Deep echocardiography: data-efficient supervised and semi-supervised deep learning towards automated diagnosis of cardiac disease
- PMID: 31304338
- PMCID: PMC6550282
- DOI: 10.1038/s41746-018-0065-x
Deep echocardiography: data-efficient supervised and semi-supervised deep learning towards automated diagnosis of cardiac disease
Abstract
Deep learning and computer vision algorithms can deliver highly accurate and automated interpretation of medical imaging to augment and assist clinicians. However, medical imaging presents uniquely pertinent obstacles such as a lack of accessible data or a high-cost of annotation. To address this, we developed data-efficient deep learning classifiers for prediction tasks in cardiology. Using pipeline supervised models to focus relevant structures, we achieve an accuracy of 94.4% for 15-view still-image echocardiographic view classification and 91.2% accuracy for binary left ventricular hypertrophy classification. We then develop semi-supervised generative adversarial network models that can learn from both labeled and unlabeled data in a generalizable fashion. We achieve greater than 80% accuracy in view classification with only 4% of labeled data used in solely supervised techniques and achieve 92.3% accuracy for left ventricular hypertrophy classification. In exploring trade-offs between model type, resolution, data resources, and performance, we present a comprehensive analysis and improvements of efficient deep learning solutions for medical imaging assessment especially in cardiology.
Keywords: Echocardiography; Heart failure.
Conflict of interest statement
Competing interestsThe Authors declare no Competing Interests.
Figures
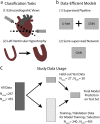
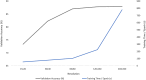
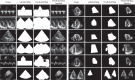
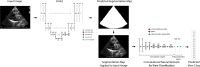
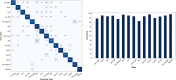

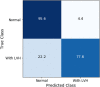
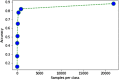
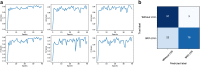
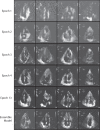
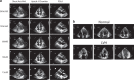
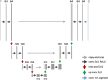
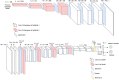
Similar articles
-
Deep virtual adversarial self-training with consistency regularization for semi-supervised medical image classification.Med Image Anal. 2021 May;70:102010. doi: 10.1016/j.media.2021.102010. Epub 2021 Feb 22. Med Image Anal. 2021. PMID: 33677262
-
Semi Supervised Learning with Deep Embedded Clustering for Image Classification and Segmentation.IEEE Access. 2019;7:11093-11104. doi: 10.1109/ACCESS.2019.2891970. Epub 2019 Jan 9. IEEE Access. 2019. PMID: 31588387 Free PMC article.
-
Semi-supervised adversarial model for benign-malignant lung nodule classification on chest CT.Med Image Anal. 2019 Oct;57:237-248. doi: 10.1016/j.media.2019.07.004. Epub 2019 Jul 10. Med Image Anal. 2019. PMID: 31352126
-
Model performance and interpretability of semi-supervised generative adversarial networks to predict oncogenic variants with unlabeled data.BMC Bioinformatics. 2023 Feb 9;24(1):43. doi: 10.1186/s12859-023-05141-2. BMC Bioinformatics. 2023. PMID: 36759776 Free PMC article. Review.
-
Self-supervised learning for medical image classification: a systematic review and implementation guidelines.NPJ Digit Med. 2023 Apr 26;6(1):74. doi: 10.1038/s41746-023-00811-0. NPJ Digit Med. 2023. PMID: 37100953 Free PMC article. Review.
Cited by
-
Deep Learning Algorithm for Automated Cardiac Murmur Detection via a Digital Stethoscope Platform.J Am Heart Assoc. 2021 May 4;10(9):e019905. doi: 10.1161/JAHA.120.019905. Epub 2021 Apr 26. J Am Heart Assoc. 2021. PMID: 33899504 Free PMC article.
-
Routine Echocardiography and Artificial Intelligence Solutions.Front Cardiovasc Med. 2021 Feb 23;8:648877. doi: 10.3389/fcvm.2021.648877. eCollection 2021. Front Cardiovasc Med. 2021. PMID: 33708808 Free PMC article. Review.
-
Artificial Intelligence-Enhanced Echocardiography for Systolic Function Assessment.J Clin Med. 2022 May 20;11(10):2893. doi: 10.3390/jcm11102893. J Clin Med. 2022. PMID: 35629019 Free PMC article. Review.
-
Innovations in Genomics and Big Data Analytics for Personalized Medicine and Health Care: A Review.Int J Mol Sci. 2022 Apr 22;23(9):4645. doi: 10.3390/ijms23094645. Int J Mol Sci. 2022. PMID: 35563034 Free PMC article. Review.
-
Deep Learning for Echocardiography: Introduction for Clinicians and Future Vision: State-of-the-Art Review.Life (Basel). 2023 Apr 17;13(4):1029. doi: 10.3390/life13041029. Life (Basel). 2023. PMID: 37109558 Free PMC article. Review.
References
-
- Stoitsis J, et al. Computer aided diagnosis based on medical image processing and artificial intelligence methods. Nucl. Instrum. Methods Phys. Res. Sect. A Accel. Spectrometers, Detect. Assoc. Equip. 2006;569:591–595. doi: 10.1016/j.nima.2006.08.134. - DOI
-
- Krizhevsky A, Sutskever I, Geoffrey E. H. ImageNet Classification with Deep Convolutiona lNeural Networks. Adv. Neural Inf. Process. Syst. 2012;25:1–9.
-
- Srivastava N, Hinton G, Krizhevsky A, Sutskever I, Salakhutdinov R. Dropout: A Simple Way to Prevent Neural Networks from Overfitting. J. Mach. Learn. Res. 2014;15:1929–1958.
LinkOut - more resources
Full Text Sources
Other Literature Sources