Differentially synchronized spiking enables multiplexed neural coding
- PMID: 31028148
- PMCID: PMC6525513
- DOI: 10.1073/pnas.1812171116
Differentially synchronized spiking enables multiplexed neural coding
Abstract
Multiplexing refers to the simultaneous encoding of two or more signals. Neurons have been shown to multiplex, but different stimuli require different multiplexing strategies. Whereas the frequency and amplitude of periodic stimuli can be encoded by the timing and rate of the same spikes, natural scenes, which comprise areas over which intensity varies gradually and sparse edges where intensity changes abruptly, require a different multiplexing strategy. Recording in vivo from neurons in primary somatosensory cortex during tactile stimulation, we found that stimulus onset and offset (edges) evoked highly synchronized spiking, whereas other spikes in the same neurons occurred asynchronously. Stimulus intensity modulated the rate of asynchronous spiking, but did not affect the timing of synchronous spikes. From this, we hypothesized that spikes driven by high- and low-contrast stimulus features can be distinguished on the basis of their synchronization, and that differentially synchronized spiking can thus be used to form multiplexed representations. Applying a Bayesian decoding method, we verified that information about high- and low-contrast features can be recovered from an ensemble of model neurons receiving common input. Equally good decoding was achieved by distinguishing synchronous from asynchronous spikes and applying reverse correlation methods separately to each spike type. This result, which we verified with patch clamp recordings in vitro, demonstrates that neurons receiving common input can use the rate of asynchronous spiking to encode the intensity of low-contrast features while using the timing of synchronous spikes to encode the occurrence of high-contrast features. We refer to this strategy as synchrony-division multiplexing.
Keywords: multiplexing; neural coding; rate code; synchrony; temporal code.
Copyright © 2019 the Author(s). Published by PNAS.
Conflict of interest statement
The authors declare no conflict of interest.
Figures
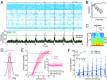
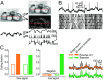
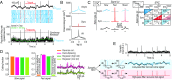
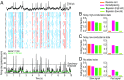
Similar articles
-
Synchrony-Division Neural Multiplexing: An Encoding Model.Entropy (Basel). 2023 Mar 30;25(4):589. doi: 10.3390/e25040589. Entropy (Basel). 2023. PMID: 37190377 Free PMC article.
-
Physiological noise facilitates multiplexed coding of vibrotactile-like signals in somatosensory cortex.Proc Natl Acad Sci U S A. 2022 Sep 13;119(37):e2118163119. doi: 10.1073/pnas.2118163119. Epub 2022 Sep 6. Proc Natl Acad Sci U S A. 2022. PMID: 36067307 Free PMC article.
-
High-velocity stimulation evokes "dense" population response in layer 2/3 vibrissal cortex.J Neurophysiol. 2017 Mar 1;117(3):1218-1228. doi: 10.1152/jn.00815.2016. Epub 2016 Dec 21. J Neurophysiol. 2017. PMID: 28003414 Free PMC article.
-
Multielectrode Recordings in the Somatosensory System.In: Nicolelis MAL, editor. Methods for Neural Ensemble Recordings. 2nd edition. Boca Raton (FL): CRC Press/Taylor & Francis; 2008. Chapter 6. In: Nicolelis MAL, editor. Methods for Neural Ensemble Recordings. 2nd edition. Boca Raton (FL): CRC Press/Taylor & Francis; 2008. Chapter 6. PMID: 21204443 Free Books & Documents. Review.
-
Population coding in somatosensory cortex.Curr Opin Neurobiol. 2002 Aug;12(4):441-7. doi: 10.1016/s0959-4388(02)00338-0. Curr Opin Neurobiol. 2002. PMID: 12139993 Review.
Cited by
-
Orientation processing by synaptic integration across first-order tactile neurons.PLoS Comput Biol. 2020 Dec 2;16(12):e1008303. doi: 10.1371/journal.pcbi.1008303. eCollection 2020 Dec. PLoS Comput Biol. 2020. PMID: 33264287 Free PMC article.
-
Basic Properties of Coordinated Neuronal Ensembles in the Auditory Thalamus.J Neurosci. 2024 May 8;44(19):e1729232024. doi: 10.1523/JNEUROSCI.1729-23.2024. J Neurosci. 2024. PMID: 38561224 Free PMC article.
-
Global and local neuronal coding of tactile information in the barrel cortex.Front Neurosci. 2024 Jan 5;17:1291864. doi: 10.3389/fnins.2023.1291864. eCollection 2023. Front Neurosci. 2024. PMID: 38249584 Free PMC article.
-
Encoding of Vibrotactile Stimuli by Mechanoreceptors in Rodent Glabrous Skin.J Neurosci. 2024 Nov 13;44(46):e1252242024. doi: 10.1523/JNEUROSCI.1252-24.2024. J Neurosci. 2024. PMID: 39379153
-
Single-neuron bursts encode pathological oscillations in subcortical nuclei of patients with Parkinson's disease and essential tremor.Proc Natl Acad Sci U S A. 2022 Aug 30;119(35):e2205881119. doi: 10.1073/pnas.2205881119. Epub 2022 Aug 26. Proc Natl Acad Sci U S A. 2022. PMID: 36018837 Free PMC article.
References
-
- Lathi BP, Ding Z. Modern Digital and Analog Communication Systems. 4th Ed Oxford Univ Press; New York: 2009.
-
- Zuo Y, et al. Complementary contributions of spike timing and spike rate to perceptual decisions in rat S1 and S2 cortex. Curr Biol. 2015;25:357–363. - PubMed
-
- Blumhagen F, et al. Neuronal filtering of multiplexed odour representations. Nature. 2011;479:493–498. - PubMed
Publication types
MeSH terms
Grants and funding
LinkOut - more resources
Full Text Sources