Predicting time to dementia using a quantitative template of disease progression
- PMID: 30859120
- PMCID: PMC6396328
- DOI: 10.1016/j.dadm.2019.01.005
Predicting time to dementia using a quantitative template of disease progression
Abstract
Introduction: Characterization of longitudinal trajectories of biomarkers implicated in sporadic Alzheimer's disease (AD) in decades before clinical diagnosis is important for disease prevention and monitoring.
Methods: We used a multivariate Bayesian model to temporally align 1369 Alzheimer's disease Neuroimaging Initiative participants based on the similarity of their longitudinal biomarker measures and estimated a quantitative template of the temporal evolution of cerebrospinal fluid A , p- , and t-tau and hippocampal volume, brain glucose metabolism, and cognitive measurements. We computed biomarker trajectories as a function of time to AD dementia and predicted AD dementia onset age in a disjoint sample.
Results: Quantitative template showed early changes in verbal memory, cerebrospinal fluid Aβ1-42 and p-tau181p, and hippocampal volume. Mean error in predicted AD dementia onset age was years.
Discussion: Our method provides a quantitative approach for characterizing the natural history of AD starting at preclinical stages despite the lack of individual-level longitudinal data spanning the entire disease timeline.
Keywords: Alzheimer; Biomarkers; Cognition; Dementia; Kaplan-Meier; Longitudinal; Onset; Prediction; Quantitative template.
Figures
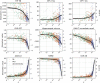
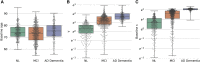
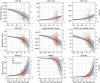
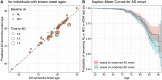
Similar articles
-
Biomarker-based prediction of progression in MCI: Comparison of AD signature and hippocampal volume with spinal fluid amyloid-β and tau.Front Aging Neurosci. 2013 Oct 11;5:55. doi: 10.3389/fnagi.2013.00055. eCollection 2013. Front Aging Neurosci. 2013. PMID: 24130528 Free PMC article.
-
Longitudinal structural cerebral changes related to core CSF biomarkers in preclinical Alzheimer's disease: A study of two independent datasets.Neuroimage Clin. 2018 Apr 16;19:190-201. doi: 10.1016/j.nicl.2018.04.016. eCollection 2018. Neuroimage Clin. 2018. PMID: 30023169 Free PMC article.
-
Clinical indications for analysis of Alzheimer's disease CSF biomarkers.Rev Neurol (Paris). 2013 Oct;169(10):709-14. doi: 10.1016/j.neurol.2013.07.024. Epub 2013 Sep 6. Rev Neurol (Paris). 2013. PMID: 24016466 Review.
-
Cerebrospinal Fluid P-Tau181P: Biomarker for Improved Differential Dementia Diagnosis.Front Neurol. 2015 Jun 17;6:138. doi: 10.3389/fneur.2015.00138. eCollection 2015. Front Neurol. 2015. PMID: 26136723 Free PMC article.
-
Understanding disease progression and improving Alzheimer's disease clinical trials: Recent highlights from the Alzheimer's Disease Neuroimaging Initiative.Alzheimers Dement. 2019 Jan;15(1):106-152. doi: 10.1016/j.jalz.2018.08.005. Epub 2018 Oct 13. Alzheimers Dement. 2019. PMID: 30321505 Review.
Cited by
-
AD Course Map charts Alzheimer's disease progression.Sci Rep. 2021 Apr 13;11(1):8020. doi: 10.1038/s41598-021-87434-1. Sci Rep. 2021. PMID: 33850174 Free PMC article.
-
Longitudinal changes in Alzheimer's-related plasma biomarkers and brain amyloid.medRxiv [Preprint]. 2023 Apr 21:2023.01.12.23284439. doi: 10.1101/2023.01.12.23284439. medRxiv. 2023. Update in: Alzheimers Dement. 2023 Oct;19(10):4335-4345. doi: 10.1002/alz.13157 PMID: 36711545 Free PMC article. Updated. Preprint.
-
The temporal event-based model: Learning event timelines in progressive diseases.Imaging Neurosci (Camb). 2023 Aug 21;1:1-19. doi: 10.1162/imag_a_00010. eCollection 2023 Aug 1. Imaging Neurosci (Camb). 2023. PMID: 37719837 Free PMC article.
-
Machine learning in neurological disorders: A multivariate LSTM and AdaBoost approach to Alzheimer's disease time series analysis.Health Care Sci. 2024 Feb 27;3(1):41-52. doi: 10.1002/hcs2.84. eCollection 2024 Feb. Health Care Sci. 2024. PMID: 38939169 Free PMC article.
-
Robust parametric modeling of Alzheimer's disease progression.Neuroimage. 2021 Jan 15;225:117460. doi: 10.1016/j.neuroimage.2020.117460. Epub 2020 Oct 16. Neuroimage. 2021. PMID: 33075562 Free PMC article.
References
-
- Villemagne V.L., Burnham S., Bourgeat P., Brown B., Ellis K.A., Salvado O. Amyloid β deposition, neurodegeneration, and cognitive decline in sporadic Alzheimer's disease: a prospective cohort study. Lancet Neurol. 2013;12:357–367. - PubMed
-
- Fonteijn H.M., Modat M., Clarkson M.J., Barnes J., Lehmann M., Hobbs N.Z. An event-based model for disease progression and its application in familial Alzheimer’s disease and Huntington’s disease. NeuroImage. 2012;60:1880–1889. - PubMed
Grants and funding
LinkOut - more resources
Full Text Sources
Other Literature Sources