Training dynamically balanced excitatory-inhibitory networks
- PMID: 31393909
- PMCID: PMC6687153
- DOI: 10.1371/journal.pone.0220547
Training dynamically balanced excitatory-inhibitory networks
Abstract
The construction of biologically plausible models of neural circuits is crucial for understanding the computational properties of the nervous system. Constructing functional networks composed of separate excitatory and inhibitory neurons obeying Dale's law presents a number of challenges. We show how a target-based approach, when combined with a fast online constrained optimization technique, is capable of building functional models of rate and spiking recurrent neural networks in which excitation and inhibition are balanced. Balanced networks can be trained to produce complicated temporal patterns and to solve input-output tasks while retaining biologically desirable features such as Dale's law and response variability.
Conflict of interest statement
The authors have declared that no competing interests exist.
Figures
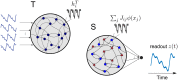
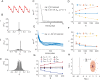
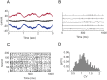
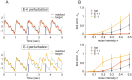
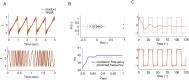
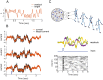
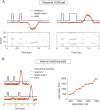
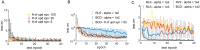
Similar articles
-
Approximating Nonlinear Functions With Latent Boundaries in Low-Rank Excitatory-Inhibitory Spiking Networks.Neural Comput. 2024 Apr 23;36(5):803-857. doi: 10.1162/neco_a_01658. Neural Comput. 2024. PMID: 38658028
-
Functional Implications of Dale's Law in Balanced Neuronal Network Dynamics and Decision Making.Front Neurosci. 2022 Feb 28;16:801847. doi: 10.3389/fnins.2022.801847. eCollection 2022. Front Neurosci. 2022. PMID: 35295091 Free PMC article.
-
Inhibitory interneurons decorrelate excitatory cells to drive sparse code formation in a spiking model of V1.J Neurosci. 2013 Mar 27;33(13):5475-85. doi: 10.1523/JNEUROSCI.4188-12.2013. J Neurosci. 2013. PMID: 23536063 Free PMC article.
-
Inhibitory stabilization and cortical computation.Nat Rev Neurosci. 2021 Jan;22(1):21-37. doi: 10.1038/s41583-020-00390-z. Epub 2020 Nov 11. Nat Rev Neurosci. 2021. PMID: 33177630 Review.
-
From artificial neural networks to spiking neuron populations and back again.Neural Netw. 2001 Jul-Sep;14(6-7):941-53. doi: 10.1016/s0893-6080(01)00068-5. Neural Netw. 2001. PMID: 11665784 Review.
Cited by
-
Geometry of population activity in spiking networks with low-rank structure.PLoS Comput Biol. 2023 Aug 7;19(8):e1011315. doi: 10.1371/journal.pcbi.1011315. eCollection 2023 Aug. PLoS Comput Biol. 2023. PMID: 37549194 Free PMC article.
-
New insights into binocular rivalry from the reconstruction of evolving percepts using model network dynamics.Front Comput Neurosci. 2023 Mar 24;17:1137015. doi: 10.3389/fncom.2023.1137015. eCollection 2023. Front Comput Neurosci. 2023. PMID: 37034441 Free PMC article.
-
Spiking Recurrent Neural Networks Represent Task-Relevant Neural Sequences in Rule-Dependent Computation.Cognit Comput. 2023 Jul;15(4):1167-1189. doi: 10.1007/s12559-022-09994-2. Epub 2022 Feb 5. Cognit Comput. 2023. PMID: 37771569 Free PMC article.
-
Distinctive properties of biological neural networks and recent advances in bottom-up approaches toward a better biologically plausible neural network.Front Comput Neurosci. 2023 Jun 28;17:1092185. doi: 10.3389/fncom.2023.1092185. eCollection 2023. Front Comput Neurosci. 2023. PMID: 37449083 Free PMC article. Review.
-
Distributing task-related neural activity across a cortical network through task-independent connections.Nat Commun. 2023 May 18;14(1):2851. doi: 10.1038/s41467-023-38529-y. Nat Commun. 2023. PMID: 37202424 Free PMC article.
References
-
- Kadmon J, Sompolinsky H. Transition to Chaos in Random Neuronal Networks. Phys Rev X. 2015;5:041030.
Publication types
MeSH terms
Grants and funding
LinkOut - more resources
Full Text Sources
Other Literature Sources