Development of a Recognition System for Spraying Areas from Unmanned Aerial Vehicles Using a Machine Learning Approach
- PMID: 30646586
- PMCID: PMC6359728
- DOI: 10.3390/s19020313
Development of a Recognition System for Spraying Areas from Unmanned Aerial Vehicles Using a Machine Learning Approach
Abstract
Unmanned aerial vehicle (UAV)-based spraying systems have recently become important for the precision application of pesticides, using machine learning approaches. Therefore, the objective of this research was to develop a machine learning system that has the advantages of high computational speed and good accuracy for recognizing spray and non-spray areas for UAV-based sprayers. A machine learning system was developed by using the mutual subspace method (MSM) for images collected from a UAV. Two target lands: agricultural croplands and orchard areas, were considered in building two classifiers for distinguishing spray and non-spray areas. The field experiments were conducted in target areas to train and test the system by using a commercial UAV (DJI Phantom 3 Pro) with an onboard 4K camera. The images were collected from low (5 m) and high (15 m) altitudes for croplands and orchards, respectively. The recognition system was divided into offline and online systems. In the offline recognition system, 74.4% accuracy was obtained for the classifiers in recognizing spray and non-spray areas for croplands. In the case of orchards, the average classifier recognition accuracy of spray and non-spray areas was 77%. On the other hand, the online recognition system performance had an average accuracy of 65.1% for croplands, and 75.1% for orchards. The computational time for the online recognition system was minimal, with an average of 0.0031 s for classifier recognition. The developed machine learning system had an average recognition accuracy of 70%, which can be implemented in an autonomous UAV spray system for recognizing spray and non-spray areas for real-time applications.
Keywords: image classifiers; machine learning system; mutual subspace method; precision agriculture; recognition system.
Conflict of interest statement
The authors declare no conflict of interest.
Figures
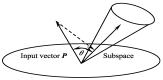
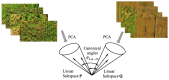
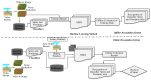
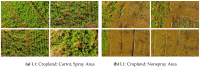
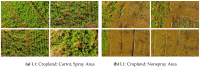
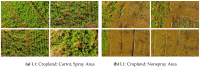
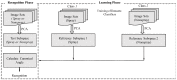
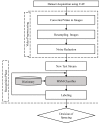
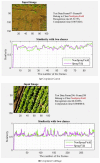
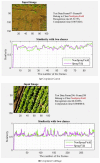
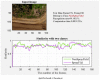
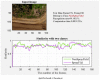
Similar articles
-
Real-time recognition of spraying area for UAV sprayers using a deep learning approach.PLoS One. 2021 Apr 1;16(4):e0249436. doi: 10.1371/journal.pone.0249436. eCollection 2021. PLoS One. 2021. PMID: 33793634 Free PMC article.
-
Spray performance evaluation of a six-rotor unmanned aerial vehicle sprayer for pesticide application using an orchard operation mode in apple orchards.Pest Manag Sci. 2022 Jun;78(6):2449-2466. doi: 10.1002/ps.6875. Epub 2022 Apr 6. Pest Manag Sci. 2022. PMID: 35306733
-
Risk assessment of environmental and bystander exposure from agricultural unmanned aerial vehicle sprayers in golden coconut plantations: Effects of droplet size and spray volume.Ecotoxicol Environ Saf. 2024 Sep 1;282:116675. doi: 10.1016/j.ecoenv.2024.116675. Epub 2024 Jul 5. Ecotoxicol Environ Saf. 2024. PMID: 38971099
-
Research on Methods Decreasing Pesticide Waste Based on Plant Protection Unmanned Aerial Vehicles: A Review.Front Plant Sci. 2022 Jul 7;13:811256. doi: 10.3389/fpls.2022.811256. eCollection 2022. Front Plant Sci. 2022. PMID: 35873963 Free PMC article. Review.
-
Challenges and opportunities of unmanned aerial vehicles as a new tool for crop pest control.Pest Manag Sci. 2023 Nov;79(11):4123-4131. doi: 10.1002/ps.7683. Epub 2023 Sep 12. Pest Manag Sci. 2023. PMID: 37494136 Review.
Cited by
-
Design of Plant Protection UAV Variable Spray System Based on Neural Networks.Sensors (Basel). 2019 Mar 5;19(5):1112. doi: 10.3390/s19051112. Sensors (Basel). 2019. PMID: 30841563 Free PMC article.
-
A Machine Learning Method for Vision-Based Unmanned Aerial Vehicle Systems to Understand Unknown Environments.Sensors (Basel). 2020 Jun 7;20(11):3245. doi: 10.3390/s20113245. Sensors (Basel). 2020. PMID: 32517309 Free PMC article.
-
Real-time recognition of spraying area for UAV sprayers using a deep learning approach.PLoS One. 2021 Apr 1;16(4):e0249436. doi: 10.1371/journal.pone.0249436. eCollection 2021. PLoS One. 2021. PMID: 33793634 Free PMC article.
-
A novel semi-supervised framework for UAV based crop/weed classification.PLoS One. 2021 May 10;16(5):e0251008. doi: 10.1371/journal.pone.0251008. eCollection 2021. PLoS One. 2021. PMID: 33970938 Free PMC article.
-
Spiking Neural Network (SNN) With Memristor Synapses Having Non-linear Weight Update.Front Comput Neurosci. 2021 Mar 11;15:646125. doi: 10.3389/fncom.2021.646125. eCollection 2021. Front Comput Neurosci. 2021. PMID: 33776676 Free PMC article.
References
-
- Zhang Y., Chen D., Wang S., Tian L. A promising trend for field information collection: An air-ground multi-sensor monitoring system. Inf. Process. Agric. 2018;5:224–233. doi: 10.1016/j.inpa.2018.02.002. - DOI
-
- Peña Barragán J.M., Kelly M., Castro A.I.D., López Granados F. Object-based approach for crop row characterization in UAV images for site-specific weed management; Proceedings of the 4th Geographic Object-Based Image Analysis (GEOBIA); Rio de Janeiro, Brazil. 7–12 May 2012; p. 426.
-
- Salvatore M., Matthew F.M., Pauline E.M., Richard L., Victor P.M., Giorgos M., Eyal B.D., David H., Lyndon E., Giuseppe C., et al. On the use of unmanned aerial systems for environmental monitoring. Remote Sens. 2018;10:641.
-
- Bandini F., Jakobsen J., Olesen D., Reyna-Gutierrez J.A., Bauer-Gottwein P. Measuring water level in rivers and lakes from lightweight unmanned aerial vehicles. J. Hydrol. 2017;548:237–250. doi: 10.1016/j.jhydrol.2017.02.038. - DOI
-
- Zarco-Tejada P.J., González-Dugo V., Berni J.A. Fluorescence, temperature and narrow-band indices acquired from a UAV platform for water stress detection using a micro-hyperspectral imager and a thermal camera. Remote Sens. Environ. 2012;117:322–337. doi: 10.1016/j.rse.2011.10.007. - DOI
LinkOut - more resources
Full Text Sources