Information-Theoretic Intrinsic Plasticity for Online Unsupervised Learning in Spiking Neural Networks
- PMID: 30804736
- PMCID: PMC6371195
- DOI: 10.3389/fnins.2019.00031
Information-Theoretic Intrinsic Plasticity for Online Unsupervised Learning in Spiking Neural Networks
Abstract
As a self-adaptive mechanism, intrinsic plasticity (IP) plays an essential role in maintaining homeostasis and shaping the dynamics of neural circuits. From a computational point of view, IP has the potential to enable promising non-Hebbian learning in artificial neural networks. While IP based learning has been attempted for spiking neuron models, the existing IP rules are ad hoc in nature, and the practical success of their application has not been demonstrated particularly toward enabling real-life learning tasks. This work aims to address the theoretical and practical limitations of the existing works by proposing a new IP rule named SpiKL-IP. SpiKL-IP is developed based on a rigorous information-theoretic approach where the target of IP tuning is to maximize the entropy of the output firing rate distribution of each spiking neuron. This goal is achieved by tuning the output firing rate distribution toward a targeted optimal exponential distribution. Operating on a proposed firing-rate transfer function, SpiKL-IP adapts the intrinsic parameters of a spiking neuron while minimizing the KL-divergence from the targeted exponential distribution to the actual output firing rate distribution. SpiKL-IP can robustly operate in an online manner under complex inputs and network settings. Simulation studies demonstrate that the application of SpiKL-IP to individual neurons in isolation or as part of a larger spiking neural network robustly produces the desired exponential distribution. The evaluation of SpiKL-IP under real-world speech and image classification tasks shows that SpiKL-IP noticeably outperforms two existing IP rules and can significantly boost recognition accuracy by up to more than 16%.
Keywords: image classification; intrinsic plasticity; liquid state machine; speech recognition; spiking neural networks; unsupervised learning.
Figures
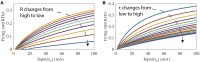

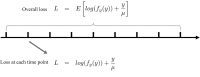
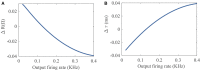
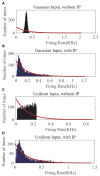
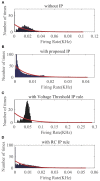
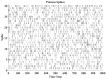
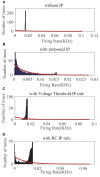
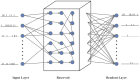
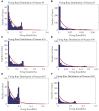
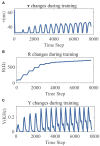
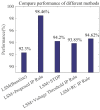
Similar articles
-
Event-Driven Intrinsic Plasticity for Spiking Convolutional Neural Networks.IEEE Trans Neural Netw Learn Syst. 2022 May;33(5):1986-1995. doi: 10.1109/TNNLS.2021.3084955. Epub 2022 May 2. IEEE Trans Neural Netw Learn Syst. 2022. PMID: 34106868
-
A Highly Effective and Robust Membrane Potential-Driven Supervised Learning Method for Spiking Neurons.IEEE Trans Neural Netw Learn Syst. 2019 Jan;30(1):123-137. doi: 10.1109/TNNLS.2018.2833077. Epub 2018 May 28. IEEE Trans Neural Netw Learn Syst. 2019. PMID: 29993588
-
Implementing Signature Neural Networks with Spiking Neurons.Front Comput Neurosci. 2016 Dec 20;10:132. doi: 10.3389/fncom.2016.00132. eCollection 2016. Front Comput Neurosci. 2016. PMID: 28066221 Free PMC article.
-
Models of Metaplasticity: A Review of Concepts.Front Comput Neurosci. 2015 Nov 10;9:138. doi: 10.3389/fncom.2015.00138. eCollection 2015. Front Comput Neurosci. 2015. PMID: 26617512 Free PMC article. Review.
-
Basic concepts of artificial neural network (ANN) modeling and its application in pharmaceutical research.J Pharm Biomed Anal. 2000 Jun;22(5):717-27. doi: 10.1016/s0731-7085(99)00272-1. J Pharm Biomed Anal. 2000. PMID: 10815714 Review.
Cited by
-
Intrinsic threshold plasticity: cholinergic activation and role in the neuronal recognition of incomplete input patterns.J Physiol. 2023 Aug;601(15):3221-3239. doi: 10.1113/JP283473. Epub 2022 Aug 11. J Physiol. 2023. PMID: 35879872 Free PMC article.
-
Adaptive structure evolution and biologically plausible synaptic plasticity for recurrent spiking neural networks.Sci Rep. 2023 Oct 7;13(1):16924. doi: 10.1038/s41598-023-43488-x. Sci Rep. 2023. PMID: 37805632 Free PMC article.
-
Extended liquid state machines for speech recognition.Front Neurosci. 2022 Oct 28;16:1023470. doi: 10.3389/fnins.2022.1023470. eCollection 2022. Front Neurosci. 2022. PMID: 36389242 Free PMC article.
-
Composing recurrent spiking neural networks using locally-recurrent motifs and risk-mitigating architectural optimization.Front Neurosci. 2024 Jun 20;18:1412559. doi: 10.3389/fnins.2024.1412559. eCollection 2024. Front Neurosci. 2024. PMID: 38966757 Free PMC article.
-
Snn and sound: a comprehensive review of spiking neural networks in sound.Biomed Eng Lett. 2024 Jul 11;14(5):981-991. doi: 10.1007/s13534-024-00406-y. eCollection 2024 Sep. Biomed Eng Lett. 2024. PMID: 39220030 Review.
References
-
- Cordts M., Omran M., Ramos S., Rehfeld T., Enzweiler M., Benenson R., et al. (2016). The cityscapes dataset for semantic urban scene understanding, in Proceedings of the IEEE Conference on Computer Vision and Pattern Recognition, 3213–3223.
-
- Dayan P., Abbott L. F. (2001). Theoretical Neuroscience, Vol. 806 Cambridge, MA: MIT Press.
LinkOut - more resources
Full Text Sources
Research Materials