Assessment of Accuracy of an Artificial Intelligence Algorithm to Detect Melanoma in Images of Skin Lesions
- PMID: 31617929
- PMCID: PMC6806667
- DOI: 10.1001/jamanetworkopen.2019.13436
Assessment of Accuracy of an Artificial Intelligence Algorithm to Detect Melanoma in Images of Skin Lesions
Erratum in
-
Error in Key Points.JAMA Netw Open. 2019 Nov 1;2(11):e1916430. doi: 10.1001/jamanetworkopen.2019.16430. JAMA Netw Open. 2019. PMID: 31693116 Free PMC article. No abstract available.
Abstract
Importance: A high proportion of suspicious pigmented skin lesions referred for investigation are benign. Techniques to improve the accuracy of melanoma diagnoses throughout the patient pathway are needed to reduce the pressure on secondary care and pathology services.
Objective: To determine the accuracy of an artificial intelligence algorithm in identifying melanoma in dermoscopic images of lesions taken with smartphone and digital single-lens reflex (DSLR) cameras.
Design, setting, and participants: This prospective, multicenter, single-arm, masked diagnostic trial took place in dermatology and plastic surgery clinics in 7 UK hospitals. Dermoscopic images of suspicious and control skin lesions from 514 patients with at least 1 suspicious pigmented skin lesion scheduled for biopsy were captured on 3 different cameras. Data were collected from January 2017 to July 2018. Clinicians and the Deep Ensemble for Recognition of Malignancy, a deterministic artificial intelligence algorithm trained to identify melanoma in dermoscopic images of pigmented skin lesions using deep learning techniques, assessed the likelihood of melanoma. Initial data analysis was conducted in September 2018; further analysis was conducted from February 2019 to August 2019.
Interventions: Clinician and algorithmic assessment of melanoma.
Main outcomes and measures: Area under the receiver operating characteristic curve (AUROC), sensitivity, and specificity of the algorithmic and specialist assessment, determined using histopathology diagnosis as the criterion standard.
Results: The study population of 514 patients included 279 women (55.7%) and 484 white patients (96.8%), with a mean (SD) age of 52.1 (18.6) years. A total of 1550 images of skin lesions were included in the analysis (551 [35.6%] biopsied lesions; 999 [64.4%] control lesions); 286 images (18.6%) were used to train the algorithm, and a further 849 (54.8%) images were missing or unsuitable for analysis. Of the biopsied lesions that were assessed by the algorithm and specialists, 125 (22.7%) were diagnosed as melanoma. Of these, 77 (16.7%) were used for the primary analysis. The algorithm achieved an AUROC of 90.1% (95% CI, 86.3%-94.0%) for biopsied lesions and 95.8% (95% CI, 94.1%-97.6%) for all lesions using iPhone 6s images; an AUROC of 85.8% (95% CI, 81.0%-90.7%) for biopsied lesions and 93.8% (95% CI, 91.4%-96.2%) for all lesions using Galaxy S6 images; and an AUROC of 86.9% (95% CI, 80.8%-93.0%) for biopsied lesions and 91.8% (95% CI, 87.5%-96.1%) for all lesions using DSLR camera images. At 100% sensitivity, the algorithm achieved a specificity of 64.8% with iPhone 6s images. Specialists achieved an AUROC of 77.8% (95% CI, 72.5%-81.9%) and a specificity of 69.9%.
Conclusions and relevance: In this study, the algorithm demonstrated an ability to identify melanoma from dermoscopic images of selected lesions with an accuracy similar to that of specialists.
Conflict of interest statement
Figures
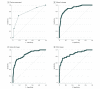
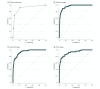
Similar articles
-
Dermoscopy, with and without visual inspection, for diagnosing melanoma in adults.Cochrane Database Syst Rev. 2018 Dec 4;12(12):CD011902. doi: 10.1002/14651858.CD011902.pub2. Cochrane Database Syst Rev. 2018. PMID: 30521682 Free PMC article.
-
Evaluation of an artificial intelligence-based decision support for the detection of cutaneous melanoma in primary care: a prospective real-life clinical trial.Br J Dermatol. 2024 Jun 20;191(1):125-133. doi: 10.1093/bjd/ljae021. Br J Dermatol. 2024. PMID: 38234043 Clinical Trial.
-
Computer-assisted diagnosis techniques (dermoscopy and spectroscopy-based) for diagnosing skin cancer in adults.Cochrane Database Syst Rev. 2018 Dec 4;12(12):CD013186. doi: 10.1002/14651858.CD013186. Cochrane Database Syst Rev. 2018. PMID: 30521691 Free PMC article.
-
Role of In Vivo Reflectance Confocal Microscopy in the Analysis of Melanocytic Lesions.Acta Dermatovenerol Croat. 2018 Apr;26(1):64-67. Acta Dermatovenerol Croat. 2018. PMID: 29782304 Review.
-
Digital image analysis for diagnosis of cutaneous melanoma. Development of a highly effective computer algorithm based on analysis of 837 melanocytic lesions.Br J Dermatol. 2004 Nov;151(5):1029-38. doi: 10.1111/j.1365-2133.2004.06210.x. Br J Dermatol. 2004. PMID: 15541081 Review.
Cited by
-
Slit lamp polarized dermoscopy: a cost-effective tool to assess eyelid lesions.Int Ophthalmol. 2023 Apr;43(4):1103-1110. doi: 10.1007/s10792-022-02505-0. Epub 2022 Sep 9. Int Ophthalmol. 2023. PMID: 36083562
-
Issues in Melanoma Detection: Semisupervised Deep Learning Algorithm Development via a Combination of Human and Artificial Intelligence.JMIR Dermatol. 2022 Dec 12;5(4):e39113. doi: 10.2196/39113. JMIR Dermatol. 2022. PMID: 37632881 Free PMC article.
-
Augmenting the accuracy of trainee doctors in diagnosing skin lesions suspected of skin neoplasms in a real-world setting: A prospective controlled before-and-after study.PLoS One. 2022 Jan 21;17(1):e0260895. doi: 10.1371/journal.pone.0260895. eCollection 2022. PLoS One. 2022. PMID: 35061692 Free PMC article.
-
Analysis of Artificial Intelligence-Based Approaches Applied to Non-Invasive Imaging for Early Detection of Melanoma: A Systematic Review.Cancers (Basel). 2023 Sep 23;15(19):4694. doi: 10.3390/cancers15194694. Cancers (Basel). 2023. PMID: 37835388 Free PMC article. Review.
-
FACES: A Deep-Learning-Based Parametric Model to Improve Rosacea Diagnoses.Appl Sci (Basel). 2023 Jan 2;13(2):970. doi: 10.3390/app13020970. Epub 2023 Jan 11. Appl Sci (Basel). 2023. PMID: 38282829 Free PMC article.
References
-
- Cancer Research UK . Melanoma skin cancer survival statistics. https://www.cancerresearchuk.org/health-professional/cancer-statistics/s.... Accessed March 21, 2019.
-
- Wernli KJ, Henrikson NB, Morrison CC, Nguyen M, Pocobelli G, Whitlock EP; US Preventive Services Task Force Evidence Syntheses formerly Systematic Evidence Reviews . Screening for skin cancer in adults: an updated systematic evidence review for the US Preventive Services Task Force. JAMA. 2016;316(4):-. - PubMed
-
- National Institute for Health and Care Excellence . Melanoma: assessment and management. https://www.nice.org.uk/guidance/ng14. Accessed September 5, 2019.
Publication types
MeSH terms
LinkOut - more resources
Full Text Sources
Medical