarial networks offer a novel method for data augmentation. We evaluate the use of CycleGAN for data augmentation in CT segmentation tasks. Using a large image database we trained a CycleGAN to transform contrast CT images into non-contrast images. We then used the trained CycleGAN to augment our training using these synthetic non-contrast images. We compared the segmentation performance of a U-Net trained on the original dataset compared to a U-Net trained on the combined dataset of original data and synthetic non-contrast images. We further evaluated the U-Net segmentation performance on two separate datasets: The original contrast CT dataset on which segmentations were created and a second dataset from a different hospital containing only non-contrast CTs. We refer to these 2 separate datasets as the in-distribution and out-of-distribution datasets, respectively. We show that in several CT segmentation tasks performance is improved significantly, especially in out-of-distribution (noncontrast CT) data. For example, when training the model with standard augmentation techniques, performance of segmentation of the kidneys on out-of-distribution non-contrast images was dramatically lower than for in-distribution data (Dice score of 0.09 vs. 0.94 for out-of-distribution vs. in-distribution data, respectively, p < 0.001). When the kidney model was trained with CycleGAN augmentation techniques, the out-of-distribution (non-contrast) performance increased dramatically (from a Dice score of 0.09 to 0.66, p < 0.001). Improvements for the liver and spleen were smaller, from 0.86 to 0.89 and 0.65 to 0.69, respectively. We believe this method will be valuable to medical imaging researchers to reduce manual segmentation effort and cost in CT imaging.
Conflict of interest statement
Author RMS reports receiving royalties from iCAD, Philips, PingAn and ScanMed, and his lab receives research support from PingAn (Cooperative Research and Development Agreement) and NVIDIA (GPU card donation). Author PJP reports being an advisor to Bracco and a shareholder in SHINE, Elucent, and Cellectar. Author VS reports no competing interests. Author KY reports no competing interests.
Figures
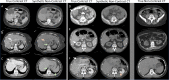
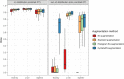
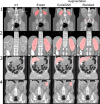
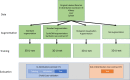
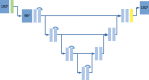
Similar articles
-
Anatomy-constrained synthesis for spleen segmentation improvement in unpaired mouse micro-CT scans with 3D CycleGAN.Biomed Phys Eng Express. 2024 Aug 8;10(5). doi: 10.1088/2057-1976/ad6a63. Biomed Phys Eng Express. 2024. PMID: 39094603
-
A conventional-to-spectral CT image translation augmentation workflow for robust contrast injection-independent organ segmentation.Med Phys. 2022 Feb;49(2):1108-1122. doi: 10.1002/mp.15310. Epub 2021 Dec 20. Med Phys. 2022. PMID: 34689353
-
CT2US: Cross-modal transfer learning for kidney segmentation in ultrasound images with synthesized data.Ultrasonics. 2022 May;122:106706. doi: 10.1016/j.ultras.2022.106706. Epub 2022 Feb 7. Ultrasonics. 2022. PMID: 35149255
-
Automated vessel segmentation in lung CT and CTA images via deep neural networks.J Xray Sci Technol. 2021;29(6):1123-1137. doi: 10.3233/XST-210955. J Xray Sci Technol. 2021. PMID: 34421004 Review.
-
Automatic Segmentation of Multiple Organs on 3D CT Images by Using Deep Learning Approaches.Adv Exp Med Biol. 2020;1213:135-147. doi: 10.1007/978-3-030-33128-3_9. Adv Exp Med Biol. 2020. PMID: 32030668 Review.
Cited by
-
[Development of an Optimized Deep Learning Model for Medical Imaging].Taehan Yongsang Uihakhoe Chi. 2020 Nov;81(6):1274-1289. doi: 10.3348/jksr.2020.0171. Epub 2020 Nov 30. Taehan Yongsang Uihakhoe Chi. 2020. PMID: 36237706 Free PMC article. Review. Korean.
-
Denoising Multiphase Functional Cardiac CT Angiography Using Deep Learning and Synthetic Data.Radiol Artif Intell. 2024 Mar;6(2):e230153. doi: 10.1148/ryai.230153. Radiol Artif Intell. 2024. PMID: 38416035 Free PMC article.
-
The Challenge of Data Annotation in Deep Learning-A Case Study on Whole Plant Corn Silage.Sensors (Basel). 2022 Feb 18;22(4):1596. doi: 10.3390/s22041596. Sensors (Basel). 2022. PMID: 35214497 Free PMC article.
-
Artifact-free fat-water separation in Dixon MRI using deep learning.J Big Data. 2023;10(1):4. doi: 10.1186/s40537-022-00677-1. Epub 2023 Jan 12. J Big Data. 2023. PMID: 36686622 Free PMC article.
-
Advancing COVID-19 Diagnosis with Privacy-Preserving Collaboration in Artificial Intelligence.ArXiv [Preprint]. 2021 Nov 18:arXiv:2111.09461v1. ArXiv. 2021. Update in: Nat Mach Intell. 2022;4(4):413. doi: 10.1038/s42256-022-00485-5 PMID: 34815983 Free PMC article. Updated. Preprint.
References
-
- Moreno-Torres JG, Raeder T, Alaiz-RodríGuez R, Chawla NV, Herrera F. A unifying view on dataset shift in classification. Pattern Recogn. 2012;45:521–530. doi: 10.1016/j.patcog.2011.06.019. - DOI
-
- Goodfellow, I. et al. Generative adversarial nets. In Ghahramani, Z., Welling, M., Cortes, C., Lawrence, N. D. & Weinberger, K. Q. (eds) Advances in Neural Information Processing Systems 27, 2672–2680 (Curran Associates, Inc., 2014).
-
- Antoniou, A., Storkey, A. & Edwards, H. Data augmentation generative adversarial networks, arXiv:1711.04340 (2017).
-
- Shrivastava, A. et al. Learning from simulated and unsupervised images through adversarial training. In 2017 IEEE Conference on Computer Vision and Pattern Recognition (CVPR), 2242–2251, 10.1109/CVPR.2017.241 (2017).
Publication types
MeSH terms
LinkOut - more resources
Full Text Sources
Other Literature Sources
Medical
Research Materials